mirror of
https://github.com/w-okada/voice-changer.git
synced 2025-01-23 21:45:00 +03:00
commit
29d3aa0ba4
51
README.md
51
README.md
@ -1,5 +1,19 @@
|
||||
Voice Changer Trainer and Player
|
||||
----
|
||||
# News
|
||||
2022/12/09: Anacondaに対応しました(β版)。以下の構成に対応します。
|
||||
|
||||
| # | os | middle |
|
||||
| --- | ------------- | -------- |
|
||||
| 1 | Windows | Anaconda |
|
||||
| 2 | Windows(WSL2) | Docker |
|
||||
| 3 | Windows(WSL2) | Anaconda |
|
||||
| 4 | Mac(Intel) | Anaconda |
|
||||
| 5 | Mac(M1) | Anaconda |
|
||||
| 6 | Linux | Docker |
|
||||
| 7 | Linux | Anaconda |
|
||||
| 8 | Colab | Notebook |
|
||||
|
||||
# 概要
|
||||
AIを使ったリアルタイムボイスチェンジャー[MMVC](https://github.com/isletennos/MMVC_Trainer)のヘルパーアプリケーションです。
|
||||
|
||||
@ -8,17 +22,17 @@ MMVCで必要となる一連の作業(トレーニング用の音声の録音
|
||||
|
||||
このアプリケーションを用いることで、以下のことを簡単に行うことができます。
|
||||
|
||||
- MMVCトレーニング用の音声録音 (GithubPages (Docker不要))
|
||||
- MMVCのモデルのトレーニング (Dockerを強く推奨、Colabでも可)
|
||||
- MMVCモデルを用いたリアルタイムボイスチェンジャー(Docker推奨、Colabでも可)
|
||||
- MMVCトレーニング用の音声録音 (GithubPages (ローカル環境構築不要))
|
||||
- MMVCのモデルのトレーニング (Dockerを強く推奨、その他構成Colabでも可)
|
||||
- MMVCモデルを用いたリアルタイムボイスチェンジャー
|
||||
- リアルタイム話者切り替え
|
||||
- CPU/GPU切り替え
|
||||
- リアルタイム/ニアリアルタイム声質変換
|
||||
|
||||
|
||||
本アプリケーションのリアルタイムボイスチェンジャーは、サーバ・クライアント構成で動きます。MMVCのサーバを別のPC上で動かすことで、ゲーム実況など他の負荷の高い処理への影響を抑えながら動かすことができます。(MacのChromeからも利用できます!!)
|
||||
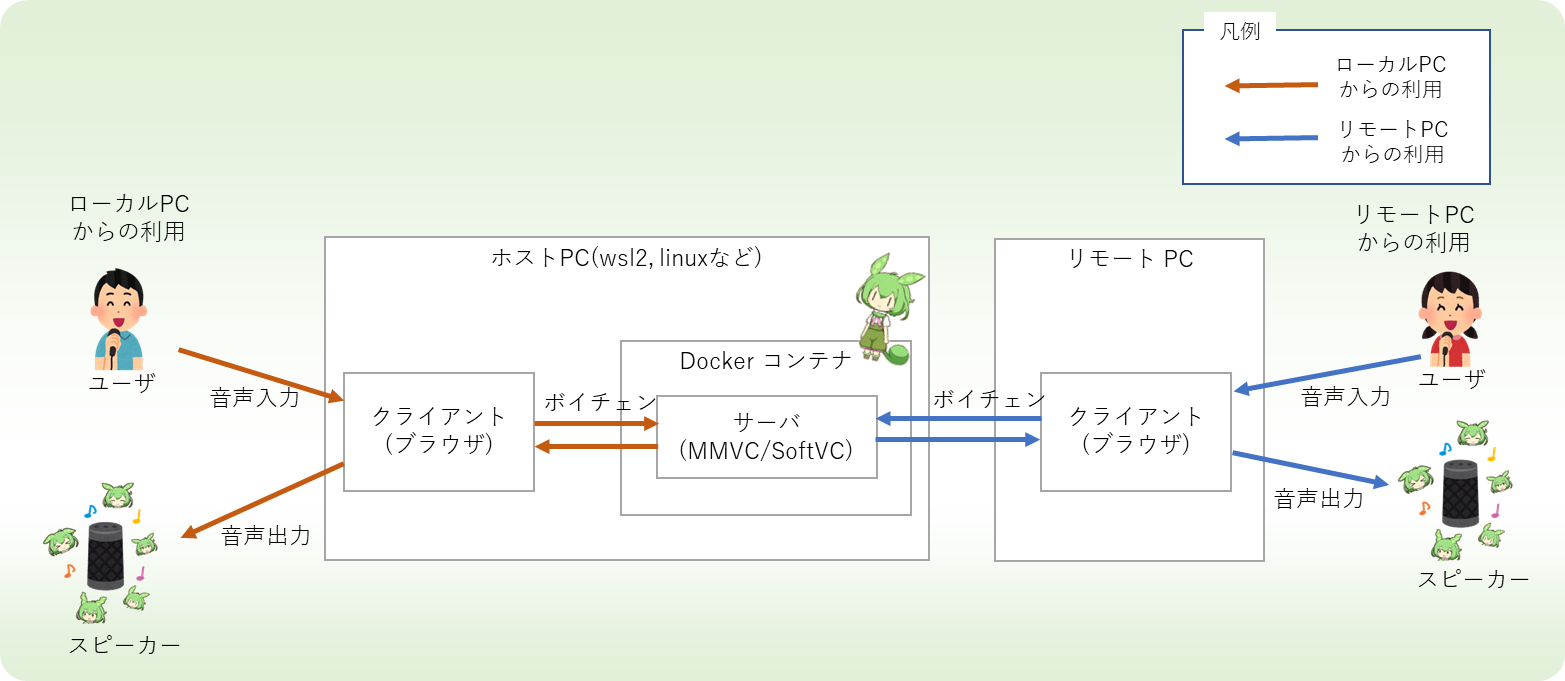
|
||||
本アプリケーションのリアルタイムボイスチェンジャーは、サーバ・クライアント構成で動きます。MMVCのサーバを別のPC上で動かすことで、ゲーム実況など他の負荷の高い処理への影響を抑えながら動かすことができます。
|
||||
|
||||
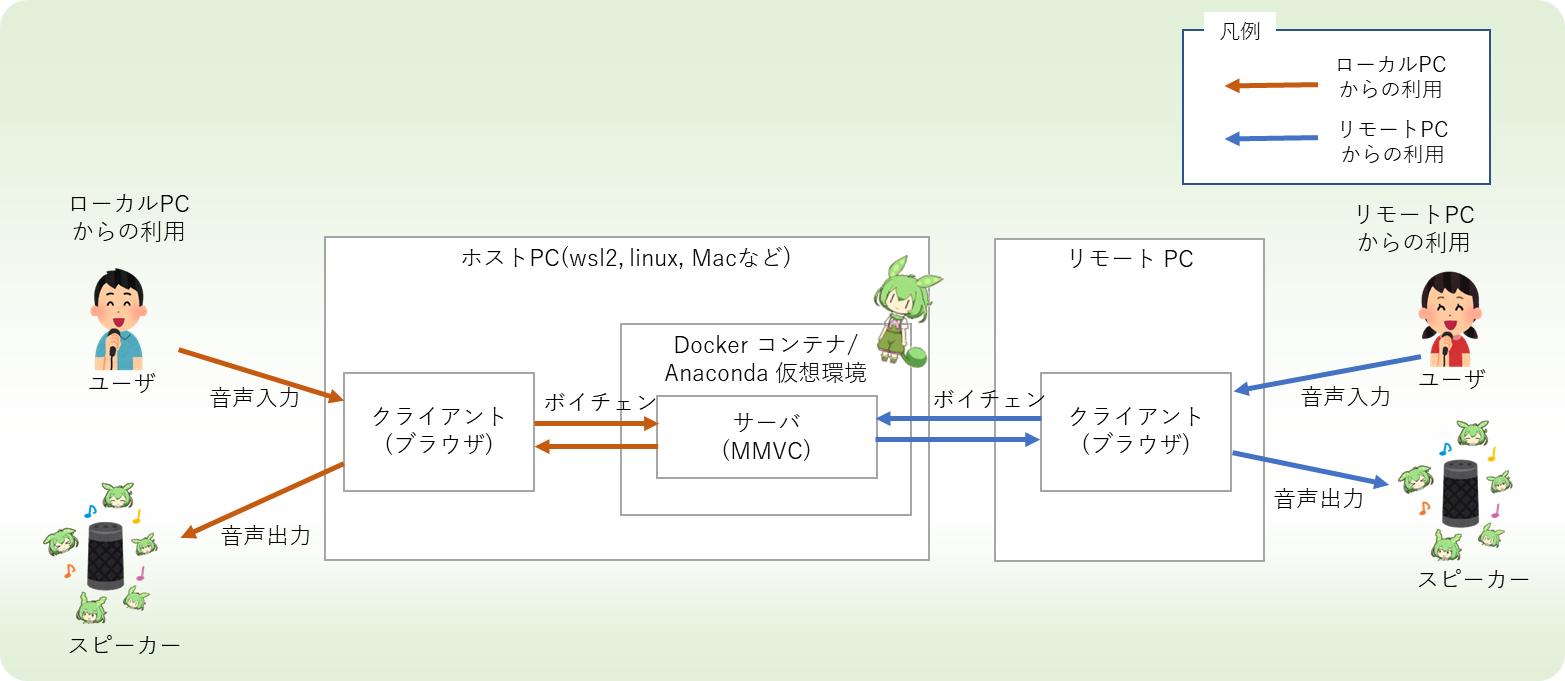
|
||||
|
||||
# 使用方法
|
||||
|
||||
@ -45,28 +59,19 @@ Colaboratoryで実行する場合は、Colabの制約により途中切断が発
|
||||
|
||||
## プレイヤー(ボイスチェンジャーアプリ)
|
||||
MMVCでボイチェンを行うためのアプリです。
|
||||
Dockerで起動する場合は
|
||||
Dockerでの使用を推奨します。一部ユーザ体験が劣化しますが、次のノートでColaboratoryでの実行も可能です。
|
||||
お手元のPCでの使用を推奨します。一部ユーザ体験が劣化しますが、次のノートでColaboratoryでの実行も可能です。
|
||||
- [超簡単バージョン](https://github.com/w-okada/voice-changer/blob/master/VoiceChangerDemo_Simple.ipynb): 事前設定なしでColabから実行できます。
|
||||
- [普通バージョン](https://github.com/w-okada/voice-changer/blob/master/VoiceChangerDemo.ipynb): Google Driveと連携してモデルを読み込むことができます。
|
||||
|
||||
[説明動画](https://twitter.com/DannadoriYellow/status/1564897136999022592)
|
||||
|
||||
動画との差分
|
||||
|
||||
- サーバの起動完了のメッセージは、「Debuggerほにゃらら」ではなく「Application startup complete.」です。
|
||||
- プロキシにアクセスする際に、index.htmlを追加する必要はありません。
|
||||
|
||||
詳細な使用方法等は[wiki](https://github.com/w-okada/voice-changer/wiki/040_%E3%83%9C%E3%82%A4%E3%82%B9%E3%83%81%E3%82%A7%E3%83%B3%E3%82%B8%E3%83%A3%E3%83%BC)をご参照ください。
|
||||
|
||||
# 説明動画
|
||||
|No|タイトル|リンク|
|
||||
|---|---|---|
|
||||
|01|ざっくり説明編| [youtube](https://www.youtube.com/watch?v=MOPqnDPqhAU)|
|
||||
|02|ユーザー音声の録音編|[youtube](https://www.youtube.com/watch?v=s_GirFEGvaA)|
|
||||
|03|トレーニング編| 作成中|
|
||||
|04a|Colabでボイチェン編| 作成中|
|
||||
|04b|PCでボイチェン編| 作成中|
|
||||
| No | タイトル | リンク |
|
||||
| --- | ---------------------------------- | ------------------------------------------------------ |
|
||||
| 01 | ざっくり説明編 | [youtube](https://www.youtube.com/watch?v=MOPqnDPqhAU) |
|
||||
| 02 | ユーザー音声の録音編 | [youtube](https://www.youtube.com/watch?v=s_GirFEGvaA) |
|
||||
| 03 | トレーニング編 | 作成中 |
|
||||
| 04a | Colabでボイチェン編 | [youtube](https://youtu.be/TogfMzXH1T0) |
|
||||
| 04b | PCでボイチェン編 | 作成中 |
|
||||
| ex1 | 番外編:WSL2とDockerのインストール | [youtube](https://youtu.be/POo_Cg0eFMU) |
|
||||
|
||||
## リアルタイム性
|
||||
|
||||
|
16
conda/requirements.txt
Normal file
16
conda/requirements.txt
Normal file
@ -0,0 +1,16 @@
|
||||
Cython==0.29.32
|
||||
fastapi==0.88.0
|
||||
librosa==0.9.2
|
||||
numpy==1.23.5
|
||||
phonemizer==3.2.1
|
||||
pyOpenSSL==22.1.0
|
||||
python-multipart==0.0.5
|
||||
python-socketio==5.7.2
|
||||
retry==0.9.2
|
||||
scipy==1.9.3
|
||||
torch==1.13.0
|
||||
torchaudio==0.13.0
|
||||
tqdm==4.64.1
|
||||
Unidecode==1.3.6
|
||||
uvicorn==0.20.0
|
||||
websockets==10.4
|
@ -6,8 +6,8 @@ from distutils.util import strtobool
|
||||
import numpy as np
|
||||
from scipy.io.wavfile import write, read
|
||||
|
||||
sys.path.append("/MMVC_Trainer")
|
||||
sys.path.append("/MMVC_Trainer/text")
|
||||
sys.path.append("MMVC_Trainer")
|
||||
sys.path.append("MMVC_Trainer/text")
|
||||
|
||||
from fastapi.routing import APIRoute
|
||||
from fastapi import HTTPException, Request, Response, FastAPI, UploadFile, File, Form
|
||||
@ -244,7 +244,7 @@ if __name__ == thisFilename or args.colab == True:
|
||||
# ##########
|
||||
UPLOAD_DIR = "upload_dir"
|
||||
os.makedirs(UPLOAD_DIR, exist_ok=True)
|
||||
MODEL_DIR = "/MMVC_Trainer/logs"
|
||||
MODEL_DIR = "MMVC_Trainer/logs"
|
||||
os.makedirs(MODEL_DIR, exist_ok=True)
|
||||
|
||||
@app_fastapi.post("/upload_file")
|
||||
@ -290,7 +290,7 @@ if __name__ == thisFilename or args.colab == True:
|
||||
):
|
||||
zipFilePath = concat_file_chunks(
|
||||
UPLOAD_DIR, zipFilename, zipFileChunkNum, UPLOAD_DIR)
|
||||
shutil.unpack_archive(zipFilePath, "/MMVC_Trainer/dataset/textful/")
|
||||
shutil.unpack_archive(zipFilePath, "MMVC_Trainer/dataset/textful/")
|
||||
return {"Zip file unpacked": f"{zipFilePath}"}
|
||||
|
||||
############
|
||||
@ -476,12 +476,12 @@ if __name__ == '__main__':
|
||||
path = ""
|
||||
else:
|
||||
path = "trainer"
|
||||
if EX_PORT and EX_IP and args.https == 1:
|
||||
if "EX_PORT" in locals() and "EX_IP" in locals() and args.https == 1:
|
||||
printMessage(f"In many cases it is one of the following", level=1)
|
||||
printMessage(f"https://localhost:{EX_PORT}/{path}", level=1)
|
||||
for ip in EX_IP.strip().split(" "):
|
||||
printMessage(f"https://{ip}:{EX_PORT}/{path}", level=1)
|
||||
elif EX_PORT and EX_IP and args.https == 0:
|
||||
elif "EX_PORT" in locals() and "EX_IP" in locals() and args.https == 0:
|
||||
printMessage(f"In many cases it is one of the following", level=1)
|
||||
printMessage(f"http://localhost:{EX_PORT}/{path}", level=1)
|
||||
|
||||
|
43
demo/MMVC_Trainer/LICENSE
Executable file
43
demo/MMVC_Trainer/LICENSE
Executable file
@ -0,0 +1,43 @@
|
||||
MIT License
|
||||
|
||||
Copyright (c) 2022 Isle Tennos
|
||||
|
||||
Permission is hereby granted, free of charge, to any person obtaining a copy
|
||||
of this software and associated documentation files (the "Software"), to deal
|
||||
in the Software without restriction, including without limitation the rights
|
||||
to use, copy, modify, merge, publish, distribute, sublicense, and/or sell
|
||||
copies of the Software, and to permit persons to whom the Software is
|
||||
furnished to do so, subject to the following conditions:
|
||||
|
||||
The above copyright notice and this permission notice shall be included in all
|
||||
copies or substantial portions of the Software.
