mirror of
https://github.com/w-okada/voice-changer.git
synced 2025-02-02 16:23:58 +03:00
Merge branch 'master' into tutorial_for_rvc
This commit is contained in:
commit
7db3047999
71
README.md
71
README.md
@ -4,13 +4,18 @@
|
||||
|
||||
## What's New!
|
||||
|
||||
- v.1.5.2.5
|
||||
|
||||
- RVC: Support pitch-less model and rvc-webui model
|
||||
- so-vits-svc40: some bugfix
|
||||
|
||||
- v.1.5.2.4a
|
||||
|
||||
- Fix: Export ONNX
|
||||
|
||||
- v.1.5.2.4
|
||||
|
||||
- RVC で複数も出出る切り替えを実装
|
||||
- RVC で複数モデル切り替えを実装
|
||||
- 通信路を 48KHz に固定
|
||||
|
||||
# VC Client とは
|
||||
@ -21,12 +26,13 @@
|
||||
- [MMVC](https://github.com/isletennos/MMVC_Trainer)
|
||||
- [so-vits-svc](https://github.com/svc-develop-team/so-vits-svc)
|
||||
- [RVC(Retrieval-based-Voice-Conversion)](https://github.com/liujing04/Retrieval-based-Voice-Conversion-WebUI)
|
||||
- [DDSP-SVC](https://github.com/yxlllc/DDSP-SVC)
|
||||
|
||||
2. 本ソフトウェアは、ネットワークを介した利用も可能であり、ゲームなどの高負荷なアプリケーションと同時に使用する場合などに音声変換処理の負荷を外部にオフロードすることができます。
|
||||
|
||||
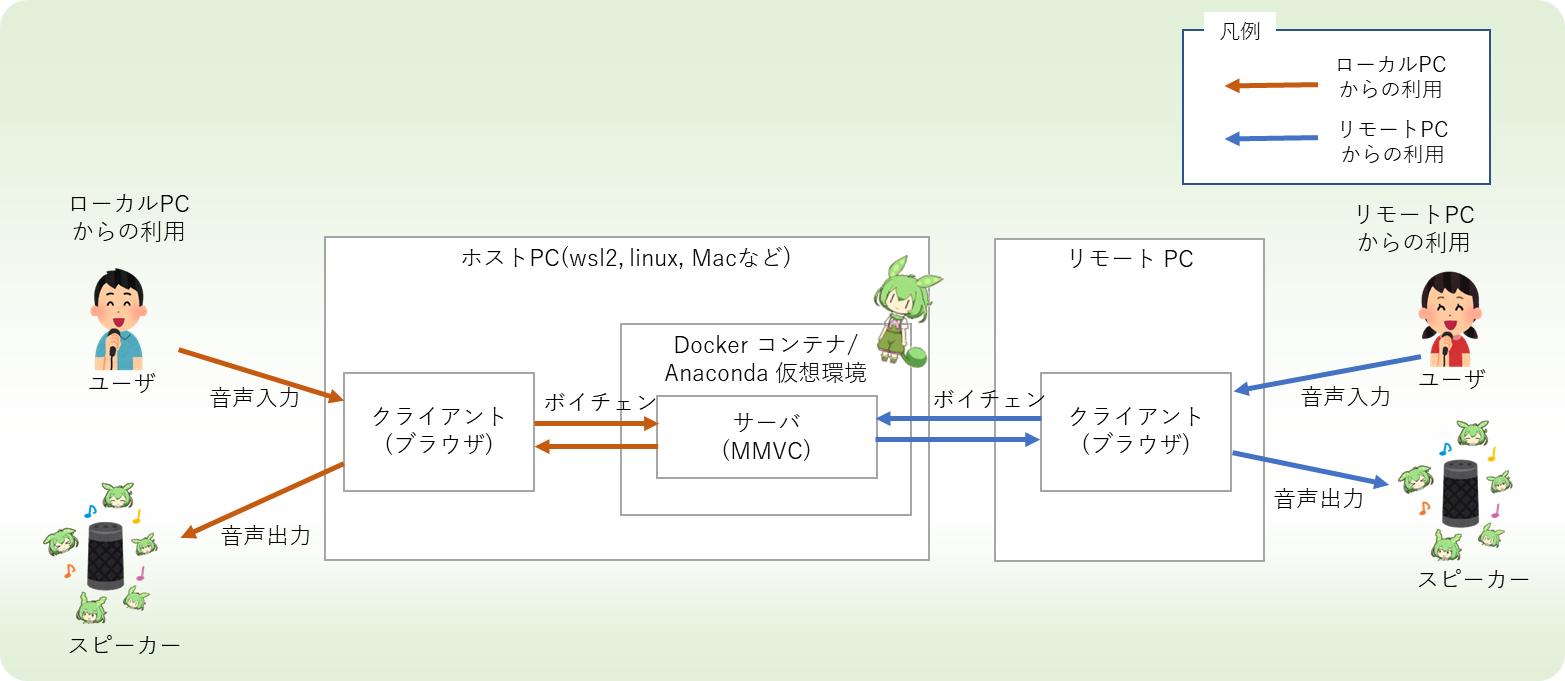
|
||||
|
||||
3. 複数のプラットフォーに対応しています。
|
||||
3. 複数のプラットフォームに対応しています。
|
||||
|
||||
- Windows, Mac(M1), Linux, Google Colab (MMVC のみ)
|
||||
|
||||
@ -59,14 +65,10 @@ Windows 版と Mac 版を提供しています。
|
||||
|
||||
- Windows 版は、ダウンロードした zip ファイルを解凍して、`start_http.bat`を実行してください。
|
||||
|
||||
- Mac 版はダウンロードファイルを解凍したのちに、`startHttp.command`を実行してください。開発元を検証できない旨が示される場合は、再度コントロールキーを押してクリックして実行してください(or 右クリックから実行してください)。(詳細下記 \*1)
|
||||
- Mac 版はダウンロードファイルを解凍したのちに、`startHttp.command`を実行してください。開発元を検証できない旨が示される場合は、再度コントロールキーを押してクリックして実行してください(or 右クリックから実行してください)。
|
||||
|
||||
- リモートから接続する場合は、`.bat`ファイル(win)、`.command`ファイル(mac)の http が https に置き換わっているものを使用してください。
|
||||
|
||||
- Windows 環境で Nvidia の GPU をお持ちの方は多くの場合は `ONNX(cpu,cuda), PyTorch(cpu,cuda)`版で動きます。
|
||||
|
||||
- Windows 環境で Nvidia の GPU をお持ちでない方は多くの場合は `ONNX(cpu,DirectML), PyTorch(cpu) `版で動きます。
|
||||
|
||||
- つくよみちゃん、あみたろ、黄琴まひろ、黄琴海月、の動作には content vec のモデルが必要となります。こちらの[リポジトリ](https://github.com/auspicious3000/contentvec)から、ContentVec_legacy 500 のモデルをダウンロードして、実行する`startHttp.command`や`start_http.bat`と同じフォルダに配置してください。
|
||||
|
||||
- so-vits-svc 4.0/so-vits-svc 4.0v2、RVC(Retrieval-based-Voice-Conversion)の動作には hubert のモデルが必要になります。[このリポジトリ](https://huggingface.co/lj1995/VoiceConversionWebUI/tree/main)から`hubert_base.pt`をダウンロードして、バッチファイルがあるフォルダに格納してください。
|
||||
@ -74,14 +76,16 @@ Windows 版と Mac 版を提供しています。
|
||||
- DDSP-SVC の動作には、hubert-soft と enhancer のモデルが必要です。hubert-soft は[このリンク](https://github.com/bshall/hubert/releases/download/v0.1/hubert-soft-0d54a1f4.pt)からダウンロードして、バッチファイルがあるフォルダに格納してください。enhancer は[このサイト](https://github.com/openvpi/vocoders/releases/tag/nsf-hifigan-v1)から`nsf_hifigan_20221211.zip`ダウンロードして下さい。解凍すると出てくる`nsf_hifigan`というフォルダをバッチファイルがあるフォルダに格納してください。
|
||||
- DDPS-SVC の encoder は hubert-soft のみ対応です。
|
||||
|
||||
| Version | OS | フレームワーク | link | サポート VC | サイズ |
|
||||
| ---------- | --- | --------------------------------- | ------------------------------------------------------------------------------------------ | ----------------------------------------------------------------------------- | ------ |
|
||||
| v.1.5.2.4a | mac | ONNX(cpu,cuda), PyTorch(cpu,cuda) | [normal](https://drive.google.com/uc?id=1fR86gRWalhpi8kQURJmMfWuDvi53V2Ah&export=download) | MMVC v.1.5.x, MMVC v.1.3.x, so-vits-svc 4.0, RVC | 795MB |
|
||||
| | win | ONNX(cpu,cuda), PyTorch(cpu,cuda) | [normal](https://drive.google.com/uc?id=1lttvCgnZengcKkP4f0O2UBAVOcOph4b2&export=download) | MMVC v.1.5.x, MMVC v.1.3.x, so-vits-svc 4.0, so-vits-svc 4.0v2, RVC, DDSP-SVC | 2871MB |
|
||||
| v.1.5.2.4 | mac | ONNX(cpu,cuda), PyTorch(cpu,mps) | [normal](https://drive.google.com/uc?id=1UC0n6Lgyy4ugPznJ-Erd7lskKaOE6--X&export=download) | MMVC v.1.5.x, MMVC v.1.3.x, so-vits-svc 4.0, RVC | 795MB |
|
||||
| | win | ONNX(cpu,cuda), PyTorch(cpu,cuda) | [normal](https://drive.google.com/uc?id=1OmSug85MUR58cnYo_P6Xe_GtNAG7PkKO&export=download) | MMVC v.1.5.x, MMVC v.1.3.x, so-vits-svc 4.0, so-vits-svc 4.0v2, RVC, DDSP-SVC | 2871MB |
|
||||
- RVC で使用する場合の GUI の各項目説明は[こちら](tutorials/tutorial_rvc_ja.md)をご覧ください
|
||||
|
||||
※ [hugging_face](https://huggingface.co/wok000/vcclient/tree/main)でも公開(experimental)
|
||||
- ダウンロードはこちらから。
|
||||
|
||||
| Version | OS | フレームワーク | link | サポート VC | サイズ |
|
||||
| --------- | --- | --------------------------------- | ---------------------------------------------------------------------------------------- | ----------------------------------------------------------------------------- | ------ |
|
||||
| v.1.5.2.6 | mac | ONNX(cpu), PyTorch(cpu,mps) | [通常](https://drive.google.com/uc?id=1NTdtBeKU1bdQKP0_LpbmU3xAjuua1dCT&export=download) | MMVC v.1.5.x, MMVC v.1.3.x, so-vits-svc 4.0, RVC | 784MB |
|
||||
| | win | ONNX(cpu,cuda), PyTorch(cpu,cuda) | [通常](https://drive.google.com/uc?id=1XdoMQoghBOjW__rE2a02zMyQDz8Gi56n&export=download) | MMVC v.1.5.x, MMVC v.1.3.x, so-vits-svc 4.0, so-vits-svc 4.0v2, RVC, DDSP-SVC | 2861MB |
|
||||
|
||||
(\*1) Google Drive からダウンロードできない方は[hugging_face](https://huggingface.co/wok000/vcclient000/tree/main)からダウンロードしてみてください
|
||||
|
||||
- 各キャラクター専用(近々 RVC 版として提供予定)
|
||||
|
||||
@ -99,12 +103,6 @@ Windows 版と Mac 版を提供しています。
|
||||
|
||||
\*2 解凍や起動が遅い場合、ウィルス対策ソフトのチェックが走っている可能性があります。ファイルやフォルダを対象外にして実行してみてください。(自己責任です)
|
||||
|
||||
\*3 本ソフトウェアは開発元の署名しておりません。下記のように警告が出ますが、コントロールキーを押しながらアイコンをクリックすると実行できるようになります。これは Apple のセキュリティポリシーによるものです。実行は自己責任となります。
|
||||
|
||||
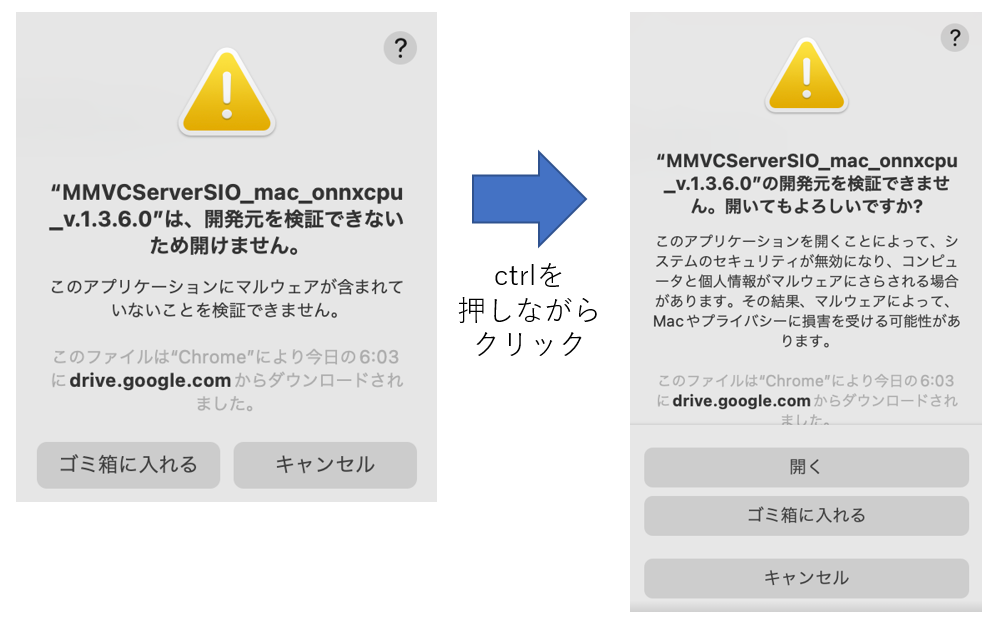
|
||||
|
||||
https://user-images.githubusercontent.com/48346627/212569645-e30b7f4e-079d-4504-8cf8-7816c5f40b00.mp4
|
||||
|
||||
## (3) Docker や Anaconda など環境構築を行った上での利用
|
||||
|
||||
本リポジトリをクローンして利用します。Windows では WSL2 の環境構築が必須になります。また、WSL2 上で Docker もしくは Anaconda などの仮想環境の構築が必要となります。Mac では Anaconda などの Python の仮想環境の構築が必要となります。事前準備が必要となりますが、多くの環境においてこの方法が一番高速で動きます。**<font color="red"> GPU が無くてもそこそこ新しい CPU であれば十分動く可能性があります </font>(下記のリアルタイム性の節を参照)**。
|
||||
@ -117,7 +115,7 @@ Docker での実行は、[Docker を使用する](docker_vcclient/README.md)を
|
||||
|
||||
Anaconda の仮想環境上での実行は、[サーバ開発者向けのページ](README_dev_ja.md)を参考にサーバを起動してください。
|
||||
|
||||
## リアルタイム性
|
||||
# リアルタイム性(MMVC)
|
||||
|
||||
GPU を使用するとほとんどタイムラグなく変換可能です。
|
||||
|
||||
@ -129,6 +127,12 @@ https://twitter.com/DannadoriYellow/status/1613553862773997569?s=20&t=7CLD79h1F3
|
||||
|
||||
古い CPU( i7-4770)だと、1000msec くらいかかってしまう。
|
||||
|
||||
# 開発者の署名について
|
||||
|
||||
本ソフトウェアは開発元の署名しておりません。下記のように警告が出ますが、コントロールキーを押しながらアイコンをクリックすると実行できるようになります。これは Apple のセキュリティポリシーによるものです。実行は自己責任となります。
|
||||
|
||||
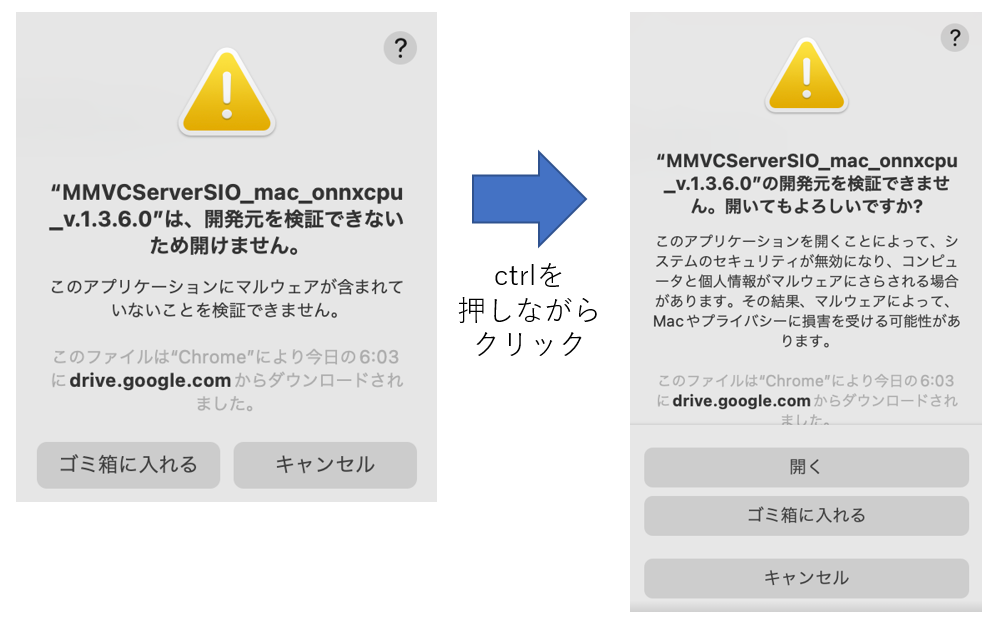
|
||||
|
||||
# Acknowledgments
|
||||
|
||||
- [立ちずんだもん素材](https://seiga.nicovideo.jp/seiga/im10792934)
|
||||
@ -185,28 +189,3 @@ Github Pages 上で実行できるため、ブラウザのみあれば様々な
|
||||
[録音アプリ on Github Pages](https://w-okada.github.io/voice-changer/)
|
||||
|
||||
[解説動画](https://youtu.be/s_GirFEGvaA)
|
||||
|
||||
# 過去バージョン
|
||||
|
||||
| Version | OS | フレームワーク | link | サポート VC | サイズ |
|
||||
| ---------- | --- | --------------------------------- | -------------------------------------------------------------------------------------------- | ----------------------------------------------------------------------------- | ------ |
|
||||
| v.1.5.2.3a | mac | ONNX(cpu,cuda), PyTorch(cpu,mps) | [通常](https://drive.google.com/uc?id=1Ll6_m2ArZrOhwvbqz4lcHNVFFJnZXHRk&export=download) | MMVC v.1.5.x, MMVC v.1.3.x, so-vits-svc 4.0, RVC | 797MB |
|
||||
| | win | ONNX(cpu,cuda), PyTorch(cpu,cuda) | [通常](https://drive.google.com/uc?id=1sZhcrx6sZmmBnfXz_jFEr9Wqez2DGhgj&export=download) | MMVC v.1.5.x, MMVC v.1.3.x, so-vits-svc 4.0, so-vits-svc 4.0v2, RVC, DDSP-SVC | 2871MB |
|
||||
| v.1.5.2.3 | mac | ONNX(cpu,cuda), PyTorch(cpu,mps) | [standard](https://drive.google.com/uc?id=1isX5N9FyC125D5FynJ7NuMnjBCf5dAll&export=download) | MMVC v.1.5.x, MMVC v.1.3.x, so-vits-svc 4.0, RVC | 798MB |
|
||||
| | win | ONNX(cpu,cuda), PyTorch(cpu,cuda) | [standard](https://drive.google.com/uc?id=1UezbE-QTa5jK4mXHRvZz4w07qRnMaPL5&export=download) | MMVC v.1.5.x, MMVC v.1.3.x, so-vits-svc 4.0, so-vits-svc 4.0v2, RVC, DDSP-SVC | 2871MB |
|
||||
| v.1.5.2.2 | mac | ONNX(cpu), PyTorch(cpu) | [通常](https://drive.google.com/uc?id=1dbAiGkPtGWWcQDNL0IHXl4OyTRZR8SIQ&export=download) | MMVC v.1.5.x, MMVC v.1.3.x, so-vits-svc 4.0, so-vits-svc 4.0v2, RVC | 635MB |
|
||||
| | win | ONNX(cpu,cuda), PyTorch(cpu,cuda) | [通常](https://drive.google.com/uc?id=1vIGnrhrU6d_HjvD6JqyWZKT0NruISdj3&export=download) | MMVC v.1.5.x, MMVC v.1.3.x, so-vits-svc 4.0, so-vits-svc 4.0v2, RVC | 2795MB |
|
||||
| v.1.5.2.1 | mac | ONNX(cpu), PyTorch(cpu) | [通常](https://drive.google.com/uc?id=1jaK1ZBdvFpnMmi0PBV8zETw7OY28cKI2&export=download) | MMVC v.1.5.x, MMVC v.1.3.x, so-vits-svc 4.0, so-vits-svc 4.0v2, RVC | 635MB |
|
||||
| | win | ONNX(cpu,cuda), PyTorch(cpu,cuda) | [通常](https://drive.google.com/uc?id=1F7WUSO5P7PT77Zw5xD8pK6KMYFJNV9Ip&export=download) | MMVC v.1.5.x, MMVC v.1.3.x, so-vits-svc 4.0, so-vits-svc 4.0v2, RVC | 2794MB |
|
||||
|
||||
| Version | OS | フレームワーク | link | サポート VC | サイズ |
|
||||
| ----------- | ------------------------------------- | ------------------------------------- | ------------------------------------------------------------------------------------------ | ------------------------------------------------------------------- | ------ |
|
||||
| v.1.5.1.15b | <span style="color: blue;">win</span> | ONNX(cpu,cuda), PyTorch(cpu) | [通常](https://drive.google.com/uc?id=1nb5DxHQJqnYgzWFTBNxCDOx64__uQqyR&export=download) | MMVC v.1.5.x, MMVC v.1.3.x, RVC | 773MB |
|
||||
| | <span style="color: blue;">win</span> | ONNX(cpu,cuda), PyTorch(cpu,cuda) | [通常](https://drive.google.com/uc?id=197U6ip9ypBSyxhIf3oGnkWfBP-M3Gc12&export=download) | MMVC v.1.5.x, MMVC v.1.3.x, so-vits-svc 4.0, so-vits-svc 4.0v2, RVC | 2794MB |
|
||||
| | <span style="color: blue;">win</span> | ONNX(cpu,DirectML), PyTorch(cpu) | [通常](https://drive.google.com/uc?id=18Q9CDBnjgTHwOeklVLWAVMFZI-kk9j3l&export=download) | MMVC v.1.5.x, MMVC v.1.3.x, RVC | 488MB |
|
||||
| | <span style="color: blue;">win</span> | ONNX(cpu,DirectML), PyTorch(cpu,cuda) | [通常](https://drive.google.com/uc?id=1rlGewdhvenv1Yn3WFOLcsWQeuo8ecIQ1&export=download) | MMVC v.1.5.x, MMVC v.1.3.x, so-vits-svc 4.0, so-vits-svc 4.0v2, RVC | 2665MB |
|
||||
| | <span style="color: red;">mac</span> | ONNX(cpu), PyTorch(cpu) | [normal](https://drive.google.com/uc?id=1saAe8vycI4zv0LRbvNmFLfYt0utGRWyZ&export=download) | MMVC v.1.5.x, MMVC v.1.3.x, so-vits-svc 4.0, so-vits-svc 4.0v2, RVC | 615MB |
|
||||
|
||||
| Version | OS | フレームワーク | link | サポート VC | サイズ |
|
||||
| ----------- | ------------------------------------- | --------------------------------- | ---------------------------------------------------------------------------------------- | ------------------------------------------------------------------- | ------ |
|
||||
| v.1.5.1.15a | <span style="color: blue;">win</span> | ONNX(cpu,cuda), PyTorch(cpu,cuda) | [通常](https://drive.google.com/uc?id=1lCo4P3D3QVvrl-0DRh305e34d_YmsI10&export=download) | MMVC v.1.5.x, MMVC v.1.3.x, so-vits-svc 4.0, so-vits-svc 4.0v2, RVC | 2641MB |
|
||||
|
71
README_en.md
71
README_en.md
@ -4,6 +4,11 @@
|
||||
|
||||
## What's New!
|
||||
|
||||
- v.1.5.2.5
|
||||
|
||||
- RVC: Support pitch-less model and rvc-webui model
|
||||
- so-vits-svc40: some bugfix
|
||||
|
||||
- v.1.5.2.4a
|
||||
|
||||
- Fix: Export ONNX
|
||||
@ -15,21 +20,21 @@
|
||||
|
||||
# What is VC Client
|
||||
|
||||
[VC Client](https://github.com/w-okada/voice-changer) is a client software for real-time voice changers that uses AI such as [MMVC](https://github.com/isletennos/MMVC_Trainer) and [so-vits-svc](https://github.com/svc-develop-team/so-vits-svc), [RVC(Retrieval-based-Voice-Conversion)](https://github.com/liujing04/Retrieval-based-Voice-Conversion-WebUI). It also provides an app for recording training audio for real-time voice changers, specifically for MMVC.
|
||||
1. This is a client software for performing real-time voice conversion using various Voice Conversion (VC) AI. The supported AI for voice conversion are as follows.
|
||||
|
||||
# Features
|
||||
- [MMVC](https://github.com/isletennos/MMVC_Trainer)
|
||||
- [so-vits-svc](https://github.com/svc-develop-team/so-vits-svc)
|
||||
- [RVC(Retrieval-based-Voice-Conversion)](https://github.com/liujing04/Retrieval-based-Voice-Conversion-WebUI)
|
||||
- [DDSP-SVC](https://github.com/yxlllc/DDSP-SVC)
|
||||
|
||||
1. Cross-platform compatibility
|
||||
Supports Windows, Mac (including Apple Silicon M1), Linux, and Google Colaboratory.