|
||||
|
||||
THE SOFTWARE IS PROVIDED "AS IS", WITHOUT WARRANTY OF ANY KIND, EXPRESS OR
|
||||
IMPLIED, INCLUDING BUT NOT LIMITED TO THE WARRANTIES OF MERCHANTABILITY,
|
||||
FITNESS FOR A PARTICULAR PURPOSE AND NONINFRINGEMENT. IN NO EVENT SHALL THE
|
||||
AUTHORS OR COPYRIGHT HOLDERS BE LIABLE FOR ANY CLAIM, DAMAGES OR OTHER
|
||||
LIABILITY, WHETHER IN AN ACTION OF CONTRACT, TORT OR OTHERWISE, ARISING FROM,
|
||||
OUT OF OR IN CONNECTION WITH THE SOFTWARE OR THE USE OR OTHER DEALINGS IN THE
|
||||
SOFTWARE.
|
||||
|
||||
MIT License
|
||||
|
||||
Copyright (c) 2021 Jaehyeon Kim
|
||||
|
||||
Permission is hereby granted, free of charge, to any person obtaining a copy
|
||||
of this software and associated documentation files (the "Software"), to deal
|
||||
in the Software without restriction, including without limitation the rights
|
||||
to use, copy, modify, merge, publish, distribute, sublicense, and/or sell
|
||||
copies of the Software, and to permit persons to whom the Software is
|
||||
furnished to do so, subject to the following conditions:
|
||||
|
||||
The above copyright notice and this permission notice shall be included in all
|
||||
copies or substantial portions of the Software.
|
||||
|
||||
THE SOFTWARE IS PROVIDED "AS IS", WITHOUT WARRANTY OF ANY KIND, EXPRESS OR
|
||||
IMPLIED, INCLUDING BUT NOT LIMITED TO THE WARRANTIES OF MERCHANTABILITY,
|
||||
FITNESS FOR A PARTICULAR PURPOSE AND NONINFRINGEMENT. IN NO EVENT SHALL THE
|
||||
AUTHORS OR COPYRIGHT HOLDERS BE LIABLE FOR ANY CLAIM, DAMAGES OR OTHER
|
||||
LIABILITY, WHETHER IN AN ACTION OF CONTRACT, TORT OR OTHERWISE, ARISING FROM,
|
||||
OUT OF OR IN CONNECTION WITH THE SOFTWARE OR THE USE OR OTHER DEALINGS IN THE
|
||||
SOFTWARE.
|
220
demo/MMVC_Trainer/README.md
Executable file
220
demo/MMVC_Trainer/README.md
Executable file
@ -0,0 +1,220 @@
|
||||
MMVC_Trainer
|
||||
====
|
||||
|
||||
AIを使ったリアルタイムボイスチェンジャーのモデル学習用ツール
|
||||
|
||||
## Description
|
||||
AIを使ったリアルタイムボイスチェンジャー「MMVC(RealTime-Many to Many Voice Conversion)」
|
||||
で使用するモデルを学習するためのリポジトリです。
|
||||
google colaboratoryを用いることで、個人の環境に依存せず、かつ簡単に機械学習の学習フェーズを実行可能です。
|
||||
## MMVC_Client
|
||||
MMVCを実際に動かすClient software
|
||||
https://github.com/isletennos/MMVC_Client
|
||||
## concept
|
||||
「簡単」「だれでも」「好きな声に」「リアルタイムで」
|
||||
## Demo
|
||||
制作中 (v1.3.0.0)
|
||||
https://www.nicovideo.jp/watch/sm40386035 (v1.2.0.0)
|
||||
|
||||
## MMVCの利用規約 及び MMVC用音源の配布先(2022/08/10)
|
||||
本ソフトウェアの利用規約は基本的にMITライセンスに準拠します。
|
||||
1. このソフトウェアは、コピー利用、配布、変更の追加、変更を加えたもの再配布、商用利用、有料販売など
|
||||
どなたでも自由にお使いいただくことができます。
|
||||
2. ライセンスの記載が可能なプラットフォームでの利用の場合、下記クレジットどちらかををご利用ください。
|
||||
**VRCでの利用などライセンス記載が不可の場合、記載は不要です。**
|
||||
(可能であればパターン2を使ってくれると製作者はうれしいです)
|
||||
3. このソフトウェアについて、製作者はいかなる保証も致しません。
|
||||
また、このソフトウェアを利用したことで問題が起きた際に、ソフトウェアの製作者は一切の責任を負いません。
|
||||
4. このソフトウェアで利用する音声データは、必ず元の音声データの所持者の許諾を得たものを利用すること。
|
||||
または音声データの配布元の利用規約内で利用すること。
|
||||
|
||||
### MMVC公式配布の音声データの利用規約とダウンロード先について
|
||||
MMVCの利用規約とは別に、下記音声データを利用する場合、それぞれの音声ライブラリ提供者様の利用規約に同意する必要があります。
|
||||
※本ソフトウェアでは下記企業様・団体様に特別に許可を頂き、音声データを本ソフトウェア用に改変、再配布を行っております。
|
||||
#### SSS LLC.
|
||||
[[利用規約](https://zunko.jp/guideline.html)][[ずんだもん 音声データ](https://drive.google.com/file/d/1h8Ajyvoig7Hl3LSSt2vYX0sUHX3JDF3R/view?usp=sharing)] ※本ソフトウェアに同梱しているものと同様の音声データになります
|
||||
[[利用規約](https://zunko.jp/guideline.html)][[九州そら 音声データ](https://drive.google.com/file/d/1MXfMRG_sjbsaLihm7wEASG2PwuCponZF/view?usp=sharing)]
|
||||
[[利用規約](https://zunko.jp/guideline.html)][[四国めたん 音声データ](https://drive.google.com/file/d/1iCrpzhqXm-0YdktOPM8M1pMtgQIDF3r4/view?usp=sharing)]
|
||||
#### 春日部つむぎプロジェクト様
|
||||
[[利用規約](https://tsumugi-official.studio.site/rule)][[春日部つむぎ 音声データ](https://drive.google.com/file/d/14zE0F_5ZCQWXf6m6SUPF5Y3gpL6yb7zk/view?usp=sharing)]
|
||||
|
||||
### ライセンス表記について
|
||||
ずんだもん/四国めたん/九州そら/春日部つむぎ
|
||||
の3キャラクターを利用する場合に限り、下記ライセンスパターンに加えて、どのツールで作られた音声かわかるように
|
||||
```
|
||||
MMVC:ずんだもん
|
||||
MMVC:ずんだもん/四国めたん
|
||||
```
|
||||
等の記載を下記ライセンスパターンと一緒に記載ください。
|
||||
こちらも**VRCでの利用などライセンス記載が不可の場合、記載は不要です。**
|
||||
|
||||
ライセンスパターン 1
|
||||
```
|
||||
Copyright (c) 2022 Isle.Tennos
|
||||
Released under the MIT license
|
||||
https://opensource.org/licenses/mit-license.php
|
||||
```
|
||||
|
||||
ライセンスパターン 2
|
||||
```
|
||||
MMVCv1.x.x.x(使用バージョン)
|
||||
Copyright (c) 2022 Isle.Tennos
|
||||
Released under the MIT license
|
||||
https://opensource.org/licenses/mit-license.php
|
||||
git:https://github.com/isletennos/MMVC_Trainer
|
||||
community(discord):https://discord.gg/PgspuDSTEc
|
||||
```
|
||||
## Requirement
|
||||
・Google アカウント
|
||||
## Install
|
||||
このリポジトリをダウンロードして、展開、展開したディレクトリをgoogle drive上にアップロードしてください。
|
||||
## Usage
|
||||
### チュートリアル : ずんだもんになる
|
||||
本チュートリアルではずんだもん(SSS LLC.)の音声データを利用します。
|
||||
そのため、MMVCの利用規約とは別に[[ずんだもん 利用規約](https://zunko.jp/guideline.html)]を遵守する必要があります。
|
||||
#### Ph1. 自分の音声の録音と音声データの配置
|
||||
1. 自分の声の音声データを録音します。
|
||||
JVSコーパスやITAコーパス等を台本にし、100文程度読み上げます。
|
||||
また、録音した音声は**24000Hz 16bit 1ch**である必要があります。
|
||||
※MMVC用にテキストを分割したITAコーパスです。ご利用ください。
|
||||
https://drive.google.com/file/d/14oXoQqLxRkP8NJK8qMYGee1_q2uEED1z/view?usp=sharing
|
||||
|
||||
2. dataset/textful/000_myvoice に音声データとテキストデータを配置します。
|
||||
最終的に下記のようなディレクトリ構成になります。
|
||||
```
|
||||
dataset
|
||||
├── textful
|
||||
│ ├── 000_myvoice
|
||||
│ │ ├── text
|
||||
│ │ │ ├── s_voice_001.txt
|
||||
│ │ │ ├── s_voice_002.txt
|
||||
│ │ │ ├── ...
|
||||
│ │ └── wav
|
||||
│ │ ├── s_voice_001.wav
|
||||
│ │ ├── s_voice_002.wav
|
||||
│ │ ├── ...
|
||||
│ │── 001_target
|
||||
│ │ ├── text
|
||||
│ │ └── wav
|
||||
│ │
|
||||
│ └── 1205_zundamon
|
||||
│ ├── text
|
||||
│ │ ├── t_voice_001.txt
|
||||
│ │ ├── t_voice_002.txt
|
||||
│ │ ├── ...
|
||||
│ └── wav
|
||||
│ ├── t_voice_001.wav
|
||||
│ ├── t_voice_002.wav
|
||||
│ ├── ...
|
||||
│
|
||||
└── textless
|
||||
```
|
||||
|
||||
#### Ph2. モデルの学習方法
|
||||
1. 下記リンクより、「G_180000.pth」「D_180000.pth」をダウンロード。
|
||||
https://drive.google.com/drive/folders/1vXdL1zSrgsuyACMkiTUtVbHgpMSA1Y5I?usp=sharing
|
||||
2. 「G_180000.pth」「D_180000.pth」をfine_modelに配置します。**(良く忘れるポイントなので要注意!)**
|
||||
3. notebookディレクトリにある「Create_Configfile_zundamon.ipynb」をgoogle colab 上で実行、学習に必要なconfigファイルを作成します
|
||||
4. configsに作成されたtrain_config_zundamon.jsonの
|
||||
|
||||
- "eval_interval"
|
||||
modelを保存する間隔です。
|
||||
- "batch_size"
|
||||
colabで割り当てたGPUに合わせて調整してください。
|
||||
|
||||
上記2項目を環境に応じて最適化してください。わからない方はそのままで大丈夫です。
|
||||
|
||||
5. notebookディレクトリにある「Train_MMVC.ipynb」をgoogle colab 上で実行してください。
|
||||
logs/にモデルが生成されます。
|
||||
|
||||
#### Ph3. 学習したモデルの性能検証
|
||||
1. notebookディレクトリにある「MMVC_Interface.ipynb」をgoogle colab 上で実行してください。
|
||||
### 好きなキャラクターの声になる
|
||||
#### Ph1. 自分の音声の録音と音声データの配置 及びターゲット音声データの配置
|
||||
1. 自分の声の音声データとその音声データに対応するテキスト、変換したい声の音声データとその音声データに対応するテキストを用意します。
|
||||
この時、用意する音声(自分の声の音声データ/変換したい声の音声データ共に)は**24000Hz 16bit 1ch**を強く推奨しております。
|
||||
2. 下記のようなディレクトリ構成になるように音声データとテキストデータを配置します。
|
||||
textfulの直下には2ディレクトリになります。
|
||||
(1205_zundamonディレクトリは無くても問題ありません)
|
||||
|
||||
```
|
||||
dataset
|
||||
├── textful
|
||||
│ ├── 000_myvoice
|
||||
│ │ ├── text
|
||||
│ │ │ ├── s_voice_001.txt
|
||||
│ │ │ ├── s_voice_002.txt
|
||||
│ │ │ ├── ...
|
||||
│ │ └── wav
|
||||
│ │ ├── s_voice_001.wav
|
||||
│ │ ├── s_voice_002.wav
|
||||
│ │ ├── ...
|
||||
│ │── 001_target
|
||||
│ │ ├── text
|
||||
│ │ │ ├── t_voice_001.txt
|
||||
│ │ │ ├── t_voice_002.txt
|
||||
│ │ │ ├── ...
|
||||
│ │ └── wav
|
||||
│ │ ├── t_voice_001.wav
|
||||
│ │ ├── t_voice_002.wav
|
||||
│ │ ├── ...
|
||||
│ └── 1205_zundamon
|
||||
│ ├── text
|
||||
│ │ ├── t_voice_001.txt
|
||||
│ │ ├── t_voice_002.txt
|
||||
│ │ ├── ...
|
||||
│ └── wav
|
||||
│ ├── t_voice_001.wav
|
||||
│ ├── t_voice_002.wav
|
||||
│ ├── ...