|
||||
|
||||
2. No need to install a separate audio recording app
|
||||
Audio recording can be done directly on the application hosted on Github Pages. Since it runs entirely on the browser, there is no need to install any special application. Additionally, since it works entirely as a browser application, no data is sent to the server.
|
||||
|
||||
3. Distribute the load by running Voice Changer on a different PC
|
||||
2. Distribute the load by running Voice Changer on a different PC
|
||||
The real-time voice changer of this application works on a server-client configuration. By running the MMVC server on a separate PC, you can run it while minimizing the impact on other resource-intensive processes such as gaming commentary.
|
||||
|
||||
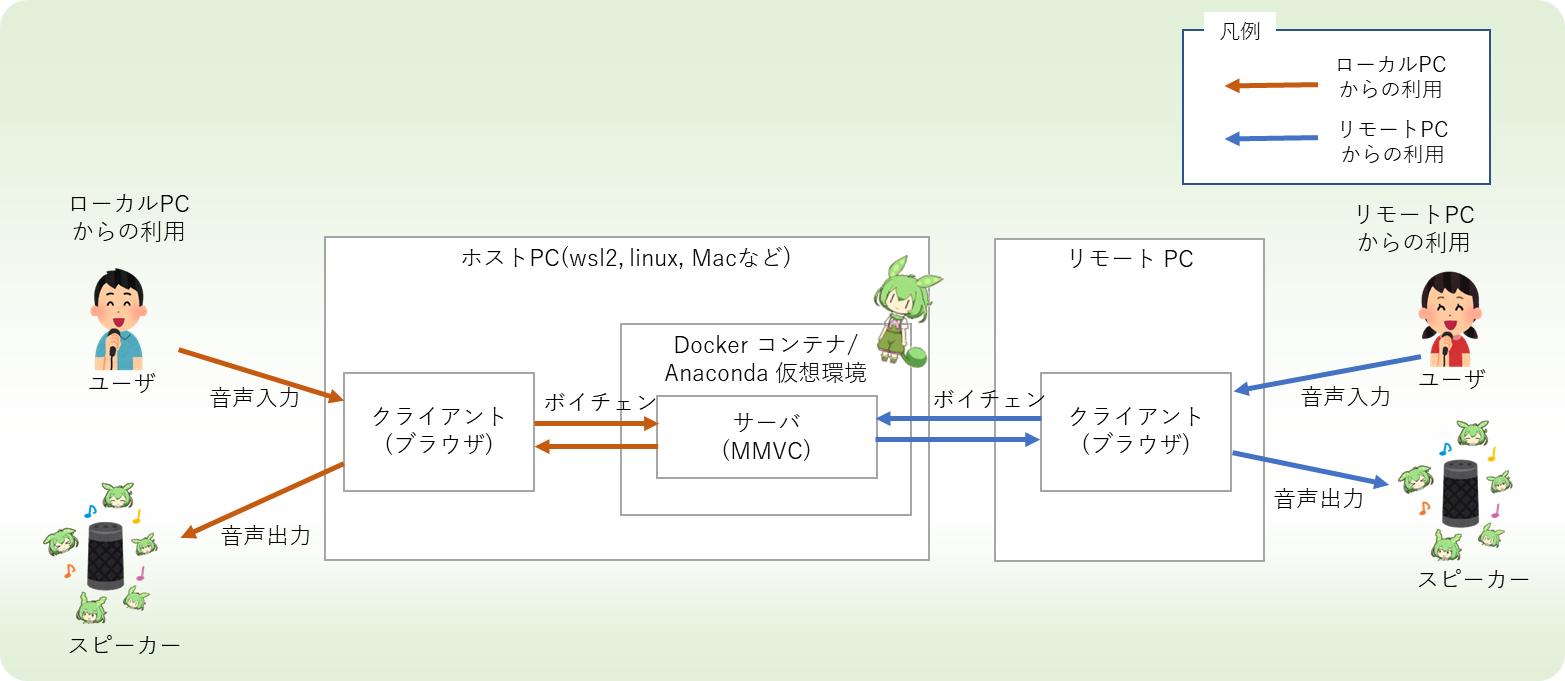
|
||||
|
||||
3. Cross-platform compatibility
|
||||
Supports Windows, Mac (including Apple Silicon M1), Linux, and Google Colaboratory.
|
||||
|
||||
# usage
|
||||
|
||||
Details are summarized [here](https://zenn.dev/wok/books/0004_vc-client-v_1_5_1_x).
|
||||
@ -58,30 +63,28 @@ You can run it on Google's machine learning platform, Colaboratory. If you have
|
||||
You can download and run executable binaries.
|
||||
We offer Windows and Mac versions.
|
||||
|
||||
- For Mac version, after unzipping the downloaded file, double-click the `startHttp.command` file corresponding to your VC. If a message indicating that the developer cannot be verified is displayed, please press the control key and click to run it again (or right-click to run it). (Details below \* 1)
|
||||
|
||||
- For Windows user, after unzipping the downloaded zip file, please run the `start_http.bat` file corresponding to your VC.
|
||||
|
||||
- For Mac version, after unzipping the downloaded file, double-click the `startHttp.command` file corresponding to your VC. If a message indicating that the developer cannot be verified is displayed, please press the control key and click to run it again (or right-click to run it).
|
||||
|
||||
- If you are connecting remotely, please use the `.command` file (Mac) or `.bat` file (Windows) with https instead of http.
|
||||
|
||||
- If you have an Nvidia GPU on Windows, it will usually work with the `ONNX(cpu,cuda),PyTorch(cpu)` version. In rare cases, the GPU may not be recognized, in which case please use the `ONNX(cpu,cuda), PyTorch(cpu,cuda)` version (which is much larger in size).
|
||||
- Tsukuyomi-chan, Ami-taro, Kogane Mahiro, and Kogane Kaigetsu require the Content Vec model for their actions. Please download the ContentVec_legacy 500 model from [this repository](https://github.com/auspicious3000/contentvec) and place it in the same folder as startHttp.command or start_http.bat to execute it.
|
||||
|
||||
- If you do not have an Nvidia GPU on Windows, it will usually work with the `ONNX(cpu,DirectML), PyTorch(cpu)` version.
|
||||
- For the operation of RVC (Retrieval-based-Voice-Conversion) on so-vits-svc 4.0/so-vits-svc 4.0v2, a model of hubert is required. Please download `hubert_base.pt` from [this repository](https://huggingface.co/lj1995/VoiceConversionWebUI/tree/main) and store it in the folder where the batch files are located.
|
||||
|
||||
- If you are using `so-vits-svc 4.0`/`so-vits-svc 4.0v2` on Windows, please use the `ONNX(cpu,cuda), PyTorch(cpu,cuda)` version.
|
||||
- To run DDSP-SVC, you need to download the hubert-soft and enhancer models. Download hubert-soft from [this link](https://github.com/bshall/hubert/releases/download/v0.1/hubert-soft-0d54a1f4.pt) and store it in the folder with the batch files. Download nsf_hifigan_20221211.zip from [this site](https://github.com/openvpi/vocoders/releases/tag/nsf-hifigan-v1) for enhancer. After unzipping, store the nsf_hifigan folder in the folder with the batch files.
|
||||
|
||||
- To use `so-vits-svc 4.0`/`so-vits-svc 4.0v2` or `tsukuyomi-chan`, you need the content vec model. Please download the ContentVec_legacy 500 model from [this repository](https://github.com/auspicious3000/contentvec), and place it in the same folder as `startHttp_xxx.command` or `start_http_xxx.bat` to run.
|
||||
- The encoder of DDPS-SVC only supports hubert-soft.
|
||||
|
||||
- You need to have the hubert model to use RVC(Retrieval-based-Voice-Conversion). Please download `hubert_base.pt` from [this repository](https://huggingface.co/lj1995/VoiceConversionWebUI/tree/main) and store it in the folder where the batch file is located.
|
||||
- Please refer to [here](tutorials/tutorial_rvc_en.md) for the description of each item of GUI to be used in RVC.
|
||||
|
||||
| Version | OS | Framework | link | VC Support | Size |
|
||||
| ---------- | --- | --------------------------------- | ------------------------------------------------------------------------------------------ | ----------------------------------------------------------------------------- | ------ |
|
||||
| v.1.5.2.4a | mac | ONNX(cpu,cuda), PyTorch(cpu,cuda) | [normal](https://drive.google.com/uc?id=1fR86gRWalhpi8kQURJmMfWuDvi53V2Ah&export=download) | MMVC v.1.5.x, MMVC v.1.3.x, so-vits-svc 4.0, RVC | 795MB |
|
||||
| | win | ONNX(cpu,cuda), PyTorch(cpu,cuda) | [normal](https://drive.google.com/uc?id=1lttvCgnZengcKkP4f0O2UBAVOcOph4b2&export=download) | MMVC v.1.5.x, MMVC v.1.3.x, so-vits-svc 4.0, so-vits-svc 4.0v2, RVC, DDSP-SVC | 2871MB |
|
||||
| v.1.5.2.4 | mac | ONNX(cpu,cuda), PyTorch(cpu,mps) | [normal](https://drive.google.com/uc?id=1UC0n6Lgyy4ugPznJ-Erd7lskKaOE6--X&export=download) | MMVC v.1.5.x, MMVC v.1.3.x, so-vits-svc 4.0, RVC | 795MB |
|
||||
| | win | ONNX(cpu,cuda), PyTorch(cpu,cuda) | [normal](https://drive.google.com/uc?id=1OmSug85MUR58cnYo_P6Xe_GtNAG7PkKO&export=download) | MMVC v.1.5.x, MMVC v.1.3.x, so-vits-svc 4.0, so-vits-svc 4.0v2, RVC, DDSP-SVC | 2871MB |
|
||||
- Download (When you cannot download from google drive, try [hugging_face](https://huggingface.co/wok000/vcclient000/tree/main))
|
||||
|
||||
\*\*\* [hugging_face](https://huggingface.co/wok000/vcclient/tree/main) (experimental)
|
||||
| Version | OS | フレームワーク | link | サポート VC | サイズ |
|
||||
| --------- | --- | --------------------------------- | ---------------------------------------------------------------------------------------- | ----------------------------------------------------------------------------- | ------ |
|
||||
| v.1.5.2.6 | mac | ONNX(cpu), PyTorch(cpu,mps) | [通常](https://drive.google.com/uc?id=1NTdtBeKU1bdQKP0_LpbmU3xAjuua1dCT&export=download) | MMVC v.1.5.x, MMVC v.1.3.x, so-vits-svc 4.0, RVC | 784MB |
|
||||
| | win | ONNX(cpu,cuda), PyTorch(cpu,cuda) | [通常](https://drive.google.com/uc?id=1XdoMQoghBOjW__rE2a02zMyQDz8Gi56n&export=download) | MMVC v.1.5.x, MMVC v.1.3.x, so-vits-svc 4.0, so-vits-svc 4.0v2, RVC, DDSP-SVC | 2861MB |
|
||||
|
||||
| Version | OS | Framework | link | VC Support | Size |
|
||||
| ---------- | ------------------------------------- | --------- | -------------------------------------------------------------------------------------------------- | ---------- | ----- |
|
||||
@ -94,17 +97,9 @@ We offer Windows and Mac versions.
|
||||
| | <span style="color: blue;">win</span> | - | [Kikoto Kurage](https://drive.google.com/uc?id=1fiymPcoYzwE1yxyIfC_FTPiFfGEC2jA8&export=download) | - | 823MB |
|
||||
| | <span style="color: blue;">win</span> | - | [Amitaro](https://drive.google.com/uc?id=1Vt4WBEOAz0EhIWs3ZRFIcg7ELtSHnYfe&export=download) | - | 821MB |
|
||||
|
||||
\*1 MMVC v.1.5.x is Experimental.
|
||||
\*1 Tsukuyo Michan uses free character "Tsukuyo Michan" voice data that is publicly available for free. (Details such as terms of use are at the end of the document)
|
||||
|
||||
\*2 Tsukuyo Michan uses free character "Tsukuyo Michan" voice data that is publicly available for free. (Details such as terms of use are at the end of the document)
|
||||
|
||||
\*3 If unpacking or starting is slow, there is a possibility that virus checking is running on your antivirus software. Please try running it with the file or folder excluded from the target. (At your own risk)
|
||||
|
||||
\*4 This software is not signed by the developer. A warning message will appear, but you can run the software by clicking the icon while holding down the control key. This is due to Apple's security policy. Running the software is at your own risk.