|
||||
│
|
||||
└── textless
|
||||
```
|
||||
#### Ph2. モデルの学習方法
|
||||
以降、「チュートリアル : ずんだもんになる Ph2.」と同様のため割愛
|
||||
#### Ph3. 学習したモデルの性能検証
|
||||
以降、「チュートリアル : ずんだもんになる Ph3.」と同様のため割愛
|
||||
## 有志によるチュートリアル動画
|
||||
### v1.2.1.x
|
||||
| 前準備編 | [ニコニコ動画](https://www.nicovideo.jp/watch/sm40415108) | [YouTube](https://www.youtube.com/watch?v=gq1Hpn5CARw&ab_channel=popi) |
|
||||
|:--------------|:------------|:------------|
|
||||
| 要修正音声 | [ニコニコ動画](https://www.nicovideo.jp/watch/sm40420683)| [YouTube](https://youtu.be/NgzC7Nuk6gg) |
|
||||
| 前準備編2 | [ニコニコ動画](https://www.nicovideo.jp/watch/sm40445164)| [YouTube](https://youtu.be/m4Jew7sTs9w)
|
||||
| 学習編_前1 | [ニコニコ動画](https://www.nicovideo.jp/watch/sm40467662)| [YouTube](https://youtu.be/HRSPEy2jUvg)
|
||||
| 学習編_前2 | [ニコニコ動画](https://www.nicovideo.jp/watch/sm40473168)| [YouTube](https://youtu.be/zQW59vrOSuA)
|
||||
| 学習編_後 | [ニコニコ動画](https://www.nicovideo.jp/watch/sm40490554)| [YouTube](https://www.youtube.com/watch?v=uB3YfdKzo-g&ab_channel=popi)
|
||||
| リアルタイム編 | [ニコニコ動画](https://www.nicovideo.jp/watch/sm40415108)| [YouTube](https://youtu.be/Al5DFCvKLFA)
|
||||
| 質問編 | [ニコニコ動画](https://www.nicovideo.jp/watch/sm40599514)| [YouTube](https://youtu.be/aGBcqu5M6-c)
|
||||
| 応用編_九州そら| [ニコニコ動画](https://www.nicovideo.jp/watch/sm40647601)| [YouTube](https://youtu.be/MEXKZoHVd-A)
|
||||
| 応用編_音街ウナ| [ニコニコ動画](https://www.nicovideo.jp/watch/sm40714406)| [YouTube](https://youtu.be/JDMlRz-PkSE)
|
||||
|
||||
## Q&A
|
||||
下記サイトをご参考ください。
|
||||
https://mmvc.readthedocs.io/ja/latest/index.html
|
||||
## MMVCコミュニティサーバ(discord)
|
||||
開発の最新情報や、不明点のお問合せ、MMVCの活用法などMMVCに関するコミュニティサーバです。
|
||||
https://discord.gg/PgspuDSTEc
|
||||
|
||||
## Special thanks
|
||||
- JVS (Japanese versatile speech) corpus
|
||||
contributors : 高道 慎之介様/三井 健太郎様/齋藤 佑樹様/郡山 知樹様/丹治 尚子様/猿渡 洋様
|
||||
https://sites.google.com/site/shinnosuketakamichi/research-topics/jvs_corpus
|
||||
|
||||
- ITAコーパス マルチモーダルデータベース
|
||||
contributors : 金井郁也様/千葉隆壱様/齊藤剛史様/森勢将雅様/小口純矢様/能勢隆様/尾上真惟子様/小田恭央様
|
||||
CharacterVoice : 東北イタコ(木戸衣吹様)/ずんだもん(伊藤ゆいな様)/四国めたん(田中小雪様)/九州そら(西田望見)
|
||||
https://zunko.jp/multimodal_dev/login.php
|
||||
|
||||
- つくよみちゃんコーパス
|
||||
contributor : 夢前黎様
|
||||
CharacterVoice : つくよみちゃん(夢前黎様)
|
||||
https://tyc.rei-yumesaki.net/material/corpus/
|
||||
|
||||
## Reference
|
||||
https://arxiv.org/abs/2106.06103
|
||||
https://github.com/jaywalnut310/vits
|
||||
|
||||
## Author
|
||||
Isle Tennos
|
||||
Twitter : https://twitter.com/IsleTennos
|
||||
|
303
demo/MMVC_Trainer/attentions.py
Executable file
303
demo/MMVC_Trainer/attentions.py
Executable file
@ -0,0 +1,303 @@
|
||||
import copy
|
||||
import math
|
||||
import numpy as np
|
||||
import torch
|
||||
from torch import nn
|
||||
from torch.nn import functional as F
|
||||
|
||||
import commons
|
||||
import modules
|
||||
from modules import LayerNorm
|
||||
|
||||
|
||||
class Encoder(nn.Module):
|
||||
def __init__(self, hidden_channels, filter_channels, n_heads, n_layers, kernel_size=1, p_dropout=0., window_size=4, **kwargs):
|
||||
super().__init__()
|
||||
self.hidden_channels = hidden_channels
|
||||
self.filter_channels = filter_channels
|
||||
self.n_heads = n_heads
|
||||
self.n_layers = n_layers
|
||||
self.kernel_size = kernel_size
|
||||
self.p_dropout = p_dropout
|
||||
self.window_size = window_size
|
||||
|
||||
self.drop = nn.Dropout(p_dropout)
|
||||
self.attn_layers = nn.ModuleList()
|
||||
self.norm_layers_1 = nn.ModuleList()
|
||||
self.ffn_layers = nn.ModuleList()
|
||||
self.norm_layers_2 = nn.ModuleList()
|
||||
for i in range(self.n_layers):
|
||||
self.attn_layers.append(MultiHeadAttention(hidden_channels, hidden_channels, n_heads, p_dropout=p_dropout, window_size=window_size))
|
||||
self.norm_layers_1.append(LayerNorm(hidden_channels))
|
||||
self.ffn_layers.append(FFN(hidden_channels, hidden_channels, filter_channels, kernel_size, p_dropout=p_dropout))
|
||||
self.norm_layers_2.append(LayerNorm(hidden_channels))
|
||||
|
||||
def forward(self, x, x_mask):
|
||||
attn_mask = x_mask.unsqueeze(2) * x_mask.unsqueeze(-1)
|
||||
x = x * x_mask
|
||||
for i in range(self.n_layers):
|
||||
y = self.attn_layers[i](x, x, attn_mask)
|
||||
y = self.drop(y)
|
||||
x = self.norm_layers_1[i](x + y)
|
||||
|
||||
y = self.ffn_layers[i](x, x_mask)
|
||||
y = self.drop(y)
|
||||
x = self.norm_layers_2[i](x + y)
|
||||
x = x * x_mask
|
||||
return x
|
||||
|
||||
|
||||
class Decoder(nn.Module):
|
||||
def __init__(self, hidden_channels, filter_channels, n_heads, n_layers, kernel_size=1, p_dropout=0., proximal_bias=False, proximal_init=True, **kwargs):
|
||||
super().__init__()
|
||||
self.hidden_channels = hidden_channels
|
||||
self.filter_channels = filter_channels
|
||||
self.n_heads = n_heads
|
||||
self.n_layers = n_layers
|
||||
self.kernel_size = kernel_size
|
||||
self.p_dropout = p_dropout
|
||||
self.proximal_bias = proximal_bias
|
||||
self.proximal_init = proximal_init
|
||||
|
||||
self.drop = nn.Dropout(p_dropout)
|
||||
self.self_attn_layers = nn.ModuleList()
|
||||
self.norm_layers_0 = nn.ModuleList()
|
||||
self.encdec_attn_layers = nn.ModuleList()
|
||||
self.norm_layers_1 = nn.ModuleList()
|
||||
self.ffn_layers = nn.ModuleList()
|
||||
self.norm_layers_2 = nn.ModuleList()
|
||||
for i in range(self.n_layers):
|
||||
self.self_attn_layers.append(MultiHeadAttention(hidden_channels, hidden_channels, n_heads, p_dropout=p_dropout, proximal_bias=proximal_bias, proximal_init=proximal_init))
|
||||
self.norm_layers_0.append(LayerNorm(hidden_channels))
|
||||
self.encdec_attn_layers.append(MultiHeadAttention(hidden_channels, hidden_channels, n_heads, p_dropout=p_dropout))
|
||||
self.norm_layers_1.append(LayerNorm(hidden_channels))
|
||||
self.ffn_layers.append(FFN(hidden_channels, hidden_channels, filter_channels, kernel_size, p_dropout=p_dropout, causal=True))
|
||||
self.norm_layers_2.append(LayerNorm(hidden_channels))
|
||||
|
||||
def forward(self, x, x_mask, h, h_mask):
|
||||
"""
|
||||
x: decoder input
|
||||
h: encoder output
|
||||
"""
|
||||
self_attn_mask = commons.subsequent_mask(x_mask.size(2)).to(device=x.device, dtype=x.dtype)
|
||||
encdec_attn_mask = h_mask.unsqueeze(2) * x_mask.unsqueeze(-1)
|
||||
x = x * x_mask
|
||||
for i in range(self.n_layers):
|
||||
y = self.self_attn_layers[i](x, x, self_attn_mask)
|
||||
y = self.drop(y)
|
||||
x = self.norm_layers_0[i](x + y)
|
||||
|
||||
y = self.encdec_attn_layers[i](x, h, encdec_attn_mask)
|
||||
y = self.drop(y)
|
||||
x = self.norm_layers_1[i](x + y)
|
||||
|
||||
y = self.ffn_layers[i](x, x_mask)
|
||||
y = self.drop(y)
|
||||
x = self.norm_layers_2[i](x + y)
|
||||
x = x * x_mask
|
||||
return x
|
||||
|
||||
|
||||
class MultiHeadAttention(nn.Module):
|
||||
def __init__(self, channels, out_channels, n_heads, p_dropout=0., window_size=None, heads_share=True, block_length=None, proximal_bias=False, proximal_init=False):
|
||||
super().__init__()
|
||||
assert channels % n_heads == 0
|
||||
|
||||
self.channels = channels
|
||||
self.out_channels = out_channels
|
||||
self.n_heads = n_heads
|
||||
self.p_dropout = p_dropout
|
||||
self.window_size = window_size
|
||||
self.heads_share = heads_share
|
||||
self.block_length = block_length
|
||||
self.proximal_bias = proximal_bias
|
||||
self.proximal_init = proximal_init
|
||||
self.attn = None
|
||||
|
||||
self.k_channels = channels // n_heads
|
||||
self.conv_q = nn.Conv1d(channels, channels, 1)
|
||||
self.conv_k = nn.Conv1d(channels, channels, 1)
|
||||
self.conv_v = nn.Conv1d(channels, channels, 1)
|
||||
self.conv_o = nn.Conv1d(channels, out_channels, 1)
|
||||
self.drop = nn.Dropout(p_dropout)
|
||||
|
||||
if window_size is not None:
|
||||
n_heads_rel = 1 if heads_share else n_heads
|
||||
rel_stddev = self.k_channels**-0.5
|
||||
self.emb_rel_k = nn.Parameter(torch.randn(n_heads_rel, window_size * 2 + 1, self.k_channels) * rel_stddev)
|
||||
self.emb_rel_v = nn.Parameter(torch.randn(n_heads_rel, window_size * 2 + 1, self.k_channels) * rel_stddev)
|
||||
|
||||
nn.init.xavier_uniform_(self.conv_q.weight)
|
||||
nn.init.xavier_uniform_(self.conv_k.weight)
|
||||
nn.init.xavier_uniform_(self.conv_v.weight)
|
||||
if proximal_init:
|
||||
with torch.no_grad():
|
||||
self.conv_k.weight.copy_(self.conv_q.weight)
|
||||
self.conv_k.bias.copy_(self.conv_q.bias)
|
||||
|
||||
def forward(self, x, c, attn_mask=None):
|
||||
q = self.conv_q(x)
|
||||
k = self.conv_k(c)
|
||||
v = self.conv_v(c)
|
||||
|
||||
x, self.attn = self.attention(q, k, v, mask=attn_mask)
|
||||
|
||||
x = self.conv_o(x)
|
||||
return x
|
||||
|
||||
def attention(self, query, key, value, mask=None):
|
||||
# reshape [b, d, t] -> [b, n_h, t, d_k]
|
||||
b, d, t_s, t_t = (*key.size(), query.size(2))
|
||||
query = query.view(b, self.n_heads, self.k_channels, t_t).transpose(2, 3)
|
||||
key = key.view(b, self.n_heads, self.k_channels, t_s).transpose(2, 3)
|
||||
value = value.view(b, self.n_heads, self.k_channels, t_s).transpose(2, 3)
|
||||
|
||||
scores = torch.matmul(query / math.sqrt(self.k_channels), key.transpose(-2, -1))
|
||||
if self.window_size is not None:
|
||||
assert t_s == t_t, "Relative attention is only available for self-attention."
|
||||
key_relative_embeddings = self._get_relative_embeddings(self.emb_rel_k, t_s)
|
||||
rel_logits = self._matmul_with_relative_keys(query /math.sqrt(self.k_channels), key_relative_embeddings)
|
||||
scores_local = self._relative_position_to_absolute_position(rel_logits)
|
||||
scores = scores + scores_local
|
||||
if self.proximal_bias:
|
||||
assert t_s == t_t, "Proximal bias is only available for self-attention."
|
||||
scores = scores + self._attention_bias_proximal(t_s).to(device=scores.device, dtype=scores.dtype)
|
||||
if mask is not None:
|
||||
scores = scores.masked_fill(mask == 0, -1e4)
|
||||
if self.block_length is not None:
|
||||
assert t_s == t_t, "Local attention is only available for self-attention."
|
||||
block_mask = torch.ones_like(scores).triu(-self.block_length).tril(self.block_length)
|
||||
scores = scores.masked_fill(block_mask == 0, -1e4)
|
||||
p_attn = F.softmax(scores, dim=-1) # [b, n_h, t_t, t_s]
|
||||
p_attn = self.drop(p_attn)
|
||||
output = torch.matmul(p_attn, value)
|
||||
if self.window_size is not None:
|
||||
relative_weights = self._absolute_position_to_relative_position(p_attn)
|
||||
value_relative_embeddings = self._get_relative_embeddings(self.emb_rel_v, t_s)
|
||||
output = output + self._matmul_with_relative_values(relative_weights, value_relative_embeddings)
|
||||
output = output.transpose(2, 3).contiguous().view(b, d, t_t) # [b, n_h, t_t, d_k] -> [b, d, t_t]
|
||||
return output, p_attn
|
||||
|
||||
def _matmul_with_relative_values(self, x, y):
|
||||
"""
|
||||
x: [b, h, l, m]
|
||||
y: [h or 1, m, d]
|
||||
ret: [b, h, l, d]
|
||||
"""
|
||||
ret = torch.matmul(x, y.unsqueeze(0))
|
||||
return ret
|
||||
|
||||
def _matmul_with_relative_keys(self, x, y):
|
||||
"""
|
||||
x: [b, h, l, d]
|
||||
y: [h or 1, m, d]
|
||||
ret: [b, h, l, m]
|
||||
"""
|
||||
ret = torch.matmul(x, y.unsqueeze(0).transpose(-2, -1))
|
||||
return ret
|
||||
|
||||
def _get_relative_embeddings(self, relative_embeddings, length):
|
||||
max_relative_position = 2 * self.window_size + 1
|
||||
# Pad first before slice to avoid using cond ops.
|
||||
pad_length = max(length - (self.window_size + 1), 0)
|
||||
slice_start_position = max((self.window_size + 1) - length, 0)
|
||||
slice_end_position = slice_start_position + 2 * length - 1
|
||||
if pad_length > 0:
|
||||
padded_relative_embeddings = F.pad(
|
||||
relative_embeddings,
|
||||
commons.convert_pad_shape([[0, 0], [pad_length, pad_length], [0, 0]]))
|
||||
else:
|
||||
padded_relative_embeddings = relative_embeddings
|
||||
used_relative_embeddings = padded_relative_embeddings[:,slice_start_position:slice_end_position]
|
||||
return used_relative_embeddings
|
||||
|
||||
def _relative_position_to_absolute_position(self, x):
|
||||
"""
|
||||
x: [b, h, l, 2*l-1]
|
||||
ret: [b, h, l, l]
|
||||
"""
|
||||
batch, heads, length, _ = x.size()
|
||||
# Concat columns of pad to shift from relative to absolute indexing.
|
||||
x = F.pad(x, commons.convert_pad_shape([[0,0],[0,0],[0,0],[0,1]]))
|
||||
|
||||
# Concat extra elements so to add up to shape (len+1, 2*len-1).
|
||||
x_flat = x.view([batch, heads, length * 2 * length])
|
||||
x_flat = F.pad(x_flat, commons.convert_pad_shape([[0,0],[0,0],[0,length-1]]))
|
||||
|
||||
# Reshape and slice out the padded elements.
|
||||
x_final = x_flat.view([batch, heads, length+1, 2*length-1])[:, :, :length, length-1:]
|
||||
return x_final
|
||||
|
||||
def _absolute_position_to_relative_position(self, x):
|
||||
"""
|
||||
x: [b, h, l, l]
|
||||
ret: [b, h, l, 2*l-1]
|
||||
"""
|
||||
batch, heads, length, _ = x.size()
|
||||
# padd along column
|
||||
x = F.pad(x, commons.convert_pad_shape([[0, 0], [0, 0], [0, 0], [0, length-1]]))
|
||||
x_flat = x.view([batch, heads, length**2 + length*(length -1)])
|
||||
# add 0's in the beginning that will skew the elements after reshape
|
||||
x_flat = F.pad(x_flat, commons.convert_pad_shape([[0, 0], [0, 0], [length, 0]]))
|
||||
x_final = x_flat.view([batch, heads, length, 2*length])[:,:,:,1:]
|
||||
return x_final
|
||||
|
||||
def _attention_bias_proximal(self, length):
|
||||
"""Bias for self-attention to encourage attention to close positions.
|
||||
Args:
|
||||
length: an integer scalar.
|
||||
Returns:
|
||||
a Tensor with shape [1, 1, length, length]
|
||||
"""
|
||||
r = torch.arange(length, dtype=torch.float32)
|
||||
diff = torch.unsqueeze(r, 0) - torch.unsqueeze(r, 1)
|
||||
return torch.unsqueeze(torch.unsqueeze(-torch.log1p(torch.abs(diff)), 0), 0)
|
||||
|
||||
|
||||
class FFN(nn.Module):
|
||||
def __init__(self, in_channels, out_channels, filter_channels, kernel_size, p_dropout=0., activation=None, causal=False):
|
||||
super().__init__()
|
||||
self.in_channels = in_channels
|
||||
self.out_channels = out_channels
|
||||
self.filter_channels = filter_channels
|
||||
self.kernel_size = kernel_size
|
||||
self.p_dropout = p_dropout
|
||||
self.activation = activation
|
||||
self.causal = causal
|
||||
|
||||
if causal:
|
||||
self.padding = self._causal_padding
|
||||
else:
|
||||
self.padding = self._same_padding
|
||||
|
||||
self.conv_1 = nn.Conv1d(in_channels, filter_channels, kernel_size)
|
||||
self.conv_2 = nn.Conv1d(filter_channels, out_channels, kernel_size)
|
||||
self.drop = nn.Dropout(p_dropout)
|
||||
|
||||
def forward(self, x, x_mask):
|
||||
x = self.conv_1(self.padding(x * x_mask))
|
||||
if self.activation == "gelu":
|
||||
x = x * torch.sigmoid(1.702 * x)
|
||||
else:
|
||||
x = torch.relu(x)
|
||||
x = self.drop(x)
|
||||
x = self.conv_2(self.padding(x * x_mask))
|
||||
return x * x_mask
|
||||
|
||||
def _causal_padding(self, x):
|
||||
if self.kernel_size == 1:
|
||||
return x
|
||||
pad_l = self.kernel_size - 1
|
||||
pad_r = 0
|
||||
padding = [[0, 0], [0, 0], [pad_l, pad_r]]
|
||||
x = F.pad(x, commons.convert_pad_shape(padding))
|
||||
return x
|
||||
|
||||
def _same_padding(self, x):
|
||||
if self.kernel_size == 1:
|
||||
return x
|
||||
pad_l = (self.kernel_size - 1) // 2
|
||||
pad_r = self.kernel_size // 2
|
||||
padding = [[0, 0], [0, 0], [pad_l, pad_r]]
|
||||
x = F.pad(x, commons.convert_pad_shape(padding))
|
||||
return x
|
161
demo/MMVC_Trainer/commons.py
Executable file
161
demo/MMVC_Trainer/commons.py
Executable file
@ -0,0 +1,161 @@
|
||||
import math
|
||||
import numpy as np
|
||||
import torch
|
||||
from torch import nn
|
||||
from torch.nn import functional as F
|
||||
|
||||
|
||||
def init_weights(m, mean=0.0, std=0.01):
|
||||
classname = m.__class__.__name__
|
||||
if classname.find("Conv") != -1:
|
||||
m.weight.data.normal_(mean, std)
|
||||
|
||||
|
||||
def get_padding(kernel_size, dilation=1):
|
||||
return int((kernel_size*dilation - dilation)/2)
|
||||
|
||||
|
||||
def convert_pad_shape(pad_shape):
|
||||
l = pad_shape[::-1]
|
||||
pad_shape = [item for sublist in l for item in sublist]
|
||||
return pad_shape
|
||||
|
||||
|
||||
def intersperse(lst, item):
|
||||
result = [item] * (len(lst) * 2 + 1)
|
||||
result[1::2] = lst
|
||||
return result
|
||||
|
||||
|
||||
def kl_divergence(m_p, logs_p, m_q, logs_q):
|
||||
"""KL(P||Q)"""
|
||||
kl = (logs_q - logs_p) - 0.5
|
||||
kl += 0.5 * (torch.exp(2. * logs_p) + ((m_p - m_q)**2)) * torch.exp(-2. * logs_q)
|
||||
return kl
|
||||
|
||||
|
||||
def rand_gumbel(shape):
|
||||
"""Sample from the Gumbel distribution, protect from overflows."""
|
||||
uniform_samples = torch.rand(shape) * 0.99998 + 0.00001
|
||||
return -torch.log(-torch.log(uniform_samples))
|
||||
|
||||
|
||||
def rand_gumbel_like(x):
|
||||
g = rand_gumbel(x.size()).to(dtype=x.dtype, device=x.device)
|
||||
return g
|
||||
|
||||
|
||||
def slice_segments(x, ids_str, segment_size=4):
|
||||
ret = torch.zeros_like(x[:, :, :segment_size])
|
||||
for i in range(x.size(0)):
|
||||
idx_str = ids_str[i]
|
||||
idx_end = idx_str + segment_size
|
||||
ret[i] = x[i, :, idx_str:idx_end]
|
||||
return ret
|
||||
|
||||
|
||||
def rand_slice_segments(x, x_lengths=None, segment_size=4):
|
||||
b, d, t = x.size()
|
||||
if x_lengths is None:
|
||||
x_lengths = t
|
||||
ids_str_max = x_lengths - segment_size + 1
|
||||
ids_str = (torch.rand([b]).to(device=x.device) * ids_str_max).to(dtype=torch.long)
|
||||
ret = slice_segments(x, ids_str, segment_size)
|
||||
return ret, ids_str
|
||||
|
||||
|
||||
def get_timing_signal_1d(
|
||||
length, channels, min_timescale=1.0, max_timescale=1.0e4):
|
||||
position = torch.arange(length, dtype=torch.float)
|
||||
num_timescales = channels // 2
|
||||
log_timescale_increment = (
|
||||
math.log(float(max_timescale) / float(min_timescale)) /
|
||||
(num_timescales - 1))
|
||||
inv_timescales = min_timescale * torch.exp(
|
||||
torch.arange(num_timescales, dtype=torch.float) * -log_timescale_increment)
|
||||
scaled_time = position.unsqueeze(0) * inv_timescales.unsqueeze(1)
|
||||
signal = torch.cat([torch.sin(scaled_time), torch.cos(scaled_time)], 0)
|
||||
signal = F.pad(signal, [0, 0, 0, channels % 2])
|
||||
signal = signal.view(1, channels, length)
|
||||
return signal
|
||||
|
||||
|
||||
def add_timing_signal_1d(x, min_timescale=1.0, max_timescale=1.0e4):
|
||||
b, channels, length = x.size()
|
||||
signal = get_timing_signal_1d(length, channels, min_timescale, max_timescale)
|
||||
return x + signal.to(dtype=x.dtype, device=x.device)
|
||||
|
||||
|
||||
def cat_timing_signal_1d(x, min_timescale=1.0, max_timescale=1.0e4, axis=1):
|
||||
b, channels, length = x.size()
|
||||
signal = get_timing_signal_1d(length, channels, min_timescale, max_timescale)
|
||||
return torch.cat([x, signal.to(dtype=x.dtype, device=x.device)], axis)
|
||||
|
||||
|
||||
def subsequent_mask(length):
|
||||
mask = torch.tril(torch.ones(length, length)).unsqueeze(0).unsqueeze(0)
|
||||
return mask
|
||||
|
||||
|
||||
@torch.jit.script
|
||||
def fused_add_tanh_sigmoid_multiply(input_a, input_b, n_channels):
|
||||
n_channels_int = n_channels[0]
|
||||
in_act = input_a + input_b
|
||||
t_act = torch.tanh(in_act[:, :n_channels_int, :])
|
||||
s_act = torch.sigmoid(in_act[:, n_channels_int:, :])
|
||||
acts = t_act * s_act
|
||||
return acts
|
||||
|
||||
|
||||
def convert_pad_shape(pad_shape):
|
||||
l = pad_shape[::-1]
|
||||
pad_shape = [item for sublist in l for item in sublist]
|
||||
return pad_shape
|
||||
|
||||
|
||||
def shift_1d(x):
|
||||
x = F.pad(x, convert_pad_shape([[0, 0], [0, 0], [1, 0]]))[:, :, :-1]
|
||||
return x
|
||||
|
||||
|
||||
def sequence_mask(length, max_length=None):
|
||||
if max_length is None:
|
||||
max_length = length.max()
|
||||
x = torch.arange(max_length, dtype=length.dtype, device=length.device)
|
||||
return x.unsqueeze(0) < length.unsqueeze(1)
|
||||
|
||||
|
||||
def generate_path(duration, mask):
|
||||
"""
|
||||
duration: [b, 1, t_x]
|
||||
mask: [b, 1, t_y, t_x]
|
||||
"""
|
||||
device = duration.device
|
||||
|
||||
b, _, t_y, t_x = mask.shape
|
||||
cum_duration = torch.cumsum(duration, -1)
|
||||
|
||||
cum_duration_flat = cum_duration.view(b * t_x)
|
||||
path = sequence_mask(cum_duration_flat, t_y).to(mask.dtype)
|
||||
path = path.view(b, t_x, t_y)
|
||||
path = path - F.pad(path, convert_pad_shape([[0, 0], [1, 0], [0, 0]]))[:, :-1]
|
||||
path = path.unsqueeze(1).transpose(2,3) * mask
|
||||
return path
|
||||
|
||||
|
||||
def clip_grad_value_(parameters, clip_value, norm_type=2):
|
||||
if isinstance(parameters, torch.Tensor):
|
||||
parameters = [parameters]
|
||||
parameters = list(filter(lambda p: p.grad is not None, parameters))
|
||||
norm_type = float(norm_type)
|
||||
if clip_value is not None:
|
||||
clip_value = float(clip_value)
|
||||
|
||||
total_norm = 0
|
||||
for p in parameters:
|
||||
param_norm = p.grad.data.norm(norm_type)
|
||||
total_norm += param_norm.item() ** norm_type
|
||||
if clip_value is not None:
|
||||
p.grad.data.clamp_(min=-clip_value, max=clip_value)
|
||||
total_norm = total_norm ** (1. / norm_type)
|
||||
return total_norm
|
110
demo/MMVC_Trainer/configs/baseconfig.json
Executable file
110
demo/MMVC_Trainer/configs/baseconfig.json
Executable file
@ -0,0 +1,110 @@
|
||||
{
|
||||
"train": {
|
||||
"log_interval": 1000,
|
||||
"eval_interval": 2000,
|
||||
"seed": 1234,
|
||||
"epochs": 10000,
|
||||
"learning_rate": 0.0002,
|
||||
"betas": [
|
||||
0.8,
|
||||
0.99
|
||||
],
|
||||
"eps": 1e-09,
|
||||
"batch_size": 10,
|
||||
"fp16_run": true,
|
||||
"lr_decay": 0.999875,
|
||||
"segment_size": 8192,
|
||||
"init_lr_ratio": 1,
|
||||
"warmup_epochs": 0,
|
||||
"c_mel": 45,
|
||||
"c_kl": 1.0
|
||||
},
|
||||
"data": {
|
||||
"training_files": "filelists/dec_not_propagation_label_and_change_melspec_textful.txt",
|
||||
"validation_files": "filelists/dec_not_propagation_label_and_change_melspec_textful_val.txt",
|
||||
"training_files_notext": "filelists/dec_not_propagation_label_and_change_melspec_textless.txt",
|
||||
"validation_files_notext": "filelists/dec_not_propagation_label_and_change_melspec_val_textless.txt",
|
||||
"text_cleaners": [
|
||||
"japanese_cleaners"
|
||||
],
|
||||
"max_wav_value": 32768.0,
|
||||
"sampling_rate": 24000,
|
||||
"filter_length": 512,
|
||||
"hop_length": 128,
|
||||
"win_length": 512,
|
||||
"n_mel_channels": 80,
|
||||
"mel_fmin": 0.0,
|
||||
"mel_fmax": null,
|
||||
"add_blank": true,
|
||||
"n_speakers": 110,
|
||||
"cleaned_text": false
|
||||
},
|
||||
"model": {
|
||||
"inter_channels": 192,
|
||||
"hidden_channels": 192,
|
||||
"filter_channels": 768,
|
||||
"n_heads": 2,
|
||||
"n_layers": 6,
|
||||
"kernel_size": 3,
|
||||
"p_dropout": 0.1,
|
||||
"resblock": "1",
|
||||
"resblock_kernel_sizes": [
|
||||
3,
|
||||
7,
|
||||
11
|
||||
],
|
||||
"resblock_dilation_sizes": [
|
||||
[
|
||||
1,
|
||||
3,
|
||||
5
|
||||
],
|
||||
[
|
||||
1,
|
||||
3,
|
||||
5
|
||||
],
|
||||
[
|
||||
1,
|
||||
3,
|
||||
5
|
||||
]
|
||||
],
|
||||
"upsample_rates": [
|
||||
8,
|
||||
4,
|
||||
2,
|
||||
2
|
||||
],
|
||||
"upsample_initial_channel": 512,
|
||||
"upsample_kernel_sizes": [
|
||||
16,
|
||||
16,
|
||||
8,
|
||||
8
|
||||
],
|
||||
"n_layers_q": 3,
|
||||
"use_spectral_norm": false,
|
||||
"n_flow": 8,
|
||||
"gin_channels": 256
|
||||
},
|
||||
"others": {
|
||||
"os_type": "linux"
|
||||
},
|
||||
"augmentation": {
|
||||
"enable" : true,
|
||||
"gain_p" : 0.5,
|
||||
"min_gain_in_db" : -10,
|
||||
"max_gain_in_db" : 10,
|
||||
"time_stretch_p" : 0.5,
|
||||
"min_rate" : 0.75,
|
||||
"max_rate" : 1.25,
|
||||
"pitch_shift_p" : 0.0,
|
||||
"min_semitones" : -4.0,
|
||||
"max_semitones" : 4.0,
|
||||
"add_gaussian_noise_p" : 0.0,
|
||||
"min_amplitude" : 0.001,
|
||||
"max_amplitude" : 0.04,
|
||||
"frequency_mask_p" : 0.0
|
||||
}
|
||||
}
|
343
demo/MMVC_Trainer/create_dataset_jtalk.py
Executable file
343
demo/MMVC_Trainer/create_dataset_jtalk.py
Executable file
@ -0,0 +1,343 @@
|
||||
import glob
|
||||
import sys
|
||||
import os
|
||||
import argparse
|
||||
import pyopenjtalk
|
||||
import json
|
||||
|
||||
def mozi2phone(mozi):
|
||||
text = pyopenjtalk.g2p(mozi)
|
||||
text = "sil " + text + " sil"
|
||||
text = text.replace(' ', '-')
|
||||
return text
|
||||
|
||||
def create_json(filename, num_speakers, sr, config_path):
|
||||
if os.path.exists(config_path):
|
||||
with open(config_path, "r", encoding="utf-8") as f:
|
||||
data = json.load(f)
|
||||
data['data']['training_files'] = 'filelists/' + filename + '_textful.txt'
|
||||
data['data']['validation_files'] = 'filelists/' + filename + '_textful_val.txt'
|
||||
data['data']['training_files_notext'] = 'filelists/' + filename + '_textless.txt'
|
||||
data['data']['validation_files_notext'] = 'filelists/' + filename + '_val_textless.txt'
|
||||
data['data']['sampling_rate'] = sr
|
||||
data['data']['n_speakers'] = num_speakers
|
||||
|
||||
with open("./configs/" + filename + ".json", 'w', encoding='utf-8') as f:
|
||||
json.dump(data, f, indent=2, ensure_ascii=False)
|
||||
|
||||
def create_dataset(filename):
|
||||
speaker_id = 107
|
||||
textful_dir_list = glob.glob("dataset/textful/*")
|
||||
textless_dir_list = glob.glob("dataset/textless/*")
|
||||
textful_dir_list.sort()
|
||||
textless_dir_list.sort()
|
||||
Correspondence_list = list()
|
||||
output_file_list = list()
|
||||
output_file_list_val = list()
|
||||
output_file_list_textless = list()
|
||||
output_file_list_val_textless = list()
|
||||
for d in textful_dir_list:
|
||||
wav_file_list = glob.glob(d+"/wav/*.wav")
|
||||
lab_file_list = glob.glob(d + "/text/*.txt")
|
||||
wav_file_list.sort()
|
||||
lab_file_list.sort()
|
||||
if len(wav_file_list) == 0:
|
||||
continue
|
||||
counter = 0
|
||||
for lab, wav in zip(lab_file_list, wav_file_list):
|
||||
with open(lab, 'r', encoding="utf-8") as f:
|
||||
mozi = f.read().split("\n")
|
||||
print(str(mozi))
|
||||
test = mozi2phone(str(mozi))
|
||||
print(test)
|
||||
print(wav + "|"+ str(speaker_id) + "|"+ test)
|
||||
if counter % 10 != 0:
|
||||
output_file_list.append(wav + "|"+ str(speaker_id) + "|"+ test + "\n")
|
||||
else:
|
||||
output_file_list_val.append(wav + "|"+ str(speaker_id) + "|"+ test + "\n")
|
||||
counter = counter +1
|
||||
Correspondence_list.append(str(speaker_id)+"|"+os.path.basename(d) + "\n")
|
||||
speaker_id = speaker_id + 1
|
||||
if speaker_id > 108:
|
||||
break
|
||||
|
||||
for d in textless_dir_list:
|
||||
wav_file_list = glob.glob(d+"/*.wav")
|
||||
wav_file_list.sort()
|
||||
counter = 0
|
||||
for wav in wav_file_list:
|
||||
print(wav + "|"+ str(speaker_id) + "|a")
|
||||
if counter % 10 != 0:
|
||||
output_file_list_textless.append(wav + "|"+ str(speaker_id) + "|a" + "\n")
|
||||
else:
|
||||
output_file_list_val_textless.append(wav + "|"+ str(speaker_id) + "|a" + "\n")
|
||||
counter = counter +1
|
||||
Correspondence_list.append(str(speaker_id)+"|"+os.path.basename(d) + "\n")
|
||||
speaker_id = speaker_id + 1
|
||||
|
||||
with open('filelists/' + filename + '_textful.txt', 'w', encoding='utf-8', newline='\n') as f:
|
||||
f.writelines(output_file_list)
|
||||
with open('filelists/' + filename + '_textful_val.txt', 'w', encoding='utf-8', newline='\n') as f:
|
||||
f.writelines(output_file_list_val)
|
||||
with open('filelists/' + filename + '_textless.txt', 'w', encoding='utf-8', newline='\n') as f:
|
||||
f.writelines(output_file_list_textless)
|
||||
with open('filelists/' + filename + '_val_textless.txt', 'w', encoding='utf-8', newline='\n') as f:
|
||||
f.writelines(output_file_list_val_textless)
|
||||
with open('filelists/' + filename + '_Correspondence.txt', 'w', encoding='utf-8', newline='\n') as f:
|
||||
f.writelines(Correspondence_list)
|
||||
return speaker_id
|
||||
|
||||
def create_dataset_zundamon(filename):
|
||||
textful_dir_list = glob.glob("dataset/textful/*")
|
||||
textless_dir_list = glob.glob("dataset/textless/*")
|
||||
textful_dir_list.sort()
|
||||
textless_dir_list.sort()
|
||||
Correspondence_list = list()
|
||||
output_file_list = list()
|
||||
output_file_list_val = list()
|
||||
output_file_list_textless = list()
|
||||
output_file_list_val_textless = list()
|
||||
#paths
|
||||
my_path = "dataset/textful/00_myvoice"
|
||||
zundamon_path = "dataset/textful/1205_zundamon"
|
||||
|
||||
#set list wav and text
|
||||
#myvoice
|
||||
speaker_id = 107
|
||||
d = my_path
|
||||
wav_file_list = glob.glob(d + "/wav/*.wav")
|
||||
lab_file_list = glob.glob(d + "/text/*.txt")
|
||||
wav_file_list.sort()
|
||||
lab_file_list.sort()
|
||||
if len(wav_file_list) == 0:
|
||||
print("Error" + d + "/wav に音声データがありません")
|
||||
exit()
|
||||
counter = 0
|
||||
for lab, wav in zip(lab_file_list, wav_file_list):
|
||||
with open(lab, 'r', encoding="utf-8") as f:
|
||||
mozi = f.read().split("\n")
|
||||
print(str(mozi))
|
||||
test = mozi2phone(str(mozi))
|
||||
print(test)
|
||||
print(wav + "|"+ str(speaker_id) + "|"+ test)
|
||||
if counter % 10 != 0:
|
||||
output_file_list.append(wav + "|"+ str(speaker_id) + "|"+ test + "\n")
|
||||
else:
|
||||
output_file_list_val.append(wav + "|"+ str(speaker_id) + "|"+ test + "\n")
|
||||
counter = counter +1
|
||||
Correspondence_list.append(str(speaker_id)+"|"+os.path.basename(d) + "\n")
|
||||
|
||||
speaker_id = 100
|
||||
d = zundamon_path
|
||||
wav_file_list = glob.glob(d + "/wav/*.wav")
|
||||
lab_file_list = glob.glob(d + "/text/*.txt")
|
||||
wav_file_list.sort()
|
||||
lab_file_list.sort()
|
||||
if len(wav_file_list) == 0:
|
||||
print("Error" + d + "/wav に音声データがありません")
|
||||
exit()
|
||||
counter = 0
|
||||
for lab, wav in zip(lab_file_list, wav_file_list):
|
||||
with open(lab, 'r', encoding="utf-8") as f:
|
||||
mozi = f.read().split("\n")
|
||||