|
||||
|
||||
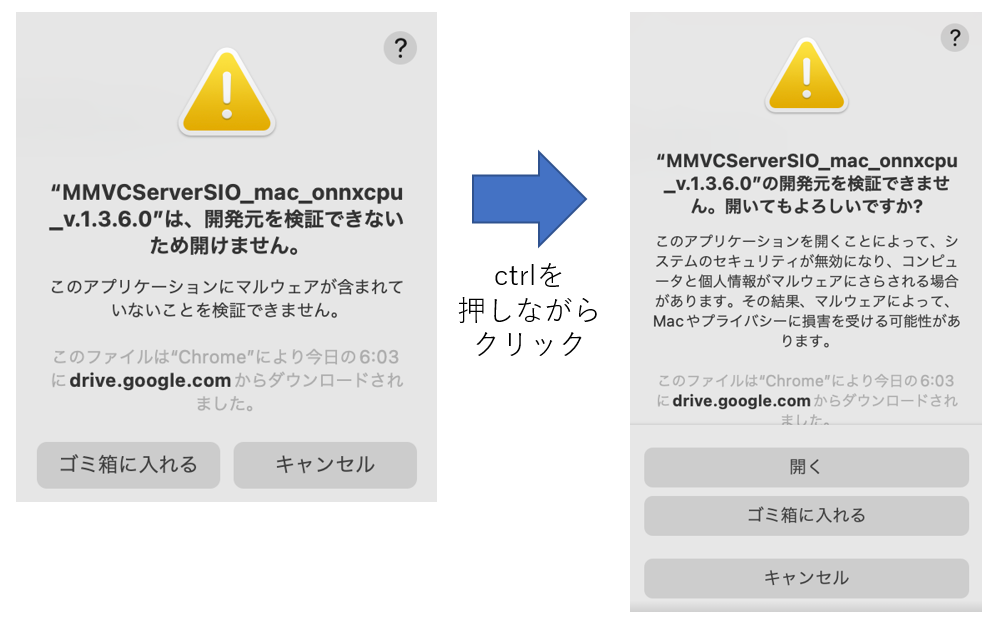
|
||||
|
||||
https://user-images.githubusercontent.com/48346627/212569645-e30b7f4e-079d-4504-8cf8-7816c5f40b00.mp4
|
||||
\*2 If unpacking or starting is slow, there is a possibility that virus checking is running on your antivirus software. Please try running it with the file or folder excluded from the target. (At your own risk)
|
||||
|
||||
## (2-3) Usage after setting up the environment such as Docker or Anaconda
|
||||
|
||||
@ -118,7 +113,7 @@ To run docker, see [start docker](docker_vcclient/README_en.md).
|
||||
|
||||
To run on Anaconda venv, see [server developer's guide](README_dev_en.md)
|
||||
|
||||
## Real-time performance
|
||||
# Real-time performance
|
||||
|
||||
Conversion is almost instantaneous when using GPU.
|
||||
|
||||
@ -130,6 +125,14 @@ https://twitter.com/DannadoriYellow/status/1613553862773997569?s=20&t=7CLD79h1F3
|
||||
|
||||
With an old CPU (i7-4770), it takes about 1000 msec for conversion.
|
||||
|
||||
# Software Signing
|
||||
|
||||
This software is not signed by the developer. A warning message will appear, but you can run the software by clicking the icon while holding down the control key. This is due to Apple's security policy. Running the software is at your own risk.
|
||||
|
||||
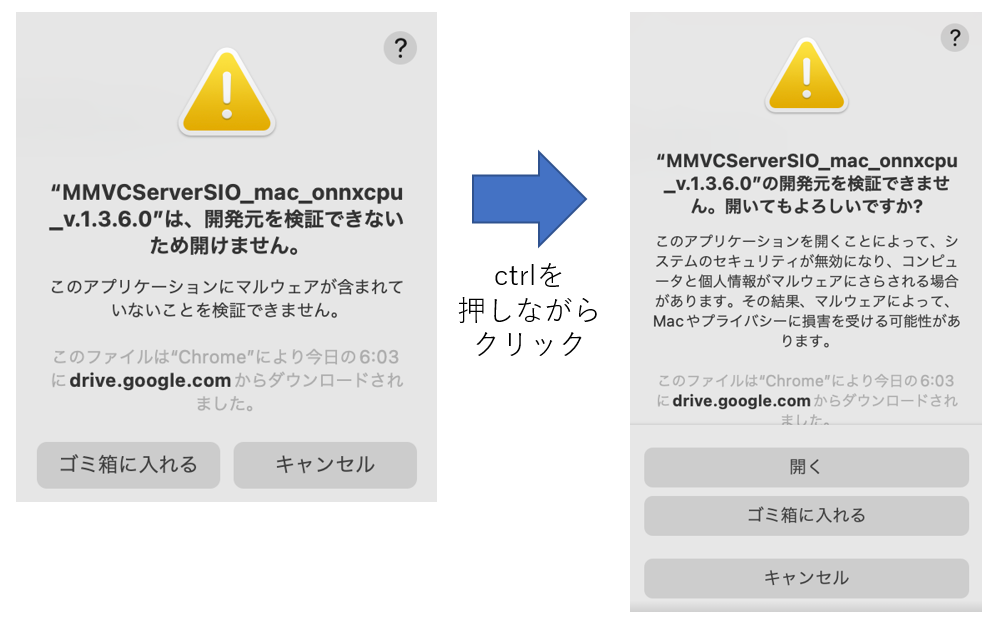
|
||||
|
||||
https://user-images.githubusercontent.com/48346627/212569645-e30b7f4e-079d-4504-8cf8-7816c5f40b00.mp4
|
||||
|
||||
# Acknowledgments
|
||||
|
||||
- [Tachizunda-mon materials](https://seiga.nicovideo.jp/seiga/im10792934)
|
||||
|
@ -43,6 +43,7 @@
|
||||
"showFeature": false,
|
||||
"showIndex": false,
|
||||
"showHalfPrecision": false,
|
||||
"showPyTorchEnableCheckBox": true,
|
||||
"defaultEnablePyTorch": true,
|
||||
|
||||
"showOnnxExportButton": false
|
||||
|
@ -40,6 +40,7 @@
|
||||
"showCorrespondence": false,
|
||||
"showPyTorchCluster": false,
|
||||
|
||||
"showPyTorchEnableCheckBox": true,
|
||||
"defaultEnablePyTorch": false
|
||||
}
|
||||
},
|
||||
|
@ -39,7 +39,7 @@
|
||||
"showPyTorch": true,
|
||||
"showCorrespondence": true,
|
||||
"showPyTorchCluster": false,
|
||||
|
||||
"showPyTorchEnableCheckBox": true,
|
||||
"defaultEnablePyTorch": false
|
||||
}
|
||||
},
|
||||
|
26
client/demo/dist/assets/gui_settings/RVC.json
vendored
26
client/demo/dist/assets/gui_settings/RVC.json
vendored
@ -36,6 +36,14 @@
|
||||
{
|
||||
"name": "onnxExport",
|
||||
"options": {}
|
||||
},
|
||||
{
|
||||
"name": "onnxExecutor",
|
||||
"options": {}
|
||||
},
|
||||
{
|
||||
"name": "modelSamplingRate",
|
||||
"options": {}
|
||||
}
|
||||
],
|
||||
"modelSetting": [
|
||||
@ -43,29 +51,23 @@
|
||||
"name": "modelUploader",
|
||||
"options": {
|
||||
"showModelSlot": true,
|
||||
"showFrameworkSelector": false,
|
||||
"showConfig": false,
|
||||
"showOnnx": true,
|
||||
"showPyTorch": true,
|
||||
"oneModelFileType": true,
|
||||
"showOnnx": false,
|
||||
"showPyTorch": false,
|
||||
"showCorrespondence": false,
|
||||
"showPyTorchCluster": false,
|
||||
|
||||
"showFeature": true,
|
||||
"showIndex": true,
|
||||
"showHalfPrecision": true,
|
||||
"showPyTorchEnableCheckBox": false,
|
||||
"defaultEnablePyTorch": true,
|
||||
"onlySelectedFramework": true,
|
||||
|
||||
"showDefaultTune": true
|
||||
}
|
||||
},
|
||||
{
|
||||
"name": "framework",
|
||||
"options": {
|
||||
"showFramework": true
|
||||
}
|
||||
},
|
||||
{
|
||||
"name": "modelSamplingRate",
|
||||
"options": {}
|
||||
}
|
||||
],
|
||||
"deviceSetting": [
|
||||
|
183
client/demo/dist/assets/gui_settings/RVC_CLASSIC.json
vendored
Normal file
183
client/demo/dist/assets/gui_settings/RVC_CLASSIC.json
vendored
Normal file
@ -0,0 +1,183 @@
|
||||
{
|
||||
"type": "demo",
|
||||
"id": "RVC",
|
||||
"front": {
|
||||
"title": [
|
||||
{
|
||||
"name": "title",
|
||||
"options": {
|
||||
"mainTitle": "Realtime Voice Changer Client",
|
||||
"subTitle": "for RVC",
|
||||
"lineNum": 1
|
||||
}
|
||||
},
|
||||
{
|
||||
"name": "clearSetting",
|
||||
"options": {}
|
||||
}
|
||||
],
|
||||
"serverControl": [
|
||||
{
|
||||
"name": "startButton",
|
||||
"options": {}
|
||||
},
|
||||
{
|
||||
"name": "performance",
|
||||
"options": {}
|
||||
},
|
||||
{
|
||||
"name": "serverInfo",
|
||||
"options": {}
|
||||
},
|
||||
{
|
||||
"name": "modelSwitch",
|
||||
"options": {}
|
||||
},
|
||||
{
|
||||
"name": "onnxExport",
|
||||
"options": {}
|
||||
}
|
||||
],
|
||||
"modelSetting": [
|
||||
{
|
||||
"name": "modelUploader",
|
||||
"options": {
|
||||
"showModelSlot": true,
|
||||
"showConfig": false,
|
||||
"showOnnx": true,
|
||||
"showPyTorch": true,
|
||||
"showCorrespondence": false,
|
||||
"showPyTorchCluster": false,
|
||||
|
||||
"showFeature": true,
|
||||
"showIndex": true,
|
||||
"showHalfPrecision": true,
|
||||
"defaultEnablePyTorch": true,
|
||||
|
||||
"showDefaultTune": true
|
||||
}
|
||||
},
|
||||
{
|
||||
"name": "framework",
|
||||
"options": {
|
||||
"showFramework": true
|
||||
}
|
||||
},
|
||||
{
|
||||
"name": "modelSamplingRate",
|
||||
"options": {}
|
||||
}
|
||||
],
|
||||
"deviceSetting": [
|
||||
{
|
||||
"name": "audioInput",
|
||||
"options": {}
|
||||
},
|
||||
{
|
||||
"name": "audioOutput",
|
||||
"options": {}
|
||||
}
|
||||
],
|
||||
"qualityControl": [
|
||||
{
|
||||
"name": "noiseControl",
|
||||
"options": {}
|
||||
},
|
||||
{
|
||||
"name": "gainControl",
|
||||
"options": {}
|
||||
},
|
||||
{
|
||||
"name": "f0Detector",
|
||||
"options": {
|
||||
"detectors": ["pm", "harvest"]
|
||||
}
|
||||
},
|
||||
{
|
||||
"name": "divider",
|
||||
"options": {}
|
||||
},
|
||||
{
|
||||
"name": "analyzer",
|
||||
"options": {}
|
||||
}
|
||||
],
|
||||
"speakerSetting": [
|
||||
{
|
||||
"name": "dstId",
|
||||
"options": {
|
||||
"showF0": true,
|
||||
"useServerInfo": false
|
||||
}
|
||||
},
|
||||
{
|
||||
"name": "tune",
|
||||
"options": {}
|
||||
},
|
||||
{
|
||||
"name": "indexRatio",
|
||||
"options": {}
|
||||
},
|
||||
{
|
||||
"name": "silentThreshold",
|
||||
"options": {}
|
||||
}
|
||||
],
|
||||
"converterSetting": [
|
||||
{
|
||||
"name": "inputChunkNum",
|
||||
"options": {}
|
||||
},
|
||||
{
|
||||
"name": "extraDataLength",
|
||||
"options": {}
|
||||
},
|
||||
{
|
||||
"name": "gpu",
|
||||
"options": {}
|
||||
}
|
||||
],
|
||||
"advancedSetting": [
|
||||
{
|
||||
"name": "protocol",
|
||||
"options": {}
|
||||
},
|
||||
{
|
||||
"name": "crossFadeOverlapSize",
|
||||
"options": {}
|
||||
},
|
||||
{
|
||||
"name": "crossFadeOffsetRate",
|
||||
"options": {}
|
||||
},
|
||||
{
|
||||
"name": "crossFadeEndRate",
|
||||
"options": {}
|
||||
},
|
||||
{
|
||||
"name": "trancateNumThreshold",
|
||||
"options": {}
|
||||
},
|
||||
{
|
||||
"name": "rvcQuality",
|
||||
"options": {}
|
||||
},
|
||||
{
|
||||
"name": "silenceFront",
|
||||
"options": {}
|
||||
}
|
||||
]
|
||||
},
|
||||
|
||||
"dialogs": {
|
||||
"license": [
|
||||
{
|
||||
"title": "Retrieval-based-Voice-Conversion-WebUI",
|
||||
"auther": "liujing04",
|
||||
"contact": "",
|
||||
"url": "https://github.com/liujing04/Retrieval-based-Voice-Conversion-WebUI",
|
||||
"license": "MIT"
|
||||
}
|
||||
]
|
||||
}
|
||||
}
|
@ -39,7 +39,7 @@
|
||||
"showPyTorch": true,
|
||||
"showCorrespondence": false,
|
||||
"showPyTorchCluster": true,
|
||||
|
||||
"showPyTorchEnableCheckBox": true,
|
||||
"defaultEnablePyTorch": true
|
||||
}
|
||||
},
|
||||
|
@ -39,7 +39,7 @@
|
||||
"showPyTorch": true,
|
||||
"showCorrespondence": false,
|
||||
"showPyTorchCluster": true,
|
||||
|
||||
"showPyTorchEnableCheckBox": true,
|
||||
"defaultEnablePyTorch": true
|
||||
}
|
||||
},
|
||||
|
2
client/demo/dist/index.js
vendored
2
client/demo/dist/index.js
vendored
File diff suppressed because one or more lines are too long
2676
client/demo/dist/index.js.LICENSE.txt
vendored
2676
client/demo/dist/index.js.LICENSE.txt
vendored
File diff suppressed because it is too large
Load Diff
25
client/demo/package-lock.json
generated
25
client/demo/package-lock.json
generated
@ -23,7 +23,7 @@