print(str(mozi))
|
||||
test = mozi2phone(str(mozi))
|
||||
print(test)
|
||||
print(wav + "|"+ str(speaker_id) + "|"+ test)
|
||||
if counter % 10 != 0:
|
||||
output_file_list.append(wav + "|"+ str(speaker_id) + "|"+ test + "\n")
|
||||
else:
|
||||
output_file_list_val.append(wav + "|"+ str(speaker_id) + "|"+ test + "\n")
|
||||
counter = counter +1
|
||||
Correspondence_list.append(str(speaker_id)+"|"+os.path.basename(d) + "\n")
|
||||
|
||||
for d in textless_dir_list:
|
||||
wav_file_list = glob.glob(d+"/*.wav")
|
||||
wav_file_list.sort()
|
||||
counter = 0
|
||||
for wav in wav_file_list:
|
||||
print(wav + "|"+ str(speaker_id) + "|a")
|
||||
if counter % 10 != 0:
|
||||
output_file_list_textless.append(wav + "|"+ str(speaker_id) + "|a" + "\n")
|
||||
else:
|
||||
output_file_list_val_textless.append(wav + "|"+ str(speaker_id) + "|a" + "\n")
|
||||
counter = counter +1
|
||||
Correspondence_list.append(str(speaker_id)+"|"+os.path.basename(d) + "\n")
|
||||
speaker_id = speaker_id + 1
|
||||
|
||||
with open('filelists/' + filename + '_textful.txt', 'w', encoding='utf-8', newline='\n') as f:
|
||||
f.writelines(output_file_list)
|
||||
with open('filelists/' + filename + '_textful_val.txt', 'w', encoding='utf-8', newline='\n') as f:
|
||||
f.writelines(output_file_list_val)
|
||||
with open('filelists/' + filename + '_textless.txt', 'w', encoding='utf-8', newline='\n') as f:
|
||||
f.writelines(output_file_list_textless)
|
||||
with open('filelists/' + filename + '_val_textless.txt', 'w', encoding='utf-8', newline='\n') as f:
|
||||
f.writelines(output_file_list_val_textless)
|
||||
with open('filelists/' + filename + '_Correspondence.txt', 'w', encoding='utf-8', newline='\n') as f:
|
||||
f.writelines(Correspondence_list)
|
||||
return 110
|
||||
|
||||
def create_dataset_character(filename, tid):
|
||||
textful_dir_list = glob.glob("dataset/textful/*")
|
||||
textless_dir_list = glob.glob("dataset/textless/*")
|
||||
textful_dir_list.sort()
|
||||
textless_dir_list.sort()
|
||||
Correspondence_list = list()
|
||||
output_file_list = list()
|
||||
output_file_list_val = list()
|
||||
output_file_list_textless = list()
|
||||
output_file_list_val_textless = list()
|
||||
#paths
|
||||
my_path = "dataset/textful/00_myvoice"
|
||||
zundamon_path = "dataset/textful/01_target"
|
||||
|
||||
#set list wav and text
|
||||
#myvoice
|
||||
speaker_id = 107
|
||||
d = my_path
|
||||
wav_file_list = glob.glob(d + "/wav/*.wav")
|
||||
lab_file_list = glob.glob(d + "/text/*.txt")
|
||||
wav_file_list.sort()
|
||||
lab_file_list.sort()
|
||||
if len(wav_file_list) == 0:
|
||||
print("Error" + d + "/wav に音声データがありません")
|
||||
exit()
|
||||
counter = 0
|
||||
for lab, wav in zip(lab_file_list, wav_file_list):
|
||||
with open(lab, 'r', encoding="utf-8") as f:
|
||||
mozi = f.read().split("\n")
|
||||
print(str(mozi))
|
||||
test = mozi2phone(str(mozi))
|
||||
print(test)
|
||||
print(wav + "|"+ str(speaker_id) + "|"+ test)
|
||||
if counter % 10 != 0:
|
||||
output_file_list.append(wav + "|"+ str(speaker_id) + "|"+ test + "\n")
|
||||
else:
|
||||
output_file_list_val.append(wav + "|"+ str(speaker_id) + "|"+ test + "\n")
|
||||
counter = counter +1
|
||||
Correspondence_list.append(str(speaker_id)+"|"+os.path.basename(d) + "\n")
|
||||
|
||||
speaker_id = tid
|
||||
d = zundamon_path
|
||||
wav_file_list = glob.glob(d + "/wav/*.wav")
|
||||
lab_file_list = glob.glob(d + "/text/*.txt")
|
||||
wav_file_list.sort()
|
||||
lab_file_list.sort()
|
||||
if len(wav_file_list) == 0:
|
||||
print("Error" + d + "/wav に音声データがありません")
|
||||
exit()
|
||||
counter = 0
|
||||
for lab, wav in zip(lab_file_list, wav_file_list):
|
||||
with open(lab, 'r', encoding="utf-8") as f:
|
||||
mozi = f.read().split("\n")
|
||||
print(str(mozi))
|
||||
test = mozi2phone(str(mozi))
|
||||
print(test)
|
||||
print(wav + "|"+ str(speaker_id) + "|"+ test)
|
||||
if counter % 10 != 0:
|
||||
output_file_list.append(wav + "|"+ str(speaker_id) + "|"+ test + "\n")
|
||||
else:
|
||||
output_file_list_val.append(wav + "|"+ str(speaker_id) + "|"+ test + "\n")
|
||||
counter = counter +1
|
||||
Correspondence_list.append(str(speaker_id)+"|"+os.path.basename(d) + "\n")
|
||||
|
||||
for d in textless_dir_list:
|
||||
wav_file_list = glob.glob(d+"/*.wav")
|
||||
wav_file_list.sort()
|
||||
counter = 0
|
||||
for wav in wav_file_list:
|
||||
print(wav + "|"+ str(speaker_id) + "|a")
|
||||
if counter % 10 != 0:
|
||||
output_file_list_textless.append(wav + "|"+ str(speaker_id) + "|a" + "\n")
|
||||
else:
|
||||
output_file_list_val_textless.append(wav + "|"+ str(speaker_id) + "|a" + "\n")
|
||||
counter = counter +1
|
||||
Correspondence_list.append(str(speaker_id)+"|"+os.path.basename(d) + "\n")
|
||||
speaker_id = speaker_id + 1
|
||||
|
||||
with open('filelists/' + filename + '_textful.txt', 'w', encoding='utf-8', newline='\n') as f:
|
||||
f.writelines(output_file_list)
|
||||
with open('filelists/' + filename + '_textful_val.txt', 'w', encoding='utf-8', newline='\n') as f:
|
||||
f.writelines(output_file_list_val)
|
||||
with open('filelists/' + filename + '_textless.txt', 'w', encoding='utf-8', newline='\n') as f:
|
||||
f.writelines(output_file_list_textless)
|
||||
with open('filelists/' + filename + '_val_textless.txt', 'w', encoding='utf-8', newline='\n') as f:
|
||||
f.writelines(output_file_list_val_textless)
|
||||
with open('filelists/' + filename + '_Correspondence.txt', 'w', encoding='utf-8', newline='\n') as f:
|
||||
f.writelines(Correspondence_list)
|
||||
return 110
|
||||
|
||||
def create_dataset_multi_character(filename, file_path):
|
||||
Correspondence_list = list()
|
||||
textless_dir_list = glob.glob("dataset/textless/*")
|
||||
textless_dir_list.sort()
|
||||
output_file_list = list()
|
||||
output_file_list_val = list()
|
||||
output_file_list_textless = list()
|
||||
output_file_list_val_textless = list()
|
||||
with open(file_path, "r") as f:
|
||||
for line in f.readlines():
|
||||
target_dir , sid = line.split("|")
|
||||
sid = sid.rstrip('\n')
|
||||
wav_file_list = glob.glob("dataset/textful/" + target_dir + "/wav/*.wav")
|
||||
lab_file_list = glob.glob("dataset/textful/" + target_dir + "/text/*.txt")
|
||||
wav_file_list.sort()
|
||||
lab_file_list.sort()
|
||||
if len(wav_file_list) == 0:
|
||||
print("Error" + target_dir + "/wav に音声データがありません")
|
||||
exit()
|
||||
counter = 0
|
||||
for lab, wav in zip(lab_file_list, wav_file_list):
|
||||
with open(lab, 'r', encoding="utf-8") as f_text:
|
||||
mozi = f_text.read().split("\n")
|
||||
print(str(mozi))
|
||||
test = mozi2phone(str(mozi))
|
||||
print(test)
|
||||
print(wav + "|"+ str(sid) + "|"+ test)
|
||||
if counter % 10 != 0:
|
||||
output_file_list.append(wav + "|"+ str(sid) + "|"+ test + "\n")
|
||||
else:
|
||||
output_file_list_val.append(wav + "|"+ str(sid) + "|"+ test + "\n")
|
||||
counter = counter +1
|
||||
Correspondence_list.append(str(sid)+"|"+ target_dir + "\n")
|
||||
|
||||
with open('filelists/' + filename + '_textful.txt', 'w', encoding='utf-8', newline='\n') as f:
|
||||
f.writelines(output_file_list)
|
||||
with open('filelists/' + filename + '_textful_val.txt', 'w', encoding='utf-8', newline='\n') as f:
|
||||
f.writelines(output_file_list_val)
|
||||
with open('filelists/' + filename + '_textless.txt', 'w', encoding='utf-8', newline='\n') as f:
|
||||
f.writelines(output_file_list_textless)
|
||||
with open('filelists/' + filename + '_val_textless.txt', 'w', encoding='utf-8', newline='\n') as f:
|
||||
f.writelines(output_file_list_val_textless)
|
||||
with open('filelists/' + filename + '_Correspondence.txt', 'w', encoding='utf-8', newline='\n') as f:
|
||||
f.writelines(Correspondence_list)
|
||||
return 110
|
||||
|
||||
def main():
|
||||
parser = argparse.ArgumentParser()
|
||||
parser.add_argument('-f', '--filename', type=str, required=True,
|
||||
help='filelist for configuration')
|
||||
parser.add_argument('-s', '--sr', type=int, default=24000,
|
||||
help='sampling rate (default = 24000)')
|
||||
parser.add_argument('-t', '--target', type=int, default=9999,
|
||||
help='pre_traind targetid (zundamon = 100, sora = 101, methane = 102, tsumugi = 103)')
|
||||
parser.add_argument('-m', '--multi_target', type=str, default=None,
|
||||
help='pre_traind targetid (zundamon = 100, sora = 101, methane = 102, tsumugi = 103)')
|
||||
parser.add_argument('-c', '--config', type=str, default="./configs/baseconfig.json",
|
||||
help='JSON file for configuration')
|
||||
args = parser.parse_args()
|
||||
filename = args.filename
|
||||
print(filename)
|
||||
if args.multi_target != None:
|
||||
n_spk = create_dataset_multi_character(filename, args.multi_target)
|
||||
elif args.target != 9999 and args.target == 100:
|
||||
n_spk = create_dataset_zundamon(filename)
|
||||
elif args.target != 9999:
|
||||
n_spk = create_dataset_character(filename, args.target)
|
||||
else:
|
||||
n_spk = create_dataset(filename)
|
||||
|
||||
create_json(filename, n_spk, args.sr, args.config)
|
||||
|
||||
if __name__ == '__main__':
|
||||
main()
|
305
demo/MMVC_Trainer/create_dataset_jtalk_feature.py
Executable file
305
demo/MMVC_Trainer/create_dataset_jtalk_feature.py
Executable file
@ -0,0 +1,305 @@
|
||||
import glob
|
||||
import sys
|
||||
import os
|
||||
import argparse
|
||||
import pyopenjtalk
|
||||
import json
|
||||
|
||||
def mozi2phone(mozi):
|
||||
text = pyopenjtalk.g2p(mozi)
|
||||
text = "sil " + text + " sil"
|
||||
text = text.replace(' ', '-')
|
||||
return text
|
||||
|
||||
def create_json(filename, num_speakers, sr, config_path):
|
||||
if os.path.exists(config_path):
|
||||
with open(config_path, "r", encoding="utf-8") as f:
|
||||
data = json.load(f)
|
||||
data['data']['training_files'] = 'filelists/' + filename + '_textful.txt'
|
||||
data['data']['validation_files'] = 'filelists/' + filename + '_textful_val.txt'
|
||||
data['data']['training_files_notext'] = 'filelists/' + filename + '_textless.txt'
|
||||
data['data']['validation_files_notext'] = 'filelists/' + filename + '_val_textless.txt'
|
||||
data['data']['sampling_rate'] = sr
|
||||
data['data']['n_speakers'] = num_speakers
|
||||
|
||||
with open("./configs/" + filename + ".json", 'w', encoding='utf-8') as f:
|
||||
json.dump(data, f, indent=2, ensure_ascii=False)
|
||||
|
||||
def create_dataset(filename, my_sid):
|
||||
speaker_id = my_sid
|
||||
textful_dir_list = glob.glob("dataset/textful/*")
|
||||
textless_dir_list = glob.glob("dataset/textless/*")
|
||||
textful_dir_list.sort()
|
||||
textless_dir_list.sort()
|
||||
Correspondence_list = list()
|
||||
output_file_list = list()
|
||||
output_file_list_val = list()
|
||||
output_file_list_textless = list()
|
||||
output_file_list_val_textless = list()
|
||||
for d in textful_dir_list:
|
||||
wav_file_list = glob.glob(d+"/wav/*.wav")
|
||||
lab_file_list = glob.glob(d + "/text/*.txt")
|
||||
wav_file_list.sort()
|
||||
lab_file_list.sort()
|
||||
if len(wav_file_list) == 0:
|
||||
continue
|
||||
counter = 0
|
||||
for lab, wav in zip(lab_file_list, wav_file_list):
|
||||
with open(lab, 'r', encoding="utf-8") as f:
|
||||
mozi = f.read().split("\n")
|
||||
print(str(mozi))
|
||||
test = mozi2phone(str(mozi))
|
||||
print(test)
|
||||
print(wav + "|"+ str(speaker_id) + "|"+ test)
|
||||
if counter % 10 != 0:
|
||||
output_file_list.append(wav + "|"+ str(speaker_id) + "|"+ test + "\n")
|
||||
else:
|
||||
output_file_list_val.append(wav + "|"+ str(speaker_id) + "|"+ test + "\n")
|
||||
counter = counter +1
|
||||
Correspondence_list.append(str(speaker_id)+"|"+os.path.basename(d) + "\n")
|
||||
speaker_id = speaker_id + 1
|
||||
if speaker_id > 108:
|
||||
break
|
||||
|
||||
for d in textless_dir_list:
|
||||
wav_file_list = glob.glob(d+"/*.wav")
|
||||
wav_file_list.sort()
|
||||
counter = 0
|
||||
for wav in wav_file_list:
|
||||
print(wav + "|"+ str(speaker_id) + "|a")
|
||||
if counter % 10 != 0:
|
||||
output_file_list_textless.append(wav + "|"+ str(speaker_id) + "|a" + "\n")
|
||||
else:
|
||||
output_file_list_val_textless.append(wav + "|"+ str(speaker_id) + "|a" + "\n")
|
||||
counter = counter +1
|
||||
Correspondence_list.append(str(speaker_id)+"|"+os.path.basename(d) + "\n")
|
||||
speaker_id = speaker_id + 1
|
||||
|
||||
with open('filelists/' + filename + '_textful.txt', 'w', encoding='utf-8', newline='\n') as f:
|
||||
f.writelines(output_file_list)
|
||||
with open('filelists/' + filename + '_textful_val.txt', 'w', encoding='utf-8', newline='\n') as f:
|
||||
f.writelines(output_file_list_val)
|
||||
with open('filelists/' + filename + '_textless.txt', 'w', encoding='utf-8', newline='\n') as f:
|
||||
f.writelines(output_file_list_textless)
|
||||
with open('filelists/' + filename + '_val_textless.txt', 'w', encoding='utf-8', newline='\n') as f:
|
||||
f.writelines(output_file_list_val_textless)
|
||||
with open('filelists/' + filename + '_Correspondence.txt', 'w', encoding='utf-8', newline='\n') as f:
|
||||
f.writelines(Correspondence_list)
|
||||
return speaker_id + 1
|
||||
|
||||
def create_dataset(filename, my_sid):
|
||||
textful_dir_list = glob.glob("dataset/textful/*")
|
||||
textless_dir_list = glob.glob("dataset/textless/*")