|
||||
"@babel/preset-env": "^7.21.4",
|
||||
"@babel/preset-react": "^7.18.6",
|
||||
"@babel/preset-typescript": "^7.21.4",
|
||||
"@types/node": "^18.15.13",
|
||||
"@types/node": "^18.16.0",
|
||||
"@types/react": "^18.0.38",
|
||||
"@types/react-dom": "^18.0.11",
|
||||
"autoprefixer": "^10.4.14",
|
||||
@ -40,7 +40,7 @@
|
||||
"npm-run-all": "^4.1.5",
|
||||
"postcss-loader": "^7.2.4",
|
||||
"postcss-nested": "^6.0.1",
|
||||
"prettier": "^2.8.7",
|
||||
"prettier": "^2.8.8",
|
||||
"rimraf": "^5.0.0",
|
||||
"style-loader": "^3.3.2",
|
||||
"ts-loader": "^9.4.2",
|
||||
@ -3691,9 +3691,9 @@
|
||||
"license": "MIT"
|
||||
},
|
||||
"node_modules/@types/node": {
|
||||
"version": "18.15.13",
|
||||
"resolved": "https://registry.npmjs.org/@types/node/-/node-18.15.13.tgz",
|
||||
"integrity": "sha512-N+0kuo9KgrUQ1Sn/ifDXsvg0TTleP7rIy4zOBGECxAljqvqfqpTfzx0Q1NUedOixRMBfe2Whhb056a42cWs26Q=="
|
||||
"version": "18.16.0",
|
||||
"resolved": "https://registry.npmjs.org/@types/node/-/node-18.16.0.tgz",
|
||||
"integrity": "sha512-BsAaKhB+7X+H4GnSjGhJG9Qi8Tw+inU9nJDwmD5CgOmBLEI6ArdhikpLX7DjbjDRDTbqZzU2LSQNZg8WGPiSZQ=="
|
||||
},
|
||||
"node_modules/@types/prop-types": {
|
||||
"version": "15.7.5",
|
||||
@ -8489,9 +8489,10 @@
|
||||
}
|
||||
},
|
||||
"node_modules/prettier": {
|
||||
"version": "2.8.7",
|
||||
"version": "2.8.8",
|
||||
"resolved": "https://registry.npmjs.org/prettier/-/prettier-2.8.8.tgz",
|
||||
"integrity": "sha512-tdN8qQGvNjw4CHbY+XXk0JgCXn9QiF21a55rBe5LJAU+kDyC4WQn4+awm2Xfk2lQMk5fKup9XgzTZtGkjBdP9Q==",
|
||||
"dev": true,
|
||||
"license": "MIT",
|
||||
"bin": {
|
||||
"prettier": "bin-prettier.js"
|
||||
},
|
||||
@ -13358,9 +13359,9 @@
|
||||
"dev": true
|
||||
},
|
||||
"@types/node": {
|
||||
"version": "18.15.13",
|
||||
"resolved": "https://registry.npmjs.org/@types/node/-/node-18.15.13.tgz",
|
||||
"integrity": "sha512-N+0kuo9KgrUQ1Sn/ifDXsvg0TTleP7rIy4zOBGECxAljqvqfqpTfzx0Q1NUedOixRMBfe2Whhb056a42cWs26Q=="
|
||||
"version": "18.16.0",
|
||||
"resolved": "https://registry.npmjs.org/@types/node/-/node-18.16.0.tgz",
|
||||
"integrity": "sha512-BsAaKhB+7X+H4GnSjGhJG9Qi8Tw+inU9nJDwmD5CgOmBLEI6ArdhikpLX7DjbjDRDTbqZzU2LSQNZg8WGPiSZQ=="
|
||||
},
|
||||
"@types/prop-types": {
|
||||
"version": "15.7.5",
|
||||
@ -16405,7 +16406,9 @@
|
||||
"dev": true
|
||||
},
|
||||
"prettier": {
|
||||
"version": "2.8.7",
|
||||
"version": "2.8.8",
|
||||
"resolved": "https://registry.npmjs.org/prettier/-/prettier-2.8.8.tgz",
|
||||
"integrity": "sha512-tdN8qQGvNjw4CHbY+XXk0JgCXn9QiF21a55rBe5LJAU+kDyC4WQn4+awm2Xfk2lQMk5fKup9XgzTZtGkjBdP9Q==",
|
||||
"dev": true
|
||||
},
|
||||
"prettier-linter-helpers": {
|
||||
|
@ -23,7 +23,7 @@
|
||||
"@babel/preset-env": "^7.21.4",
|
||||
"@babel/preset-react": "^7.18.6",
|
||||
"@babel/preset-typescript": "^7.21.4",
|
||||
"@types/node": "^18.15.13",
|
||||
"@types/node": "^18.16.0",
|
||||
"@types/react": "^18.0.38",
|
||||
"@types/react-dom": "^18.0.11",
|
||||
"autoprefixer": "^10.4.14",
|
||||
@ -40,7 +40,7 @@
|
||||
"npm-run-all": "^4.1.5",
|
||||
"postcss-loader": "^7.2.4",
|
||||
"postcss-nested": "^6.0.1",
|
||||
"prettier": "^2.8.7",
|
||||
"prettier": "^2.8.8",
|
||||
"rimraf": "^5.0.0",
|
||||
"style-loader": "^3.3.2",
|
||||
"ts-loader": "^9.4.2",
|
||||
|
@ -43,6 +43,7 @@
|
||||
"showFeature": false,
|
||||
"showIndex": false,
|
||||
"showHalfPrecision": false,
|
||||
"showPyTorchEnableCheckBox": true,
|
||||
"defaultEnablePyTorch": true,
|
||||
|
||||
"showOnnxExportButton": false
|
||||
|
@ -40,6 +40,7 @@
|
||||
"showCorrespondence": false,
|
||||
"showPyTorchCluster": false,
|
||||
|
||||
"showPyTorchEnableCheckBox": true,
|
||||
"defaultEnablePyTorch": false
|
||||
}
|
||||
},
|
||||
|
@ -39,7 +39,7 @@
|
||||
"showPyTorch": true,
|
||||
"showCorrespondence": true,
|
||||
"showPyTorchCluster": false,
|
||||
|
||||
"showPyTorchEnableCheckBox": true,
|
||||
"defaultEnablePyTorch": false
|
||||
}
|
||||
},
|
||||
|
@ -36,6 +36,14 @@
|
||||
{
|
||||
"name": "onnxExport",
|
||||
"options": {}
|
||||
},
|
||||
{
|
||||
"name": "onnxExecutor",
|
||||
"options": {}
|
||||
},
|
||||
{
|
||||
"name": "modelSamplingRate",
|
||||
"options": {}
|
||||
}
|
||||
],
|
||||
"modelSetting": [
|
||||
@ -43,29 +51,23 @@
|
||||
"name": "modelUploader",
|
||||
"options": {
|
||||
"showModelSlot": true,
|
||||
"showFrameworkSelector": false,
|
||||
"showConfig": false,
|
||||
"showOnnx": true,
|
||||
"showPyTorch": true,
|
||||
"oneModelFileType": true,
|
||||
"showOnnx": false,
|
||||
"showPyTorch": false,
|
||||
"showCorrespondence": false,
|
||||
"showPyTorchCluster": false,
|
||||
|
||||
"showFeature": true,
|
||||
"showIndex": true,
|
||||
"showHalfPrecision": true,
|
||||
"showPyTorchEnableCheckBox": false,
|
||||
"defaultEnablePyTorch": true,
|
||||
"onlySelectedFramework": true,
|
||||
|
||||
"showDefaultTune": true
|
||||
}
|
||||
},
|
||||
{
|
||||
"name": "framework",
|
||||
"options": {
|
||||
"showFramework": true
|
||||
}
|
||||
},
|
||||
{
|
||||
"name": "modelSamplingRate",
|
||||
"options": {}
|
||||
}
|
||||
],
|
||||
"deviceSetting": [
|
||||
|
183
client/demo/public/assets/gui_settings/RVC_CLASSIC.json
Normal file
183
client/demo/public/assets/gui_settings/RVC_CLASSIC.json
Normal file
@ -0,0 +1,183 @@
|
||||
{
|
||||
"type": "demo",
|
||||
"id": "RVC",
|
||||
"front": {
|
||||
"title": [
|
||||
{
|
||||
"name": "title",
|
||||
"options": {
|
||||
"mainTitle": "Realtime Voice Changer Client",
|
||||
"subTitle": "for RVC",
|
||||
"lineNum": 1
|
||||
}
|
||||
},
|
||||
{
|
||||
"name": "clearSetting",
|
||||
"options": {}
|
||||
}
|
||||
],
|
||||
"serverControl": [
|
||||
{
|
||||
"name": "startButton",
|
||||
"options": {}
|
||||
},
|
||||
{
|
||||
"name": "performance",
|
||||
"options": {}
|
||||
},
|
||||
{
|
||||
"name": "serverInfo",
|
||||
"options": {}
|
||||
},
|
||||
{
|
||||
"name": "modelSwitch",
|
||||
"options": {}
|
||||
},
|
||||
{
|
||||
"name": "onnxExport",
|
||||
"options": {}
|
||||
}
|
||||
],
|
||||
"modelSetting": [
|
||||
{
|
||||
"name": "modelUploader",
|
||||
"options": {
|
||||
"showModelSlot": true,
|
||||
"showConfig": false,
|
||||
"showOnnx": true,
|
||||
"showPyTorch": true,
|
||||
"showCorrespondence": false,
|
||||
"showPyTorchCluster": false,
|
||||
|
||||
"showFeature": true,
|
||||
"showIndex": true,
|
||||
"showHalfPrecision": true,
|
||||
"defaultEnablePyTorch": true,
|
||||
|
||||
"showDefaultTune": true
|
||||
}
|
||||
},
|
||||
{
|
||||
"name": "framework",
|
||||
"options": {
|
||||
"showFramework": true
|
||||
}
|
||||
},
|
||||
{
|
||||
"name": "modelSamplingRate",
|
||||
"options": {}
|
||||
}
|
||||
],
|
||||
"deviceSetting": [
|
||||
{
|
||||
"name": "audioInput",
|
||||
"options": {}
|
||||
},
|
||||
{
|
||||
"name": "audioOutput",
|
||||
"options": {}
|
||||
}
|
||||
],
|
||||
"qualityControl": [
|
||||
{
|
||||
"name": "noiseControl",
|
||||
"options": {}
|
||||
},
|
||||
{
|
||||
"name": "gainControl",
|
||||
"options": {}
|
||||
},
|
||||
{
|
||||
"name": "f0Detector",
|
||||
"options": {
|
||||
"detectors": ["pm", "harvest"]
|
||||
}
|
||||
},
|
||||
{
|
||||
"name": "divider",
|
||||
"options": {}
|
||||
},
|
||||
{
|
||||
"name": "analyzer",
|
||||
"options": {}
|
||||
}
|
||||
],
|
||||
"speakerSetting": [
|
||||
{
|
||||
"name": "dstId",
|
||||
"options": {
|
||||
"showF0": true,
|
||||
"useServerInfo": false
|
||||
}
|
||||
},
|
||||
{
|
||||
"name": "tune",
|
||||
"options": {}
|
||||
},
|
||||
{
|
||||
"name": "indexRatio",
|
||||
"options": {}
|
||||
},
|
||||
{
|
||||
"name": "silentThreshold",
|
||||
"options": {}
|
||||
}
|
||||
],
|
||||
"converterSetting": [
|
||||
{
|
||||
"name": "inputChunkNum",
|
||||
"options": {}
|
||||
},
|
||||
{
|
||||
"name": "extraDataLength",
|
||||
"options": {}
|
||||
},
|
||||
{
|
||||
"name": "gpu",
|
||||
"options": {}
|
||||
}
|
||||
],
|
||||
"advancedSetting": [
|
||||
{
|
||||
"name": "protocol",
|
||||
"options": {}
|
||||
},
|
||||
{
|
||||
"name": "crossFadeOverlapSize",
|
||||
"options": {}
|
||||
},
|
||||
{
|
||||
"name": "crossFadeOffsetRate",
|
||||
"options": {}
|
||||
},
|
||||
{
|
||||
"name": "crossFadeEndRate",
|
||||
"options": {}
|
||||
},
|
||||
{
|
||||
"name": "trancateNumThreshold",
|
||||
"options": {}
|
||||
},
|
||||
{
|
||||
"name": "rvcQuality",
|
||||
"options": {}
|
||||
},
|
||||
{
|
||||
"name": "silenceFront",
|
||||
"options": {}
|
||||
}
|
||||
]
|
||||
},
|
||||
|
||||
"dialogs": {
|
||||
"license": [
|
||||
{
|
||||
"title": "Retrieval-based-Voice-Conversion-WebUI",
|
||||
"auther": "liujing04",
|
||||
"contact": "",
|
||||
"url": "https://github.com/liujing04/Retrieval-based-Voice-Conversion-WebUI",
|
||||
"license": "MIT"
|
||||
}
|
||||
]
|
||||
}
|
||||
}
|
@ -39,7 +39,7 @@
|
||||
"showPyTorch": true,
|
||||
"showCorrespondence": false,
|
||||
"showPyTorchCluster": true,
|
||||
|
||||
"showPyTorchEnableCheckBox": true,
|
||||
"defaultEnablePyTorch": true
|
||||
}
|
||||
},
|
||||
|
@ -39,7 +39,7 @@
|
||||
"showPyTorch": true,
|
||||
"showCorrespondence": false,
|
||||
"showPyTorchCluster": true,
|
||||
|
||||
"showPyTorchEnableCheckBox": true,
|
||||
"defaultEnablePyTorch": true
|
||||
}
|
||||
},
|
||||
|
@ -42,6 +42,7 @@ import { DstIdRow2, DstIdRow2Props } from "./components/602v2_DstIdRow2"
|
||||
import { SilenceFrontRow, SilenceFrontRowProps } from "./components/812_SilenceFrontRow"
|
||||
import { ModelSwitchRow, ModelSwitchRowProps } from "./components/204_ModelSwitchRow"
|
||||
import { ONNXExportRow, ONNXExportRowProps } from "./components/205_ONNXExportRow"
|
||||
import { ONNXExecutorRow, ONNXExecutorRowProps } from "./components/206_ONNXExecutorRow"
|
||||
|
||||
export const catalog: { [key: string]: (props: any) => JSX.Element } = {}
|
||||
|
||||
@ -68,6 +69,7 @@ const initialize = () => {
|
||||
addToCatalog("serverInfo", (props: ServerInfoRowProps) => { return <ServerInfoRow {...props} /> })
|
||||
addToCatalog("modelSwitch", (props: ModelSwitchRowProps) => { return <ModelSwitchRow {...props} /> })
|
||||
addToCatalog("onnxExport", (props: ONNXExportRowProps) => { return <ONNXExportRow {...props} /> })
|
||||
addToCatalog("onnxExecutor", (props: ONNXExecutorRowProps) => { return <ONNXExecutorRow {...props} /> })
|
||||
|
||||
|
||||
|
||||
|
@ -1,3 +1,4 @@
|
||||
import { Framework } from "@dannadori/voice-changer-client-js"
|
||||
import React, { useMemo } from "react"
|
||||
import { useAppState } from "../../../001_provider/001_AppStateProvider"
|
||||
|
||||
@ -9,23 +10,40 @@ export const ModelSwitchRow = (_props: ModelSwitchRowProps) => {
|
||||
const appState = useAppState()
|
||||
|
||||
const modelSwitchRow = useMemo(() => {
|
||||
const slot = appState.serverSetting.serverSetting.modelSlotIndex
|
||||
|
||||
const onSwitchModelClicked = (index: number) => {
|
||||
|
||||
appState.serverSetting.updateServerSettings({ ...appState.serverSetting.serverSetting, modelSlotIndex: index })
|
||||
const onSwitchModelClicked = async (index: number, filename: string) => {
|
||||
const framework: Framework = filename.endsWith(".onnx") ? "ONNX" : "PyTorch"
|
||||
|
||||
// Quick hack for same slot is selected. 下3桁が実際のSlotID
|
||||
const dummyModelSlotIndex = (Math.floor(Date.now() / 1000)) * 1000 + index
|
||||
await appState.serverSetting.updateServerSettings({ ...appState.serverSetting.serverSetting, modelSlotIndex: dummyModelSlotIndex, framework: framework })
|
||||
}
|
||||
let filename = ""
|
||||
const modelOptions = appState.serverSetting.serverSetting.modelSlots.map((x, index) => {
|
||||
const className = index == slot ? "body-button-active left-margin-1" : "body-button left-margin-1"
|
||||
let filename = ""
|
||||
if (x.pyTorchModelFile && x.pyTorchModelFile.length > 0) {
|
||||
filename = x.pyTorchModelFile.replace(/^.*[\\\/]/, '')
|
||||
return <div key={index} className="body-button left-margin-1" onClick={() => { onSwitchModelClicked(index) }}>{filename}</div>
|
||||
} else if (x.onnxModelFile && x.onnxModelFile.length > 0) {
|
||||
filename = x.onnxModelFile.replace(/^.*[\\\/]/, '')
|
||||
return <div key={index} className="body-button left-margin-1" onClick={() => { onSwitchModelClicked(index) }}>{filename}</div>
|
||||
} else {
|
||||
return <div key={index} ></div>
|
||||
}
|
||||
const f0str = x.f0 == true ? "f0" : "nof0"
|
||||
const srstr = Math.floor(x.samplingRate / 1000) + "K"
|
||||
const embedstr = x.embChannels
|
||||
const typestr = x.modelType == 0 ? "org" : "webui"
|
||||
const metadata = x.deprecated ? "[deprecated version]" : `[${f0str},${srstr},${embedstr},${typestr}]`
|
||||
|
||||
|
||||
return (
|
||||
<div key={index} className={className} onClick={() => { onSwitchModelClicked(index, filename) }}>
|
||||
<div>
|
||||
{filename}
|
||||
</div>
|
||||
<div>{metadata}</div>
|
||||
</div>
|
||||
)
|
||||
|
||||
})
|
||||
|
||||
|
@ -12,6 +12,10 @@ export const ONNXExportRow = (_props: ONNXExportRowProps) => {
|
||||
const guiState = useGuiState()
|
||||
|
||||
const onnxExporthRow = useMemo(() => {
|
||||
if (appState.serverSetting.serverSetting.framework != "PyTorch") {
|
||||
return <></>
|
||||
}
|
||||
|
||||
const onnxExportButtonAction = async () => {
|
||||
|
||||
if (guiState.isConverting) {
|
||||
|
@ -0,0 +1,42 @@
|
||||
import { OnnxExecutionProvider } from "@dannadori/voice-changer-client-js"
|
||||
import React, { useMemo } from "react"
|
||||
import { useAppState } from "../../../001_provider/001_AppStateProvider"
|
||||
|
||||
|
||||
export type ONNXExecutorRowProps = {
|
||||
}
|
||||
|
||||
export const ONNXExecutorRow = (_props: ONNXExecutorRowProps) => {
|
||||
const appState = useAppState()
|
||||
|
||||
const onnxExecutorRow = useMemo(() => {
|
||||
if (appState.serverSetting.serverSetting.framework != "ONNX") {
|
||||
return <></>
|
||||
}
|
||||
const onOnnxExecutionProviderChanged = async (val: OnnxExecutionProvider) => {
|
||||
appState.serverSetting.updateServerSettings({ ...appState.serverSetting.serverSetting, onnxExecutionProvider: val })
|
||||
}
|
||||
|
||||
return (
|
||||
<div className="body-row split-3-7 left-padding-1">
|
||||
<div className="body-item-title left-padding-2">OnnxExecutionProvider</div>
|
||||
<div className="body-select-container">
|
||||
<select className="body-select" value={appState.serverSetting.serverSetting.onnxExecutionProvider} onChange={(e) => {
|
||||
onOnnxExecutionProviderChanged(e.target.value as
|
||||
OnnxExecutionProvider)
|
||||
}}>
|
||||
{
|
||||
Object.values(OnnxExecutionProvider).map(x => {
|
||||
return <option key={x} value={x}>{x}</option>
|
||||
})
|
||||
}
|
||||
</select>
|
||||
</div>
|
||||
</div>
|
||||
|
||||
)
|
||||
}, [appState.getInfo, appState.serverSetting.serverSetting])
|
||||
|
||||
return onnxExecutorRow
|
||||
}
|
||||
|
@ -0,0 +1,73 @@
|
||||
import React, { useMemo } from "react"
|
||||
import { fileSelector } from "@dannadori/voice-changer-client-js"
|
||||
import { useAppState } from "../../../001_provider/001_AppStateProvider"
|
||||
import { useGuiState } from "../001_GuiStateProvider"
|
||||
|
||||
|
||||
export const ModelSelectRow = () => {
|
||||
const appState = useAppState()
|
||||
const guiState = useGuiState()
|
||||
|
||||
|
||||
const onnxSelectRow = useMemo(() => {
|
||||
const slot = guiState.modelSlotNum
|
||||
const fileUploadSetting = appState.serverSetting.fileUploadSettings[slot]
|
||||
if (!fileUploadSetting) {
|
||||
return <></>
|
||||
}
|
||||
|
||||
const onnxModelFilenameText = fileUploadSetting.onnxModel?.filename || fileUploadSetting.onnxModel?.file?.name || ""
|
||||
const pyTorchFilenameText = fileUploadSetting.pyTorchModel?.filename || fileUploadSetting.pyTorchModel?.file?.name || ""
|
||||
const modelFilenameText = onnxModelFilenameText + pyTorchFilenameText
|
||||
|
||||
const onModelFileLoadClicked = async () => {
|
||||
const file = await fileSelector("")
|
||||
if (file.name.endsWith(".onnx") == false && file.name.endsWith(".pth") == false) {
|
||||
alert("モデルファイルの拡張子は.onnxか.pthである必要があります。(Extension of the model file should be .onnx or .pth.)")