|
||||
textful_dir_list.sort()
|
||||
textless_dir_list.sort()
|
||||
Correspondence_list = list()
|
||||
output_file_list = list()
|
||||
output_file_list_val = list()
|
||||
output_file_list_textless = list()
|
||||
output_file_list_val_textless = list()
|
||||
#paths
|
||||
my_path = "dataset/textful/00_myvoice"
|
||||
target_path = "dataset/textful/01_target"
|
||||
print("myvoice : {}".format(str(os.path.isdir(my_path))))
|
||||
print("target_path : {}".format(str(os.path.isdir(target_path))))
|
||||
|
||||
#set list wav and text
|
||||
#myvoice
|
||||
speaker_id = my_sid
|
||||
d = my_path
|
||||
wav_file_list = glob.glob(d + "/wav/*.wav")
|
||||
lab_file_list = glob.glob(d + "/text/*.txt")
|
||||
wav_file_list.sort()
|
||||
lab_file_list.sort()
|
||||
if len(wav_file_list) == 0:
|
||||
print("Error" + d + "/wav に音声データがありません")
|
||||
exit()
|
||||
if len(lab_file_list) == 0:
|
||||
print("Error : " + d + "/text にテキストデータがありません")
|
||||
exit()
|
||||
counter = 0
|
||||
|
||||
for lab, wav in zip(lab_file_list, wav_file_list):
|
||||
with open(lab, 'r', encoding="utf-8") as f:
|
||||
mozi = f.read().split("\n")
|
||||
print(str(mozi))
|
||||
test = mozi2phone(str(mozi))
|
||||
print(test)
|
||||
print(wav + "|"+ str(speaker_id) + "|"+ test)
|
||||
if counter % 10 != 0:
|
||||
output_file_list.append(wav + "|"+ str(speaker_id) + "|"+ test + "\n")
|
||||
else:
|
||||
output_file_list_val.append(wav + "|"+ str(speaker_id) + "|"+ test + "\n")
|
||||
counter = counter +1
|
||||
Correspondence_list.append(str(speaker_id)+"|"+os.path.basename(d) + "\n")
|
||||
|
||||
speaker_id = 108
|
||||
d = target_path
|
||||
wav_file_list = glob.glob(d + "/wav/*.wav")
|
||||
lab_file_list = glob.glob(d + "/text/*.txt")
|
||||
wav_file_list.sort()
|
||||
lab_file_list.sort()
|
||||
if len(wav_file_list) == 0:
|
||||
print("Error" + d + "/wav に音声データがありません")
|
||||
exit()
|
||||
counter = 0
|
||||
for lab, wav in zip(lab_file_list, wav_file_list):
|
||||
with open(lab, 'r', encoding="utf-8") as f:
|
||||
mozi = f.read().split("\n")
|
||||
print(str(mozi))
|
||||
test = mozi2phone(str(mozi))
|
||||
print(test)
|
||||
print(wav + "|"+ str(speaker_id) + "|"+ test)
|
||||
if counter % 10 != 0:
|
||||
output_file_list.append(wav + "|"+ str(speaker_id) + "|"+ test + "\n")
|
||||
else:
|
||||
output_file_list_val.append(wav + "|"+ str(speaker_id) + "|"+ test + "\n")
|
||||
counter = counter +1
|
||||
Correspondence_list.append(str(speaker_id)+"|"+os.path.basename(d) + "\n")
|
||||
|
||||
for d in textless_dir_list:
|
||||
wav_file_list = glob.glob(d+"/*.wav")
|
||||
wav_file_list.sort()
|
||||
counter = 0
|
||||
for wav in wav_file_list:
|
||||
print(wav + "|"+ str(speaker_id) + "|a")
|
||||
if counter % 10 != 0:
|
||||
output_file_list_textless.append(wav + "|"+ str(speaker_id) + "|a" + "\n")
|
||||
else:
|
||||
output_file_list_val_textless.append(wav + "|"+ str(speaker_id) + "|a" + "\n")
|
||||
counter = counter +1
|
||||
Correspondence_list.append(str(speaker_id)+"|"+os.path.basename(d) + "\n")
|
||||
speaker_id = speaker_id + 1
|
||||
|
||||
with open('filelists/' + filename + '_textful.txt', 'w', encoding='utf-8', newline='\n') as f:
|
||||
f.writelines(output_file_list)
|
||||
with open('filelists/' + filename + '_textful_val.txt', 'w', encoding='utf-8', newline='\n') as f:
|
||||
f.writelines(output_file_list_val)
|
||||
with open('filelists/' + filename + '_textless.txt', 'w', encoding='utf-8', newline='\n') as f:
|
||||
f.writelines(output_file_list_textless)
|
||||
with open('filelists/' + filename + '_val_textless.txt', 'w', encoding='utf-8', newline='\n') as f:
|
||||
f.writelines(output_file_list_val_textless)
|
||||
with open('filelists/' + filename + '_Correspondence.txt', 'w', encoding='utf-8', newline='\n') as f:
|
||||
f.writelines(Correspondence_list)
|
||||
return 110
|
||||
|
||||
def create_dataset_zundamon(filename, my_sid):
|
||||
textful_dir_list = glob.glob("dataset/textful/*")
|
||||
textless_dir_list = glob.glob("dataset/textless/*")
|
||||
textful_dir_list.sort()
|
||||
textless_dir_list.sort()
|
||||
Correspondence_list = list()
|
||||
output_file_list = list()
|
||||
output_file_list_val = list()
|
||||
output_file_list_textless = list()
|
||||
output_file_list_val_textless = list()
|
||||
#paths
|
||||
my_path = "dataset/textful/00_myvoice"
|
||||
zundamon_path = "dataset/textful/1205_zundamon"
|
||||
print("myvoice : {}".format(str(os.path.isdir(my_path))))
|
||||
print("zundamon_path : {}".format(str(os.path.isdir(zundamon_path))))
|
||||
|
||||
#set list wav and text
|
||||
#myvoice
|
||||
speaker_id = my_sid
|
||||
d = my_path
|
||||
wav_file_list = glob.glob(d + "/wav/*.wav")
|
||||
lab_file_list = glob.glob(d + "/text/*.txt")
|
||||
wav_file_list.sort()
|
||||
lab_file_list.sort()
|
||||
if len(wav_file_list) == 0:
|
||||
print("Error" + d + "/wav に音声データがありません")
|
||||
exit()
|
||||
if len(lab_file_list) == 0:
|
||||
print("Error : " + d + "/text にテキストデータがありません")
|
||||
exit()
|
||||
counter = 0
|
||||
|
||||
for lab, wav in zip(lab_file_list, wav_file_list):
|
||||
with open(lab, 'r', encoding="utf-8") as f:
|
||||
mozi = f.read().split("\n")
|
||||
print(str(mozi))
|
||||
test = mozi2phone(str(mozi))
|
||||
print(test)
|
||||
print(wav + "|"+ str(speaker_id) + "|"+ test)
|
||||
if counter % 10 != 0:
|
||||
output_file_list.append(wav + "|"+ str(speaker_id) + "|"+ test + "\n")
|
||||
else:
|
||||
output_file_list_val.append(wav + "|"+ str(speaker_id) + "|"+ test + "\n")
|
||||
counter = counter +1
|
||||
Correspondence_list.append(str(speaker_id)+"|"+os.path.basename(d) + "\n")
|
||||
|
||||
speaker_id = 100
|
||||
d = zundamon_path
|
||||
wav_file_list = glob.glob(d + "/wav/*.wav")
|
||||
lab_file_list = glob.glob(d + "/text/*.txt")
|
||||
wav_file_list.sort()
|
||||
lab_file_list.sort()
|
||||
if len(wav_file_list) == 0:
|
||||
print("Error" + d + "/wav に音声データがありません")
|
||||
exit()
|
||||
counter = 0
|
||||
for lab, wav in zip(lab_file_list, wav_file_list):
|
||||
with open(lab, 'r', encoding="utf-8") as f:
|
||||
mozi = f.read().split("\n")
|
||||
print(str(mozi))
|
||||
test = mozi2phone(str(mozi))
|
||||
print(test)
|
||||
print(wav + "|"+ str(speaker_id) + "|"+ test)
|
||||
if counter % 10 != 0:
|
||||
output_file_list.append(wav + "|"+ str(speaker_id) + "|"+ test + "\n")
|
||||
else:
|
||||
output_file_list_val.append(wav + "|"+ str(speaker_id) + "|"+ test + "\n")
|
||||
counter = counter +1
|
||||
Correspondence_list.append(str(speaker_id)+"|"+os.path.basename(d) + "\n")
|
||||
|
||||
for d in textless_dir_list:
|
||||
wav_file_list = glob.glob(d+"/*.wav")
|
||||
wav_file_list.sort()
|
||||
counter = 0
|
||||
for wav in wav_file_list:
|
||||
print(wav + "|"+ str(speaker_id) + "|a")
|
||||
if counter % 10 != 0:
|
||||
output_file_list_textless.append(wav + "|"+ str(speaker_id) + "|a" + "\n")
|
||||
else:
|
||||
output_file_list_val_textless.append(wav + "|"+ str(speaker_id) + "|a" + "\n")
|
||||
counter = counter +1
|
||||
Correspondence_list.append(str(speaker_id)+"|"+os.path.basename(d) + "\n")
|
||||
speaker_id = speaker_id + 1
|
||||
|
||||
with open('filelists/' + filename + '_textful.txt', 'w', encoding='utf-8', newline='\n') as f:
|
||||
f.writelines(output_file_list)
|
||||
with open('filelists/' + filename + '_textful_val.txt', 'w', encoding='utf-8', newline='\n') as f:
|
||||
f.writelines(output_file_list_val)
|
||||
with open('filelists/' + filename + '_textless.txt', 'w', encoding='utf-8', newline='\n') as f:
|
||||
f.writelines(output_file_list_textless)
|
||||
with open('filelists/' + filename + '_val_textless.txt', 'w', encoding='utf-8', newline='\n') as f:
|
||||
f.writelines(output_file_list_val_textless)
|
||||
with open('filelists/' + filename + '_Correspondence.txt', 'w', encoding='utf-8', newline='\n') as f:
|
||||
f.writelines(Correspondence_list)
|
||||
return 110
|
||||
|
||||
def main():
|
||||
parser = argparse.ArgumentParser()
|
||||
parser.add_argument('-f', '--filename', type=str, required=True,
|
||||
help='filelist for configuration')
|
||||
parser.add_argument('-s', '--sr', type=int, default=24000,
|
||||
help='sampling rate (default = 24000)')
|
||||
parser.add_argument('-m', '--mysid', type=int, default=107,
|
||||
help='sampling rate (default = 24000)')
|
||||
parser.add_argument('-z', '--zundamon', type=bool, default=False,
|
||||
help='U.N. zundamon Was Her? (default = False)')
|
||||
parser.add_argument('-c', '--config', type=str, default="./configs/baseconfig.json",
|
||||
help='JSON file for configuration')
|
||||
args = parser.parse_args()
|
||||
filename = args.filename
|
||||
print(filename)
|
||||
if args.zundamon:
|
||||
n_spk = create_dataset_zundamon(filename, args.mysid)
|
||||
else:
|
||||
n_spk = create_dataset(filename, args.mysid)
|
||||
|
||||
create_json(filename, n_spk, args.sr, args.config)
|
||||
|
||||
if __name__ == '__main__':
|
||||
main()
|
87
demo/MMVC_Trainer/create_dataset_julius.py
Executable file
87
demo/MMVC_Trainer/create_dataset_julius.py
Executable file
@ -0,0 +1,87 @@
|
||||
import glob
|
||||
import sys
|
||||
|
||||
def read_lab(lab_f):
|
||||
with open(lab_f, 'r') as f:
|
||||
kw_list = f.read().split("\n")
|
||||
|
||||
out_phono = []
|
||||
for i in range(len(kw_list)-1):
|
||||
out_phono.append(kw_list[i].split()[2])
|
||||
out_phono.append("-")
|
||||
|
||||
if out_phono[0] == 'silB' and out_phono[-2] == 'silE':
|
||||
out_phono[0] = 'sil'
|
||||
out_phono[-2] = 'sil'
|
||||
out_phono = out_phono[0:-1]
|
||||
out_phono_str = "".join(out_phono)
|
||||
return out_phono_str
|
||||
|
||||
else:
|
||||
print("Error!")
|
||||
exit
|
||||
|
||||
def create_dataset(filename):
|
||||
speaker_id = 0
|
||||
textful_dir_list = glob.glob("dataset/textful/*")
|
||||
textless_dir_list = glob.glob("dataset/textless/*")
|
||||
textful_dir_list.sort()
|
||||
textless_dir_list.sort()
|
||||
Correspondence_list = list()
|
||||
output_file_list = list()
|
||||
output_file_list_val = list()
|
||||
output_file_list_textless = list()
|
||||
output_file_list_val_textless = list()
|
||||
for d in textful_dir_list:
|
||||
wav_file_list = glob.glob(d+"/wav/*")
|
||||
lab_file_list = glob.glob(d + "/text/*")
|
||||
wav_file_list.sort()
|
||||
lab_file_list.sort()
|
||||
if len(wav_file_list) == 0:
|
||||
continue
|
||||
counter = 0
|
||||
for lab, wav in zip(lab_file_list, wav_file_list):
|
||||
test = read_lab(lab)
|
||||
print(wav + "|"+ str(speaker_id) + "|"+ test)
|
||||
if counter % 10 != 0:
|
||||
output_file_list.append(wav + "|"+ str(speaker_id) + "|"+ test + "\n")
|
||||
else:
|
||||
output_file_list_val.append(wav + "|"+ str(speaker_id) + "|"+ test + "\n")
|
||||
counter = counter +1
|
||||
Correspondence_list.append(str(speaker_id)+"|"+d + "\n")
|
||||
speaker_id = speaker_id + 1
|
||||
|
||||
for d in textless_dir_list:
|
||||
wav_file_list = glob.glob(d+"/*")
|
||||
wav_file_list.sort()
|
||||
counter = 0
|
||||
for wav in wav_file_list:
|
||||
print(wav + "|"+ str(speaker_id) + "|a")
|
||||
if counter % 10 != 0:
|
||||
output_file_list_textless.append(wav + "|"+ str(speaker_id) + "|a" + "\n")
|
||||
else:
|
||||
output_file_list_val_textless.append(wav + "|"+ str(speaker_id) + "|a" + "\n")
|
||||
counter = counter +1
|
||||
Correspondence_list.append(str(speaker_id)+"|"+d + "\n")
|
||||
speaker_id = speaker_id + 1
|
||||
|
||||
with open('filelists/' + filename + '_textful.txt', 'w', encoding='utf-8', newline='\n') as f:
|
||||
f.writelines(output_file_list)
|
||||
with open('filelists/' + filename + '_textful_val.txt', 'w', encoding='utf-8', newline='\n') as f:
|
||||
f.writelines(output_file_list_val)
|
||||
with open('filelists/' + filename + '_textless.txt', 'w', encoding='utf-8', newline='\n') as f:
|
||||
f.writelines(output_file_list_textless)
|
||||
with open('filelists/' + filename + '_val_textless.txt', 'w', encoding='utf-8', newline='\n') as f:
|
||||
f.writelines(output_file_list_val_textless)
|
||||
with open('filelists/' + filename + '_Correspondence.txt', 'w', encoding='utf-8', newline='\n') as f:
|
||||
f.writelines(Correspondence_list)
|
||||
return speaker_id -1
|
||||
|
||||
def main(argv):
|
||||
filename = str(sys.argv[1])
|
||||
print(filename)
|
||||
n_spk = create_dataset(filename)
|
||||
return filename, n_spk
|
||||
|
||||
if __name__ == '__main__':
|
||||
sys.exit(main(sys.argv))
|
492
demo/MMVC_Trainer/data_utils.py
Executable file
492
demo/MMVC_Trainer/data_utils.py
Executable file
@ -0,0 +1,492 @@
|
||||
import time
|
||||
import os
|
||||
import random
|
||||
import numpy as np
|
||||
import torch
|
||||
import torch.utils.data
|
||||
import tqdm
|
||||
|
||||
import commons
|
||||
from mel_processing import spectrogram_torch
|
||||
from utils import load_wav_to_torch, load_filepaths_and_text
|
||||
from text import text_to_sequence, cleaned_text_to_sequence
|
||||
|
||||
#add
|
||||
from retry import retry
|
||||
import random
|
||||
import torchaudio
|
||||
|
||||
|
||||
class TextAudioLoader(torch.utils.data.Dataset):
|
||||
"""
|
||||
1) loads audio, text pairs
|
||||
2) normalizes text and converts them to sequences of integers
|
||||
3) computes spectrograms from audio files.