|
||||
return
|
||||
}
|
||||
if (file.name.endsWith(".onnx") == true) {
|
||||
appState.serverSetting.setFileUploadSetting(slot, {
|
||||
...appState.serverSetting.fileUploadSettings[slot],
|
||||
onnxModel: {
|
||||
file: file
|
||||
},
|
||||
pyTorchModel: null
|
||||
})
|
||||
return
|
||||
}
|
||||
if (file.name.endsWith(".pth") == true) {
|
||||
appState.serverSetting.setFileUploadSetting(slot, {
|
||||
...appState.serverSetting.fileUploadSettings[slot],
|
||||
pyTorchModel: {
|
||||
file: file
|
||||
},
|
||||
onnxModel: null
|
||||
})
|
||||
return
|
||||
}
|
||||
}
|
||||
const onModelFileClearClicked = () => {
|
||||
appState.serverSetting.setFileUploadSetting(slot, {
|
||||
...appState.serverSetting.fileUploadSettings[slot],
|
||||
onnxModel: null,
|
||||
pyTorchModel: null
|
||||
})
|
||||
}
|
||||
|
||||
return (
|
||||
<div className="body-row split-3-3-4 left-padding-1 guided">
|
||||
<div className="body-item-title left-padding-2">Model(.onnx or .pth)</div>
|
||||
<div className="body-item-text">
|
||||
<div>{modelFilenameText}</div>
|
||||
</div>
|
||||
<div className="body-button-container">
|
||||
<div className="body-button" onClick={onModelFileLoadClicked}>select</div>
|
||||
<div className="body-button left-margin-1" onClick={onModelFileClearClicked}>clear</div>
|
||||
</div>
|
||||
</div>
|
||||
)
|
||||
}, [appState.serverSetting.fileUploadSettings, appState.serverSetting.setFileUploadSetting, guiState.modelSlotNum])
|
||||
|
||||
return onnxSelectRow
|
||||
}
|
@ -3,13 +3,21 @@ import { fileSelector } from "@dannadori/voice-changer-client-js"
|
||||
import { useAppState } from "../../../001_provider/001_AppStateProvider"
|
||||
import { useGuiState } from "../001_GuiStateProvider"
|
||||
|
||||
export const ONNXSelectRow = () => {
|
||||
type ONNXSelectRowProps = {
|
||||
onlyWhenSelected: boolean
|
||||
}
|
||||
|
||||
export const ONNXSelectRow = (props: ONNXSelectRowProps) => {
|
||||
const appState = useAppState()
|
||||
const guiState = useGuiState()
|
||||
|
||||
|
||||
const onnxSelectRow = useMemo(() => {
|
||||
const slot = guiState.modelSlotNum
|
||||
if (props.onlyWhenSelected && appState.serverSetting.fileUploadSettings[slot]?.framework != "ONNX") {
|
||||
return <></>
|
||||
}
|
||||
|
||||
const onnxModelFilenameText = appState.serverSetting.fileUploadSettings[slot]?.onnxModel?.filename || appState.serverSetting.fileUploadSettings[slot]?.onnxModel?.file?.name || ""
|
||||
const onOnnxFileLoadClicked = async () => {
|
||||
const file = await fileSelector("")
|
||||
|
@ -3,15 +3,24 @@ import { fileSelector } from "@dannadori/voice-changer-client-js"
|
||||
import { useAppState } from "../../../001_provider/001_AppStateProvider"
|
||||
import { useGuiState } from "../001_GuiStateProvider"
|
||||
|
||||
export type PyTorchSelectRow = {
|
||||
export type PyTorchSelectRowProps = {
|
||||
onlyWhenSelected: boolean
|
||||
}
|
||||
|
||||
export const PyTorchSelectRow = (_props: PyTorchSelectRow) => {
|
||||
export const PyTorchSelectRow = (props: PyTorchSelectRowProps) => {
|
||||
const appState = useAppState()
|
||||
const guiState = useGuiState()
|
||||
|
||||
const pyTorchSelectRow = useMemo(() => {
|
||||
if (guiState.showPyTorchModelUpload == false) {
|
||||
return <></>
|
||||
}
|
||||
const slot = guiState.modelSlotNum
|
||||
if (props.onlyWhenSelected && appState.serverSetting.fileUploadSettings[slot]?.framework != "PyTorch") {
|
||||
return <></>
|
||||
}
|
||||
|
||||
|
||||
const pyTorchFilenameText = appState.serverSetting.fileUploadSettings[slot]?.pyTorchModel?.filename || appState.serverSetting.fileUploadSettings[slot]?.pyTorchModel?.file?.name || ""
|
||||
const onPyTorchFileLoadClicked = async () => {
|
||||
const file = await fileSelector("")
|
||||
|
@ -9,6 +9,11 @@ export const HalfPrecisionRow = () => {
|
||||
|
||||
const halfPrecisionSelectRow = useMemo(() => {
|
||||
const slot = guiState.modelSlotNum
|
||||
const fileUploadSetting = appState.serverSetting.fileUploadSettings[slot]
|
||||
if (!fileUploadSetting) {
|
||||
return <></>
|
||||
}
|
||||
const currentValue = fileUploadSetting ? fileUploadSetting.isHalf : true
|
||||
const onHalfPrecisionChanged = () => {
|
||||
appState.serverSetting.setFileUploadSetting(slot, {
|
||||
...appState.serverSetting.fileUploadSettings[slot],
|
||||
@ -16,16 +21,13 @@ export const HalfPrecisionRow = () => {
|
||||
})
|
||||
}
|
||||
|
||||
|
||||
const currentVal = appState.serverSetting.fileUploadSettings[slot] ? appState.serverSetting.fileUploadSettings[slot].isHalf : true
|
||||
return (
|
||||
<div className="body-row split-3-3-4 left-padding-1 guided">
|
||||
<div className="body-item-title left-padding-2">-</div>
|
||||
<div className="body-item-text">
|
||||
<div></div>
|
||||
<input type="checkbox" checked={currentValue} onChange={() => onHalfPrecisionChanged()} /> half-precision
|
||||
</div>
|
||||
<div className="body-button-container">
|
||||
<input type="checkbox" checked={currentVal} onChange={() => onHalfPrecisionChanged()} /> half-precision
|
||||
</div>
|
||||
</div>
|
||||
)
|
||||
|
@ -27,7 +27,7 @@ export const ModelUploadButtonRow = () => {
|
||||
</div>
|
||||
<div className="body-button-container">
|
||||
<div className={uploadButtonClassName} onClick={uploadButtonAction}>{uploadButtonLabel}</div>
|
||||
<div>{uploadedText}</div>
|
||||
<div className="body-item-text-em" >{uploadedText}</div>
|
||||
</div>
|
||||
</div>
|
||||
|
||||
|
@ -8,6 +8,10 @@ export const DefaultTuneRow = () => {
|
||||
const defaultTuneRow = useMemo(() => {
|
||||
const slot = guiState.modelSlotNum
|
||||
const fileUploadSetting = appState.serverSetting.fileUploadSettings[slot]
|
||||
if (!fileUploadSetting) {
|
||||
return <></>
|
||||
}
|
||||
const currentValue = fileUploadSetting.defaultTune
|
||||
|
||||
const onDefaultTuneChanged = (val: number) => {
|
||||
appState.serverSetting.setFileUploadSetting(slot, {
|
||||
@ -20,10 +24,10 @@ export const DefaultTuneRow = () => {
|
||||
<div className="body-row split-3-2-1-4 left-padding-1 guided">
|
||||
<div className="body-item-title left-padding-2 ">Default Tune</div>
|
||||
<div>
|
||||
<input type="range" className="body-item-input-slider" min="-50" max="50" step="1" value={fileUploadSetting?.defaultTune || 0} onChange={(e) => {
|
||||
<input type="range" className="body-item-input-slider" min="-50" max="50" step="1" value={currentValue} onChange={(e) => {
|
||||
onDefaultTuneChanged(Number(e.target.value))
|
||||
}}></input>
|
||||
<span className="body-item-input-slider-val">{fileUploadSetting?.defaultTune || 0}</span>
|
||||
<span className="body-item-input-slider-val">{currentValue}</span>
|
||||
</div>
|
||||
<div>
|
||||
</div>
|
||||
|
@ -0,0 +1,42 @@
|
||||
import { Framework } from "@dannadori/voice-changer-client-js"
|
||||
import React, { useMemo } from "react"
|
||||
import { useAppState } from "../../../001_provider/001_AppStateProvider"
|
||||
import { useGuiState } from "../001_GuiStateProvider"
|
||||
|
||||
export const FrameworkSelectorRow = () => {
|
||||
const appState = useAppState()
|
||||
const guiState = useGuiState()
|
||||
const frameworkSelectorRow = useMemo(() => {
|
||||
const slot = guiState.modelSlotNum
|
||||
const fileUploadSetting = appState.serverSetting.fileUploadSettings[slot]
|
||||
const currentValue = fileUploadSetting?.framework || Framework.PyTorch
|
||||
|
||||
const onFrameworkChanged = (val: Framework) => {
|
||||
appState.serverSetting.setFileUploadSetting(slot, {
|
||||
...appState.serverSetting.fileUploadSettings[slot],
|
||||
framework: val
|
||||
})
|
||||
}
|
||||
return (
|
||||
<div className="body-row split-3-7 left-padding-1 guided">
|
||||
<div className="body-item-title left-padding-2">Framework</div>
|
||||
<div className="body-input-container">
|
||||
<div className="body-select-container">
|
||||
<select className="body-select" value={currentValue} onChange={(e) => {
|
||||
onFrameworkChanged(e.target.value as Framework)
|
||||
}}>
|
||||
{
|
||||
Object.values(Framework).map(x => {
|
||||
return <option key={x} value={x}>{x}</option>
|
||||
})
|
||||
}
|
||||
</select>
|
||||
</div>
|
||||
|
||||
</div>
|
||||
</div>
|
||||
)
|
||||
}, [appState.serverSetting.fileUploadSettings, appState.serverSetting.setFileUploadSetting, guiState.modelSlotNum])
|
||||
|
||||
return frameworkSelectorRow
|
||||
}
|
@ -1,6 +1,7 @@
|
||||
import React, { useMemo, useEffect } from "react"
|
||||
import { useGuiState } from "../001_GuiStateProvider"
|
||||
import { ConfigSelectRow } from "./301-1_ConfigSelectRow"
|
||||
import { ModelSelectRow } from "./301-2-5_ModelSelectRow"
|
||||
import { ONNXSelectRow } from "./301-2_ONNXSelectRow"
|
||||
import { PyTorchSelectRow } from "./301-3_PyTorchSelectRow"
|
||||
import { CorrespondenceSelectRow } from "./301-4_CorrespondenceSelectRow"
|
||||
@ -11,9 +12,11 @@ import { HalfPrecisionRow } from "./301-8_HalfPrescisionRow"
|
||||
import { ModelUploadButtonRow } from "./301-9_ModelUploadButtonRow"
|
||||
import { ModelSlotRow } from "./301-a_ModelSlotRow"
|
||||
import { DefaultTuneRow } from "./301-c_DefaultTuneRow"
|
||||
import { FrameworkSelectorRow } from "./301-d_FrameworkSelector"
|
||||
|
||||
export type ModelUploaderRowProps = {
|
||||
showModelSlot: boolean
|
||||
showFrameworkSelector: boolean
|
||||
showConfig: boolean
|
||||
showOnnx: boolean
|
||||
showPyTorch: boolean
|
||||
@ -26,7 +29,10 @@ export type ModelUploaderRowProps = {
|
||||
showDescription: boolean
|
||||
showDefaultTune: boolean
|
||||
|
||||
showPyTorchEnableCheckBox: boolean
|
||||
defaultEnablePyTorch: boolean
|
||||
onlySelectedFramework: boolean
|
||||
oneModelFileType: boolean
|
||||
|
||||
showOnnxExportButton: boolean
|
||||
}
|
||||
@ -38,6 +44,15 @@ export const ModelUploaderRow = (props: ModelUploaderRowProps) => {
|
||||
}, [])
|
||||
|
||||
const modelUploaderRow = useMemo(() => {
|
||||
const pytorchEnableCheckBox = props.showPyTorchEnableCheckBox ?