|
||||
"""
|
||||
def __init__(self, audiopaths_and_text, hparams, use_test = True):
|
||||
self.audiopaths_and_text = load_filepaths_and_text(audiopaths_and_text)
|
||||
self.text_cleaners = hparams.text_cleaners
|
||||
self.max_wav_value = hparams.max_wav_value
|
||||
self.sampling_rate = hparams.sampling_rate
|
||||
self.filter_length = hparams.filter_length
|
||||
self.hop_length = hparams.hop_length
|
||||
self.win_length = hparams.win_length
|
||||
self.sampling_rate = hparams.sampling_rate
|
||||
self.use_test = use_test
|
||||
|
||||
self.cleaned_text = getattr(hparams, "cleaned_text", False)
|
||||
|
||||
self.add_blank = hparams.add_blank
|
||||
self.min_text_len = getattr(hparams, "min_text_len", 1)
|
||||
self.max_text_len = getattr(hparams, "max_text_len", 190)
|
||||
|
||||
random.seed(1234)
|
||||
random.shuffle(self.audiopaths_and_text)
|
||||
self._filter()
|
||||
|
||||
|
||||
def _filter(self):
|
||||
"""
|
||||
Filter text & store spec lengths
|
||||
"""
|
||||
# Store spectrogram lengths for Bucketing
|
||||
# wav_length ~= file_size / (wav_channels * Bytes per dim) = file_size / (1 * 2)
|
||||
# spec_length = wav_length // hop_length
|
||||
|
||||
audiopaths_and_text_new = []
|
||||
lengths = []
|
||||
for audiopath, text in self.audiopaths_and_text:
|
||||
if self.min_text_len <= len(text) and len(text) <= self.max_text_len:
|
||||
audiopaths_and_text_new.append([audiopath, text])
|
||||
lengths.append(os.path.getsize(audiopath) // (2 * self.hop_length))
|
||||
self.audiopaths_and_text = audiopaths_and_text_new
|
||||
self.lengths = lengths
|
||||
|
||||
def get_audio_text_pair(self, audiopath_and_text):
|
||||
# separate filename and text
|
||||
audiopath, text = audiopath_and_text[0], audiopath_and_text[1]
|
||||
text = self.get_text(text)
|
||||
if self.use_test != True:
|
||||
text = torch.as_tensor("a")
|
||||
spec, wav = self.get_audio(audiopath)
|
||||
return (text, spec, wav)
|
||||
|
||||
def get_audio(self, filename):
|
||||
audio, sampling_rate = load_wav_to_torch(filename)
|
||||
if sampling_rate != self.sampling_rate:
|
||||
raise ValueError("{} {} SR doesn't match target {} SR".format(
|
||||
sampling_rate, self.sampling_rate))
|
||||
audio_norm = audio / self.max_wav_value
|
||||
audio_norm = audio_norm.unsqueeze(0)
|
||||
spec_filename = filename.replace(".wav", ".spec.pt")
|
||||
if os.path.exists(spec_filename):
|
||||
spec = torch.load(spec_filename)
|
||||
else:
|
||||
spec = spectrogram_torch(audio_norm, self.filter_length,
|
||||
self.sampling_rate, self.hop_length, self.win_length,
|
||||
center=False)
|
||||
spec = torch.squeeze(spec, 0)
|
||||
torch.save(spec, spec_filename)
|
||||
return spec, audio_norm
|
||||
|
||||
def get_text(self, text):
|
||||
if self.cleaned_text:
|
||||
text_norm = cleaned_text_to_sequence(text)
|
||||
else:
|
||||
text_norm = text_to_sequence(text, self.text_cleaners)
|
||||
if self.add_blank:
|
||||
text_norm = commons.intersperse(text_norm, 0)
|
||||
text_norm = torch.LongTensor(text_norm)
|
||||
return text_norm
|
||||
|
||||
def __getitem__(self, index):
|
||||
return self.get_audio_text_pair(self.audiopaths_and_text[index])
|
||||
|
||||
def __len__(self):
|
||||
return len(self.audiopaths_and_text)
|
||||
|
||||
|
||||
class TextAudioCollate():
|
||||
""" Zero-pads model inputs and targets
|
||||
"""
|
||||
def __init__(self, return_ids=False):
|
||||
self.return_ids = return_ids
|
||||
|
||||
def __call__(self, batch):
|
||||
"""Collate's training batch from normalized text and aduio
|
||||
PARAMS
|
||||
------
|
||||
batch: [text_normalized, spec_normalized, wav_normalized]
|
||||
"""
|
||||
# Right zero-pad all one-hot text sequences to max input length
|
||||
_, ids_sorted_decreasing = torch.sort(
|
||||
torch.LongTensor([x[1].size(1) for x in batch]),
|
||||
dim=0, descending=True)
|
||||
|
||||
max_text_len = max([len(x[0]) for x in batch])
|
||||
max_spec_len = max([x[1].size(1) for x in batch])
|
||||
max_wav_len = max([x[2].size(1) for x in batch])
|
||||
|
||||
text_lengths = torch.LongTensor(len(batch))
|
||||
spec_lengths = torch.LongTensor(len(batch))
|
||||
wav_lengths = torch.LongTensor(len(batch))
|
||||
|
||||
text_padded = torch.LongTensor(len(batch), max_text_len)
|
||||
spec_padded = torch.FloatTensor(len(batch), batch[0][1].size(0), max_spec_len)
|
||||
wav_padded = torch.FloatTensor(len(batch), 1, max_wav_len)
|
||||
text_padded.zero_()
|
||||
spec_padded.zero_()
|
||||
wav_padded.zero_()
|
||||
for i in range(len(ids_sorted_decreasing)):
|
||||
row = batch[ids_sorted_decreasing[i]]
|
||||
|
||||
text = row[0]
|
||||
text_padded[i, :text.size(0)] = text
|
||||
text_lengths[i] = text.size(0)
|
||||
|
||||
spec = row[1]
|
||||
spec_padded[i, :, :spec.size(1)] = spec
|
||||
spec_lengths[i] = spec.size(1)
|
||||
|
||||
wav = row[2]
|
||||
wav_padded[i, :, :wav.size(1)] = wav
|
||||
wav_lengths[i] = wav.size(1)
|
||||
|
||||
if self.return_ids:
|
||||
return text_padded, text_lengths, spec_padded, spec_lengths, wav_padded, wav_lengths, ids_sorted_decreasing
|
||||
return text_padded, text_lengths, spec_padded, spec_lengths, wav_padded, wav_lengths
|
||||
|
||||
|
||||
"""Multi speaker version"""
|
||||
class TextAudioSpeakerLoader(torch.utils.data.Dataset):
|
||||
"""
|
||||
1) loads audio, speaker_id, text pairs
|
||||
2) normalizes text and converts them to sequences of integers
|
||||
3) computes spectrograms from audio files.
|
||||
"""
|
||||
def __init__(self, audiopaths_sid_text, hparams, no_text=False, augmentation=False, augmentation_params=None, no_use_textfile = False):
|
||||
if no_use_textfile:
|
||||
self.audiopaths_sid_text = list()
|
||||
else:
|
||||
self.audiopaths_sid_text = load_filepaths_and_text(audiopaths_sid_text)
|
||||
self.text_cleaners = hparams.text_cleaners
|
||||
self.max_wav_value = hparams.max_wav_value
|
||||
self.sampling_rate = hparams.sampling_rate
|
||||
self.filter_length = hparams.filter_length
|
||||
self.hop_length = hparams.hop_length
|
||||
self.win_length = hparams.win_length
|
||||
self.sampling_rate = hparams.sampling_rate
|
||||
self.no_text = no_text
|
||||
self.augmentation = augmentation
|
||||
if augmentation :
|
||||
self.gain_p = augmentation_params.gain_p
|
||||
self.min_gain_in_db = augmentation_params.min_gain_in_db
|
||||
self.max_gain_in_db = augmentation_params.max_gain_in_db
|
||||
self.time_stretch_p = augmentation_params.time_stretch_p
|
||||
self.min_rate = augmentation_params.min_rate
|
||||
self.max_rate = augmentation_params.max_rate
|
||||
self.pitch_shift_p = augmentation_params.pitch_shift_p
|
||||
self.min_semitones = augmentation_params.min_semitones
|
||||
self.max_semitones = augmentation_params.max_semitones
|
||||
self.add_gaussian_noise_p = augmentation_params.add_gaussian_noise_p
|
||||
self.min_amplitude = augmentation_params.min_amplitude
|
||||
self.max_amplitude = augmentation_params.max_amplitude
|
||||
self.frequency_mask_p = augmentation_params.frequency_mask_p
|
||||
|
||||
self.cleaned_text = getattr(hparams, "cleaned_text", False)
|
||||
|
||||
self.add_blank = hparams.add_blank
|
||||
self.min_text_len = getattr(hparams, "min_text_len", 1)
|
||||
self.max_text_len = getattr(hparams, "max_text_len", 1000)
|
||||
|
||||
random.seed(1234)
|
||||
random.shuffle(self.audiopaths_sid_text)
|
||||
self._filter()
|
||||
|
||||
@retry(tries=30, delay=10)
|
||||
def _filter(self):
|
||||
"""
|
||||
Filter text & store spec lengths
|
||||
"""
|
||||
# Store spectrogram lengths for Bucketing
|
||||
# wav_length ~= file_size / (wav_channels * Bytes per dim) = file_size / (1 * 2)
|
||||
# spec_length = wav_length // hop_length
|
||||
|
||||
audiopaths_sid_text_new = []
|
||||
lengths = []
|
||||
|
||||
for audiopath, sid, text in tqdm.tqdm(self.audiopaths_sid_text):
|
||||
if self.min_text_len <= len(text) and len(text) <= self.max_text_len:
|
||||
audiopaths_sid_text_new.append([audiopath, sid, text])
|
||||
lengths.append(os.path.getsize(audiopath) // (2 * self.hop_length))
|
||||
self.audiopaths_sid_text = audiopaths_sid_text_new
|
||||
self.lengths = lengths
|
||||
|
||||
def get_audio_text_speaker_pair(self, audiopath_sid_text):
|
||||
# separate filename, speaker_id and text
|
||||
audiopath, sid, text = audiopath_sid_text[0], audiopath_sid_text[1], audiopath_sid_text[2]
|
||||
text = self.get_text(text)
|
||||
if self.no_text:
|
||||
text = self.get_text("a")
|
||||
spec, wav = self.get_audio(audiopath)
|
||||
sid = self.get_sid(sid)
|
||||
return (text, spec, wav, sid)
|
||||
|
||||
@retry(exceptions=(PermissionError), tries=100, delay=10)
|
||||
def get_audio(self, filename):
|
||||
# 音声データは±1.0内に正規化したtorchベクトルでunsqueeze(0)で外側1次元くるんだものを扱う
|
||||
audio, sampling_rate = load_wav_to_torch(filename)
|
||||
try:
|
||||
if sampling_rate != self.sampling_rate:
|
||||
raise ValueError("[Error] Exception: source {} SR doesn't match target {} SR".format(
|
||||
sampling_rate, self.sampling_rate))
|
||||
except ValueError as e:
|
||||
print(e)
|
||||
exit()
|
||||
audio_norm = self.get_normalized_audio(audio, self.max_wav_value)
|
||||
|
||||
if self.augmentation:
|
||||
audio_augmented = self.add_augmentation(audio_norm, sampling_rate)
|
||||
audio_noised = self.add_noise(audio_augmented, sampling_rate)
|
||||
# ノーマライズ後のaugmentationとnoise付加で範囲外になったところを削る
|
||||
audio_augmented = torch.clamp(audio_augmented, -1, 1)
|
||||
audio_noised = torch.clamp(audio_noised, -1, 1)
|
||||
# audio(音声波形)は教師信号となるのでノイズは含まずaugmentationのみしたものを使用
|
||||
audio_norm = audio_augmented
|
||||
# spec(スペクトログラム)は入力信号となるのでaugmentationしてさらにノイズを付加したものを使用
|
||||
spec = spectrogram_torch(audio_noised, self.filter_length,
|
||||
self.sampling_rate, self.hop_length, self.win_length,
|
||||
center=False)
|
||||
spec_noised = self.add_spectrogram_noise(spec)
|
||||
spec = torch.squeeze(spec_noised, 0)
|
||||
else:
|
||||
spec = spectrogram_torch(audio_norm, self.filter_length,
|
||||
self.sampling_rate, self.hop_length, self.win_length,
|
||||
center=False)
|
||||
spec = torch.squeeze(spec, 0)
|
||||
return spec, audio_norm
|
||||
|
||||
def add_augmentation(self, audio, sampling_rate):
|
||||
gain_in_db = 0.0
|
||||
if random.random() <= self.gain_p:
|
||||
gain_in_db = random.uniform(self.min_gain_in_db, self.max_gain_in_db)
|
||||
time_stretch_rate = 1.0
|
||||
if random.random() <= self.time_stretch_p:
|
||||
time_stretch_rate = random.uniform(self.min_rate, self.max_rate)
|
||||
pitch_shift_semitones = 0
|
||||
if random.random() <= self.pitch_shift_p:
|
||||
pitch_shift_semitones = random.uniform(self.min_semitones, self.max_semitones) * 100 # 1/100 semitone 単位指定のため
|
||||
augmentation_effects = [
|
||||
["gain", f"{gain_in_db}"],
|
||||
["tempo", f"{time_stretch_rate}"],
|
||||
["pitch", f"{pitch_shift_semitones}"],
|
||||
["rate", f"{sampling_rate}"]
|
||||
]
|
||||
audio_augmented, _ = torchaudio.sox_effects.apply_effects_tensor(audio, sampling_rate, augmentation_effects)
|
||||
return audio_augmented
|
||||
|
||||
def add_noise(self, audio, sampling_rate):
|
||||
# AddGaussianNoise
|
||||
audio = self.add_gaussian_noise(audio)
|
||||
return audio
|
||||
|
||||
def add_gaussian_noise(self, audio):
|
||||
assert self.min_amplitude >= 0.0
|
||||
assert self.max_amplitude >= 0.0
|
||||
assert self.max_amplitude >= self.min_amplitude
|
||||
if random.random() > self.add_gaussian_noise_p:
|
||||
return audio
|
||||
amplitude = random.uniform(self.min_amplitude, self.max_amplitude)
|
||||
noise = torch.randn(audio.size())
|
||||
noised_audio = audio + amplitude * noise
|
||||
return noised_audio
|
||||
|
||||
def add_spectrogram_noise(self, spec):
|
||||
# FrequencyMask
|
||||
masking = torchaudio.transforms.FrequencyMasking(freq_mask_param=80)
|
||||
masked = masking(spec)
|
||||
return masked
|
||||
|
||||
def get_normalized_audio(self, audio, max_wav_value):
|
||||
audio_norm = audio / max_wav_value
|
||||
audio_norm = audio_norm.unsqueeze(0)
|
||||
return audio_norm
|
||||
|
||||
def get_text(self, text):
|
||||
if self.cleaned_text:
|
||||
text_norm = cleaned_text_to_sequence(text)
|
||||
else:
|
||||
text_norm = text_to_sequence(text, self.text_cleaners)
|
||||
if self.add_blank:
|
||||
text_norm = commons.intersperse(text_norm, 0)
|
||||
text_norm = torch.LongTensor(text_norm)
|
||||
return text_norm
|
||||
|
||||
def get_sid(self, sid):
|
||||
sid = torch.LongTensor([int(sid)])
|
||||
return sid
|
||||
|
||||
def __getitem__(self, index):
|
||||
return self.get_audio_text_speaker_pair(self.audiopaths_sid_text[index])
|
||||
|
||||
def __len__(self):
|
||||
return len(self.audiopaths_sid_text)
|
||||
|
||||
|
||||
class TextAudioSpeakerCollate():
|
||||
""" Zero-pads model inputs and targets
|
||||
"""
|
||||
def __init__(self, return_ids=False, no_text = False):
|
||||
self.return_ids = return_ids
|
||||
self.no_text = no_text
|
||||
|
||||
def __call__(self, batch):
|
||||
"""Collate's training batch from normalized text, audio and speaker identities
|
||||
PARAMS
|
||||
------
|
||||
batch: [text_normalized, spec_normalized, wav_normalized, sid]
|
||||
"""
|
||||
# Right zero-pad all one-hot text sequences to max input length
|
||||
_, ids_sorted_decreasing = torch.sort(
|
||||
torch.LongTensor([x[1].size(1) for x in batch]),
|
||||
dim=0, descending=True)
|
||||
|
||||
max_text_len = max([len(x[0]) for x in batch])
|
||||
max_spec_len = max([x[1].size(1) for x in batch])
|
||||
max_wav_len = max([x[2].size(1) for x in batch])
|
||||
|
||||
text_lengths = torch.LongTensor(len(batch))
|
||||
spec_lengths = torch.LongTensor(len(batch))
|
||||
wav_lengths = torch.LongTensor(len(batch))
|
||||
sid = torch.LongTensor(len(batch))
|
||||
|
||||
text_padded = torch.LongTensor(len(batch), max_text_len)
|
||||
spec_padded = torch.FloatTensor(len(batch), batch[0][1].size(0), max_spec_len)
|
||||
wav_padded = torch.FloatTensor(len(batch), 1, max_wav_len)
|
||||
text_padded.zero_()
|
||||
spec_padded.zero_()
|
||||
wav_padded.zero_()
|
||||
for i in range(len(ids_sorted_decreasing)):
|
||||
row = batch[ids_sorted_decreasing[i]]
|
||||
|
||||
text = row[0]
|
||||
text_padded[i, :text.size(0)] = text
|
||||
text_lengths[i] = text.size(0)
|
||||
|
||||
spec = row[1]
|
||||
spec_padded[i, :, :spec.size(1)] = spec
|
||||
spec_lengths[i] = spec.size(1)
|
||||
|
||||
wav = row[2]
|
||||
wav_padded[i, :, :wav.size(1)] = wav
|
||||
wav_lengths[i] = wav.size(1)
|
||||
|
||||
sid[i] = row[3]
|
||||
|
||||
if self.return_ids:
|
||||
return text_padded, text_lengths, spec_padded, spec_lengths, wav_padded, wav_lengths, sid, ids_sorted_decreasing
|
||||
return text_padded, text_lengths, spec_padded, spec_lengths, wav_padded, wav_lengths, sid
|
||||
|
||||
|
||||
class DistributedBucketSampler(torch.utils.data.distributed.DistributedSampler):
|
||||
"""
|
||||
Maintain similar input lengths in a batch.
|
||||
Length groups are specified by boundaries.
|
||||
Ex) boundaries = [b1, b2, b3] -> any batch is included either {x | b1 < length(x) <=b2} or {x | b2 < length(x) <= b3}.
|
||||
|
||||
It removes samples which are not included in the boundaries.
|
||||
Ex) boundaries = [b1, b2, b3] -> any x s.t. length(x) <= b1 or length(x) > b3 are discarded.
|
||||
"""
|
||||
def __init__(self, dataset, batch_size, boundaries, num_replicas=None, rank=None, shuffle=True):
|
||||
super().__init__(dataset, num_replicas=num_replicas, rank=rank, shuffle=shuffle)
|
||||
self.lengths = dataset.lengths
|
||||
self.batch_size = batch_size
|
||||
self.boundaries = boundaries
|
||||
|
||||
self.buckets, self.num_samples_per_bucket = self._create_buckets()
|
||||
self.total_size = sum(self.num_samples_per_bucket)
|
||||
self.num_samples = self.total_size // self.num_replicas
|
||||
|
||||
def _create_buckets(self):
|
||||
buckets = [[] for _ in range(len(self.boundaries) - 1)]
|
||||
for i in range(len(self.lengths)):
|
||||
length = self.lengths[i]
|
||||
idx_bucket = self._bisect(length)
|
||||
if idx_bucket != -1:
|
||||
buckets[idx_bucket].append(i)
|
||||
|
||||
for i in range(len(buckets) - 1, 0, -1):
|
||||
if len(buckets[i]) == 0:
|
||||
buckets.pop(i)
|
||||
self.boundaries.pop(i+1)
|
||||
|
||||
num_samples_per_bucket = []
|
||||
for i in range(len(buckets)):
|
||||
len_bucket = len(buckets[i])
|
||||
total_batch_size = self.num_replicas * self.batch_size
|
||||
rem = (total_batch_size - (len_bucket % total_batch_size)) % total_batch_size
|
||||
num_samples_per_bucket.append(len_bucket + rem)
|
||||
return buckets, num_samples_per_bucket
|
||||
|
||||
def __iter__(self):
|
||||
# deterministically shuffle based on epoch
|
||||
g = torch.Generator()
|
||||
g.manual_seed(self.epoch)
|
||||
|
||||
indices = []
|
||||
if self.shuffle:
|
||||
for bucket in self.buckets:
|
||||
indices.append(torch.randperm(len(bucket), generator=g).tolist())
|
||||
else:
|
||||
for bucket in self.buckets:
|
||||
indices.append(list(range(len(bucket))))
|
||||
|
||||
batches = []
|
||||
for i in range(len(self.buckets)):
|
||||
next_bucket = (i+1) % len(self.buckets)
|
||||
bucket = self.buckets[i]
|
||||
len_bucket = len(bucket)
|
||||
ids_bucket = indices[i]
|
||||
num_samples_bucket = self.num_samples_per_bucket[i]
|
||||
|
||||
if len_bucket == 0:
|
||||
print("[Warn] Exception: length of buckets {} is 0. ID:{} Skip.".format(i,i))
|
||||
continue
|
||||
|
||||
# add extra samples to make it evenly divisible
|
||||
rem = num_samples_bucket - len_bucket
|
||||
ids_bucket = ids_bucket + ids_bucket * (rem // len_bucket) + ids_bucket[:(rem % len_bucket)]
|
||||
|
||||
# subsample
|
||||
ids_bucket = ids_bucket[self.rank::self.num_replicas]
|
||||
|
||||
# batching
|
||||
for j in range(len(ids_bucket) // self.batch_size):
|
||||
batch = [bucket[idx] for idx in ids_bucket[j*self.batch_size:(j+1)*self.batch_size]]
|
||||
batches.append(batch)
|
||||
|
||||
if self.shuffle:
|
||||
batch_ids = torch.randperm(len(batches), generator=g).tolist()
|
||||
batches = [batches[i] for i in batch_ids]
|
||||
self.batches = batches
|
||||
|
||||
assert len(self.batches) * self.batch_size == self.num_samples
|
||||
return iter(self.batches)
|
||||
|
||||
def _bisect(self, x, lo=0, hi=None):
|
||||
if hi is None:
|
||||
hi = len(self.boundaries) - 1
|
||||
|
||||
if hi > lo:
|
||||
mid = (hi + lo) // 2
|
||||
if self.boundaries[mid] < x and x <= self.boundaries[mid+1]:
|
||||
return mid
|
||||
elif x <= self.boundaries[mid]:
|
||||
return self._bisect(x, lo, mid)
|
||||
else:
|
||||
return self._bisect(x, mid + 1, hi)
|
||||
else:
|
||||
return -1
|
||||
|
||||
def __len__(self):
|
||||
return self.num_samples // self.batch_size
|
7
demo/MMVC_Trainer/dataset/multi_speaker_correspondence.txt
Executable file
7
demo/MMVC_Trainer/dataset/multi_speaker_correspondence.txt
Executable file
@ -0,0 +1,7 @@
|
||||
00_myvoice|107
|
||||
01_target|108
|
||||
02_target|109
|
||||
03_target|0
|
||||
04_target|1
|
||||
05_target|2
|
||||
1205_zundamon|100
|
2
demo/MMVC_Trainer/dataset/textful/00_myvoice/text/.gitignore
vendored
Executable file
2
demo/MMVC_Trainer/dataset/textful/00_myvoice/text/.gitignore
vendored
Executable file
@ -0,0 +1,2 @@
|
||||
*
|
||||
!.gitignore
|
2
demo/MMVC_Trainer/dataset/textful/00_myvoice/wav/.gitignore
vendored
Executable file
2
demo/MMVC_Trainer/dataset/textful/00_myvoice/wav/.gitignore
vendored
Executable file
@ -0,0 +1,2 @@
|
||||
*
|
||||
!.gitignore
|
2
demo/MMVC_Trainer/dataset/textful/01_target/text/.gitignore
vendored
Executable file
2
demo/MMVC_Trainer/dataset/textful/01_target/text/.gitignore
vendored
Executable file
@ -0,0 +1,2 @@
|
||||
*
|
||||
!.gitignore
|
2
demo/MMVC_Trainer/dataset/textful/01_target/wav/.gitignore
vendored
Executable file
2
demo/MMVC_Trainer/dataset/textful/01_target/wav/.gitignore
vendored
Executable file
@ -0,0 +1,2 @@
|
||||
*
|
||||
!.gitignore
|
@ -0,0 +1 @@
|
||||
オンナノコガキッキッウレシソー。
|
@ -0,0 +1 @@
|
||||
ツァツォニリョコーシタ。
|
@ -0,0 +1 @@
|
||||
ミンシュウガテュルリーキュウデンニシンニュウシタ。
|
@ -0,0 +1 @@
|
||||
ハイチキョーワコクデトゥーサンルーヴェルテュールガショーリヲオサメラレタノワ、ジッサイオーネツビョーノオカゲダッタ。
|
@ -0,0 +1 @@
|
||||
レジャンドルワミンシュウヲテュルリーキュウデンニマネータ。
|
@ -0,0 +1 @@
|
||||
ジョゲンワデキナイトデュパンワイッタ。
|
@ -0,0 +1 @@
|
||||
フランスジンシェフトニホンジンシェフワゼンゼンチガウ。
|
@ -0,0 +1 @@
|
||||
チュウゴクノガイコーダンニアタッシェトシテハケンサレタ。
|
@ -0,0 +1 @@
|
||||
ファシズムセーリョクトノソーリョクセンニノゾム。
|
@ -0,0 +1 @@
|
||||
カグショーニンノフィシェルワ、ニグルマトコウマヲカシテクレタ。
|
@ -0,0 +1 @@
|
||||
ローカルロセンニワファンモオオイ。
|
@ -0,0 +1 @@
|
||||
フェイントデアイテヲカワシテカラシュートデフィニッシュシタ。
|
@ -0,0 +1 @@
|
||||
センハッピャクナナジュウナナ、プフェファーニヨリシントーゲンショーガハッケンサレタ。
|
@ -0,0 +1 @@
|
||||
ユレルフェリーニノルノワワタシニトッテクギョーデス。
|
@ -0,0 +1 @@
|
||||
ホルロアラティタルッフォトユウトクベツナオリョーリモデマシタ。
|
@ -0,0 +1 @@
|
||||
フエノオトガナルトウサギノキッドガサッソクピョントハネタ。
|
@ -0,0 +1 @@
|
||||
アノリョキャクワウワサノキャフェニイクヨーデス。
|
@ -0,0 +1 @@
|
||||
モクヒョーワイットーショーデス。
|
@ -0,0 +1 @@
|
||||
ウサギノキッドワキブンヨクピョン、マタピョントトビツヅケタ。
|
@ -0,0 +1 @@
|
||||
アフタヌーンティーヲタノシミマショー。
|
@ -0,0 +1 @@
|
||||
カノジョワティピカルナフェミニストデス。
|
@ -0,0 +1 @@
|
||||
ジョシュタチトミッツィワサガシテイルショルイヲミツケラレナカッタ。
|
@ -0,0 +1 @@
|
||||
フィレンツェ、パドヴァ、ヴェネツィアワドレモイタリアノトシデス。
|
@ -0,0 +1 @@
|
||||
ガクフニツギノヨーニカイテアルノガ、エーフェリチェデス。
|
@ -0,0 +1 @@
|
||||
ショペンハウエルトニーチェノテツガクショヲホンダナカラトリダシタ。
|
@ -0,0 +1 @@
|
||||
サッソクメシツカイゼンインニシラセマショー。
|
@ -0,0 +1 @@
|
||||
オモイワタイレヲヌイデ、アワセニキガエル。
|
@ -0,0 +1 @@
|
||||
ボストンデ、トアルチョプスイヤエハイッテユウハンヲクッタ。
|
@ -0,0 +1 @@
|
||||
ロクスッポキュウケーヲトラズハタライタ。
|
@ -0,0 +1 @@
|
||||
カツテヒトリデコクフニシンニュウシタ。
|
@ -0,0 +1 @@
|
||||
ダガ、キョーオマエガココエゴジュライニナッタノワ、ドンナゴヨーナノカナ?
|
@ -0,0 +1 @@
|
||||
サブフランチャイザーヲフヤシテメザセヒャクテンポ。
|
@ -0,0 +1 @@
|
||||
シコクデオヘンロヲアンギャシヨー。
|
@ -0,0 +1 @@
|
||||
イツモノトオリギャンギャンナキダシマシタ。
|
@ -0,0 +1 @@
|
||||
センセーワ、タッタママニュースヲミテイマシタ。
|
@ -0,0 +1 @@
|
||||
ワタシワギョットメヲミヒライタ。
|
@ -0,0 +1 @@
|
||||
トモダチエニューイヤーカードヲオクロー。
|
@ -0,0 +1 @@
|
||||
カセーフワヤスミニオシャレナアウターウェアニミヲツツミヒトリデヤタイヲタノシミマシタ。
|
@ -0,0 +1 @@
|
||||
ウォッカノオトモニワシオヅケノキュウリガアイマス。
|
@ -0,0 +1 @@
|
||||
ヤマノムコーノミュンヒェンノヒトタチガコーゲキヲシカケタ。
|
@ -0,0 +1 @@
|
||||
ボスニアコッキョーカラノコーゲキニヨリ、ジュウイチガツニヴァリェヴォガセンリョーサレタ。
|
@ -0,0 +1 @@
|
||||
シルヴィウスワデュボアトヨバレテイタフランスノユグノーノイエニウマレタ。
|
@ -0,0 +1 @@
|
||||
ソノホカニワタシニデキルコトワナカッタノデス、ユリエワナミダゴエニナッタ。
|
@ -0,0 +1 @@
|
||||
ガルハカセヒャクタイチカク。
|
@ -0,0 +1 @@
|
||||
ニホンセーフカラノヒャクチョーエンヲコエルヨサンヨーキュウ。
|
@ -0,0 +1 @@
|
||||
シャキョーノウツクシサニワタシワギョーテンシテシマッタ。
|
@ -0,0 +1 @@
|
||||
ソプラノカシュポリランダチョワカゲキアイーダノトクベツメーカシュトヒョーバンデス。
|
@ -0,0 +1 @@
|
||||
アナタニワサイショヒャクポンドワタシマス。
|
@ -0,0 +1 @@
|
||||
シャチョーカラノシジデス。
|
@ -0,0 +1 @@
|
||||
ドーモキマグレトユウモノワタショーメフィスティックナモノデアルラシイ。
|
@ -0,0 +1 @@
|
||||
カエルガピョコピョコトビマワッテイマス。
|
@ -0,0 +1 @@
|
||||
マキョーニアシヲフミイレル。
|
@ -0,0 +1 @@
|
||||
ヴァンダーヴォットタイムチュウワ、イワユルパーティーノヨーデハレヤカデス。
|
@ -0,0 +1 @@
|
||||
スピリッツトワジョーリュウシュノコトデス。
|
@ -0,0 +1 @@
|
||||
ヌルシアノベネディクトゥスワアポロンシンデンヲコワシ、ベネディクトカイノシュウドーインヲタテタ。
|
@ -0,0 +1 @@
|
||||
チョードソノトキ、テストゥパーゴガコップヲモッテタチアガリマシタ。
|
@ -0,0 +1 @@
|
||||
パフィーノグッズガノコラズヘヤニオチツイタ。
|
@ -0,0 +1 @@
|
||||
エピファーノフワサイフヲナクシタ。
|
@ -0,0 +1 @@
|
||||
ポピュラーナソフトヲツカイセキュアナジョータイヲフッキュウスル。
|
@ -0,0 +1 @@
|
||||
チョコノザイコアッタカナ?
|
@ -0,0 +1 @@
|
||||
オメエ、コノシコミニャア、ドノクレエジカンカカルカシッテッカ。
|
@ -0,0 +1 @@
|
||||
ソレニ、コノホーガカラダノタメニャズットイインダカラネ。
|
@ -0,0 +1 @@
|
||||
ナツヤスミニ、トラアヴェミュンエリョコーシタ。
|
@ -0,0 +1 @@
|
||||
ココデイッショニウェイクフィールドノオバヲマッタ。
|
@ -0,0 +1 @@
|
||||
ヤッツニナルウォルタートイッショニデタキョーダイガイタガ、ウォルターダケハッケンサレタ。
|
@ -0,0 +1 @@
|
||||
サイショノジョブワウォーリアガイイトオモイマス。
|
@ -0,0 +1 @@
|
||||
オヨソロッピャクメートルサキヲウセツデス。
|
@ -0,0 +1 @@
|
||||
シンテンオープンノレセプションニタクサンノオキャクサンヲショータイシタ。
|
@ -0,0 +1 @@
|
||||
キャクホンサクシャピエールオービュルナンノキュウジクレマンガ、シュジンノショサイノトヲタイセツソーニヒライタ。
|
@ -0,0 +1 @@
|
||||
ワレワレワ、テンシュキョートカチョーローキョーカイハノモノデ、テンシュキョートガタスウヲシメテイル。
|
@ -0,0 +1 @@
|
||||
ケッキョクノトコロオタガイゴジッポヒャッポダ。
|
@ -0,0 +1 @@
|
||||
トッピョーシモナイハナシダガ、ケッシテウソデワナイ。
|
@ -0,0 +1 @@
|
||||
ネットデケンアンノカイケツヲメザス。
|
@ -0,0 +1 @@
|
||||
キレアジスルドイペティナイフワツカイガッテガヨイ。
|
@ -0,0 +1 @@
|
||||
ユビヲクワエテピュートヒトコエクチブエヲフイタ。
|
@ -0,0 +1 @@
|
||||
クレンペキョートーワブコツナオトコダガ、ジブンノガクモンノヒミツニワフカクヒタッテイタ。
|
@ -0,0 +1 @@
|
||||
シッポフリタテ、ヒゲクイソラス。
|
@ -0,0 +1 @@
|
||||
スベテノエモノヲノゾミドオリニネラウギジュツガアル。
|
@ -0,0 +1 @@
|
||||
タコノグニャグニャシタカンショクガイヤダ。
|
@ -0,0 +1 @@
|
||||
ワタシタチワ、チュウショーテキイシキテキジコヲヒテースルコトデ、ホントーノジコトワシンジンイチニョダトユウコトヲシル。
|
@ -0,0 +1 @@
|
||||
カシマミョージンガクギデサシツラヌイテ、サカナガウゴカナイヨーニシテイル。
|
@ -0,0 +1 @@
|
||||
ワタシワテハジメニ、ドーギョーシャカラハナシヲキクドリョクヲシタ。
|
@ -0,0 +1 @@
|
||||
トテモウレシソーニピョンピョンハネテデテイッタ。
|
Some files were not shown because too many files have changed in this diff Show More
Loading…
Reference in New Issue
Block a user