|
||||
<div>
|
||||
<input type="checkbox" checked={guiState.showPyTorchModelUpload} onChange={(e) => {
|
||||
guiState.setShowPyTorchModelUpload(e.target.checked)
|
||||
}} /> enable PyTorch
|
||||
</div>
|
||||
:
|
||||
<></>
|
||||
|
||||
return (
|
||||
<>
|
||||
<div className="body-row split-3-3-4 left-padding-1 guided">
|
||||
@ -46,17 +61,17 @@ export const ModelUploaderRow = (props: ModelUploaderRowProps) => {
|
||||
<div></div>
|
||||
</div>
|
||||
<div className="body-item-text">
|
||||
<div>
|
||||
<input type="checkbox" checked={guiState.showPyTorchModelUpload} onChange={(e) => {
|
||||
guiState.setShowPyTorchModelUpload(e.target.checked)
|
||||
}} /> enable PyTorch
|
||||
{pytorchEnableCheckBox}
|
||||
</div>
|
||||
</div>
|
||||
</div>
|
||||
<ModelSlotRow />
|
||||
{props.showModelSlot ? <ModelSlotRow /> : <></>}
|
||||
{props.showFrameworkSelector ? <FrameworkSelectorRow /> : <></>}
|
||||
{props.showConfig ? <ConfigSelectRow /> : <></>}
|
||||
{props.showOnnx ? <ONNXSelectRow /> : <></>}
|
||||
{props.showPyTorch && guiState.showPyTorchModelUpload ? <PyTorchSelectRow /> : <></>}
|
||||
|
||||
{props.oneModelFileType ? <ModelSelectRow /> : <></>}
|
||||
{props.showOnnx ? <ONNXSelectRow onlyWhenSelected={props.onlySelectedFramework} /> : <></>}
|
||||
{props.showPyTorch ? <PyTorchSelectRow onlyWhenSelected={props.onlySelectedFramework} /> : <></>}
|
||||
|
||||
{props.showCorrespondence ? <CorrespondenceSelectRow /> : <></>}
|
||||
{props.showPyTorchCluster ? <PyTorchClusterSelectRow /> : <></>}
|
||||
{props.showFeature ? <FeatureSelectRow /> : <></>}
|
||||
|
@ -1,5 +1,5 @@
|
||||
import React, { useMemo } from "react"
|
||||
import { fileSelector, ModelSamplingRate } from "@dannadori/voice-changer-client-js"
|
||||
import { ModelSamplingRate } from "@dannadori/voice-changer-client-js"
|
||||
import { useAppState } from "../../../001_provider/001_AppStateProvider"
|
||||
|
||||
export type ModelSamplingRateRowProps = {
|
||||
|
@ -535,6 +535,14 @@ body {
|
||||
color: rgb(30, 30, 30);
|
||||
font-size: 0.7rem;
|
||||
}
|
||||
.body-item-text-em {
|
||||
color: rgb(250, 30, 30);
|
||||
overflow: hidden;
|
||||
text-overflow: ellipsis;
|
||||
white-space: nowrap;
|
||||
font-weight: 700;
|
||||
}
|
||||
|
||||
.body-input-container {
|
||||
display: flex;
|
||||
}
|
||||
|
95
client/lib/package-lock.json
generated
95
client/lib/package-lock.json
generated
@ -1,12 +1,12 @@
|
||||
{
|
||||
"name": "@dannadori/voice-changer-client-js",
|
||||
"version": "1.0.114",
|
||||
"version": "1.0.115",
|
||||
"lockfileVersion": 2,
|
||||
"requires": true,
|
||||
"packages": {
|
||||
"": {
|
||||
"name": "@dannadori/voice-changer-client-js",
|
||||
"version": "1.0.114",
|
||||
"version": "1.0.115",
|
||||
"license": "ISC",
|
||||
"dependencies": {
|
||||
"@types/readable-stream": "^2.3.15",
|
||||
@ -18,17 +18,17 @@
|
||||
"socket.io-client": "^4.6.1"
|
||||
},
|
||||
"devDependencies": {
|
||||
"@types/audioworklet": "^0.0.41",
|
||||
"@types/node": "^18.15.13",
|
||||
"@types/audioworklet": "^0.0.42",
|
||||
"@types/node": "^18.16.0",
|
||||
"@types/react": "18.0.38",
|
||||
"@types/react-dom": "18.0.11",
|
||||
"eslint": "^8.38.0",
|
||||
"eslint": "^8.39.0",
|
||||
"eslint-config-prettier": "^8.8.0",
|
||||
"eslint-plugin-prettier": "^4.2.1",
|
||||
"eslint-plugin-react": "^7.32.2",
|
||||
"eslint-webpack-plugin": "^4.0.1",
|
||||
"npm-run-all": "^4.1.5",
|
||||
"prettier": "^2.8.7",
|
||||
"prettier": "^2.8.8",
|
||||
"raw-loader": "^4.0.2",
|
||||
"rimraf": "^5.0.0",
|
||||
"ts-loader": "^9.4.2",
|
||||
@ -1451,9 +1451,9 @@
|
||||
}
|
||||
},
|
||||
"node_modules/@eslint/js": {
|
||||
"version": "8.38.0",
|
||||
"resolved": "https://registry.npmjs.org/@eslint/js/-/js-8.38.0.tgz",
|
||||
"integrity": "sha512-IoD2MfUnOV58ghIHCiil01PcohxjbYR/qCxsoC+xNgUwh1EY8jOOrYmu3d3a71+tJJ23uscEV4X2HJWMsPJu4g==",
|
||||
"version": "8.39.0",
|
||||
"resolved": "https://registry.npmjs.org/@eslint/js/-/js-8.39.0.tgz",
|
||||
"integrity": "sha512-kf9RB0Fg7NZfap83B3QOqOGg9QmD9yBudqQXzzOtn3i4y7ZUXe5ONeW34Gwi+TxhH4mvj72R1Zc300KUMa9Bng==",
|
||||
"dev": true,
|
||||
"engines": {
|
||||
"node": "^12.22.0 || ^14.17.0 || >=16.0.0"
|
||||
@ -1686,9 +1686,9 @@
|
||||
"integrity": "sha512-+9jVqKhRSpsc591z5vX+X5Yyw+he/HCB4iQ/RYxw35CEPaY1gnsNE43nf9n9AaYjAQrTiI/mOwKUKdUs9vf7Xg=="
|
||||
},
|
||||
"node_modules/@types/audioworklet": {
|
||||
"version": "0.0.41",
|
||||
"resolved": "https://registry.npmjs.org/@types/audioworklet/-/audioworklet-0.0.41.tgz",
|
||||
"integrity": "sha512-8BWffzGoSRz436IviQVPye75YYWfac4OKdcLgkZxb3APZxSmAOp2SMtsH1yuM1x57/z/J7bsm05Yq98Hzk1t/w==",
|
||||
"version": "0.0.42",
|
||||
"resolved": "https://registry.npmjs.org/@types/audioworklet/-/audioworklet-0.0.42.tgz",
|
||||
"integrity": "sha512-vUHhMkam6BjeomsxZc2f7g0d4fI7PV5EnAoaHo83iy4hNlYphgBgRbcWRK0UEY7jUgfY46kCLYO1riZUdH/P+g==",
|
||||
"dev": true
|
||||
},
|
||||
"node_modules/@types/body-parser": {
|
||||
@ -1829,9 +1829,9 @@
|
||||
"dev": true
|
||||
},
|
||||
"node_modules/@types/node": {
|
||||
"version": "18.15.13",
|
||||
"resolved": "https://registry.npmjs.org/@types/node/-/node-18.15.13.tgz",
|
||||
"integrity": "sha512-N+0kuo9KgrUQ1Sn/ifDXsvg0TTleP7rIy4zOBGECxAljqvqfqpTfzx0Q1NUedOixRMBfe2Whhb056a42cWs26Q=="
|
||||
"version": "18.16.0",
|
||||
"resolved": "https://registry.npmjs.org/@types/node/-/node-18.16.0.tgz",
|
||||
"integrity": "sha512-BsAaKhB+7X+H4GnSjGhJG9Qi8Tw+inU9nJDwmD5CgOmBLEI6ArdhikpLX7DjbjDRDTbqZzU2LSQNZg8WGPiSZQ=="
|
||||
},
|
||||
"node_modules/@types/prop-types": {
|
||||
"version": "15.7.5",
|
||||
@ -3230,15 +3230,15 @@
|
||||
}
|
||||
},
|
||||
"node_modules/eslint": {
|
||||
"version": "8.38.0",
|
||||
"resolved": "https://registry.npmjs.org/eslint/-/eslint-8.38.0.tgz",
|
||||
"integrity": "sha512-pIdsD2jwlUGf/U38Jv97t8lq6HpaU/G9NKbYmpWpZGw3LdTNhZLbJePqxOXGB5+JEKfOPU/XLxYxFh03nr1KTg==",
|
||||
"version": "8.39.0",
|
||||
"resolved": "https://registry.npmjs.org/eslint/-/eslint-8.39.0.tgz",
|
||||
"integrity": "sha512-mwiok6cy7KTW7rBpo05k6+p4YVZByLNjAZ/ACB9DRCu4YDRwjXI01tWHp6KAUWelsBetTxKK/2sHB0vdS8Z2Og==",
|
||||
"dev": true,
|
||||
"dependencies": {
|
||||
"@eslint-community/eslint-utils": "^4.2.0",
|
||||
"@eslint-community/regexpp": "^4.4.0",
|
||||
"@eslint/eslintrc": "^2.0.2",
|
||||
"@eslint/js": "8.38.0",
|
||||
"@eslint/js": "8.39.0",
|
||||
"@humanwhocodes/config-array": "^0.11.8",
|
||||
"@humanwhocodes/module-importer": "^1.0.1",
|
||||
"@nodelib/fs.walk": "^1.2.8",
|
||||
@ -3248,7 +3248,7 @@
|
||||
"debug": "^4.3.2",
|
||||
"doctrine": "^3.0.0",
|
||||
"escape-string-regexp": "^4.0.0",
|
||||
"eslint-scope": "^7.1.1",
|
||||
"eslint-scope": "^7.2.0",
|
||||
"eslint-visitor-keys": "^3.4.0",
|
||||
"espree": "^9.5.1",
|
||||
"esquery": "^1.4.2",
|
||||
@ -3361,9 +3361,9 @@
|
||||
}
|
||||
},
|
||||
"node_modules/eslint-scope": {
|
||||
"version": "7.1.1",
|
||||
"resolved": "https://registry.npmjs.org/eslint-scope/-/eslint-scope-7.1.1.tgz",
|
||||
"integrity": "sha512-QKQM/UXpIiHcLqJ5AOyIW7XZmzjkzQXYE54n1++wb0u9V/abW3l9uQnxX8Z5Xd18xyKIMTUAyQ0k1e8pz6LUrw==",
|
||||
"version": "7.2.0",
|
||||
"resolved": "https://registry.npmjs.org/eslint-scope/-/eslint-scope-7.2.0.tgz",
|
||||
"integrity": "sha512-DYj5deGlHBfMt15J7rdtyKNq/Nqlv5KfU4iodrQ019XESsRnwXH9KAE0y3cwtUHDo2ob7CypAnCqefh6vioWRw==",
|
||||
"dev": true,
|
||||
"dependencies": {
|
||||
"esrecurse": "^4.3.0",
|
||||
@ -3371,6 +3371,9 @@
|
||||
},
|
||||
"engines": {
|
||||
"node": "^12.22.0 || ^14.17.0 || >=16.0.0"
|
||||
},
|
||||
"funding": {
|
||||
"url": "https://opencollective.com/eslint"
|
||||
}
|
||||
},
|
||||
"node_modules/eslint-visitor-keys": {
|
||||
@ -5843,9 +5846,9 @@
|
||||
}
|
||||
},
|
||||
"node_modules/prettier": {
|
||||
"version": "2.8.7",
|
||||
"resolved": "https://registry.npmjs.org/prettier/-/prettier-2.8.7.tgz",
|
||||
"integrity": "sha512-yPngTo3aXUUmyuTjeTUT75txrf+aMh9FiD7q9ZE/i6r0bPb22g4FsE6Y338PQX1bmfy08i9QQCB7/rcUAVntfw==",
|
||||
"version": "2.8.8",
|
||||
"resolved": "https://registry.npmjs.org/prettier/-/prettier-2.8.8.tgz",
|
||||
"integrity": "sha512-tdN8qQGvNjw4CHbY+XXk0JgCXn9QiF21a55rBe5LJAU+kDyC4WQn4+awm2Xfk2lQMk5fKup9XgzTZtGkjBdP9Q==",
|
||||
"dev": true,
|
||||
"bin": {
|
||||
"prettier": "bin-prettier.js"
|
||||
@ -9132,9 +9135,9 @@
|
||||
}
|
||||
},
|
||||
"@eslint/js": {
|
||||
"version": "8.38.0",
|
||||
"resolved": "https://registry.npmjs.org/@eslint/js/-/js-8.38.0.tgz",
|
||||
"integrity": "sha512-IoD2MfUnOV58ghIHCiil01PcohxjbYR/qCxsoC+xNgUwh1EY8jOOrYmu3d3a71+tJJ23uscEV4X2HJWMsPJu4g==",
|
||||
"version": "8.39.0",
|
||||
"resolved": "https://registry.npmjs.org/@eslint/js/-/js-8.39.0.tgz",
|
||||
"integrity": "sha512-kf9RB0Fg7NZfap83B3QOqOGg9QmD9yBudqQXzzOtn3i4y7ZUXe5ONeW34Gwi+TxhH4mvj72R1Zc300KUMa9Bng==",
|
||||
"dev": true
|
||||
},
|
||||
"@humanwhocodes/config-array": {
|
||||
@ -9330,9 +9333,9 @@
|
||||
"integrity": "sha512-+9jVqKhRSpsc591z5vX+X5Yyw+he/HCB4iQ/RYxw35CEPaY1gnsNE43nf9n9AaYjAQrTiI/mOwKUKdUs9vf7Xg=="
|
||||
},
|
||||
"@types/audioworklet": {
|
||||
"version": "0.0.41",
|
||||
"resolved": "https://registry.npmjs.org/@types/audioworklet/-/audioworklet-0.0.41.tgz",
|
||||
"integrity": "sha512-8BWffzGoSRz436IviQVPye75YYWfac4OKdcLgkZxb3APZxSmAOp2SMtsH1yuM1x57/z/J7bsm05Yq98Hzk1t/w==",
|
||||
"version": "0.0.42",
|
||||
"resolved": "https://registry.npmjs.org/@types/audioworklet/-/audioworklet-0.0.42.tgz",
|
||||
"integrity": "sha512-vUHhMkam6BjeomsxZc2f7g0d4fI7PV5EnAoaHo83iy4hNlYphgBgRbcWRK0UEY7jUgfY46kCLYO1riZUdH/P+g==",
|
||||
"dev": true
|
||||
},
|
||||
"@types/body-parser": {
|
||||
@ -9473,9 +9476,9 @@
|
||||
"dev": true
|
||||
},
|
||||
"@types/node": {
|
||||
"version": "18.15.13",
|
||||
"resolved": "https://registry.npmjs.org/@types/node/-/node-18.15.13.tgz",
|
||||
"integrity": "sha512-N+0kuo9KgrUQ1Sn/ifDXsvg0TTleP7rIy4zOBGECxAljqvqfqpTfzx0Q1NUedOixRMBfe2Whhb056a42cWs26Q=="
|
||||
"version": "18.16.0",
|
||||
"resolved": "https://registry.npmjs.org/@types/node/-/node-18.16.0.tgz",
|
||||
"integrity": "sha512-BsAaKhB+7X+H4GnSjGhJG9Qi8Tw+inU9nJDwmD5CgOmBLEI6ArdhikpLX7DjbjDRDTbqZzU2LSQNZg8WGPiSZQ=="
|
||||
},
|
||||
"@types/prop-types": {
|
||||
"version": "15.7.5",
|
||||
@ -10563,15 +10566,15 @@
|
||||
"dev": true
|
||||
},
|
||||
"eslint": {
|
||||
"version": "8.38.0",
|
||||
"resolved": "https://registry.npmjs.org/eslint/-/eslint-8.38.0.tgz",
|
||||
"integrity": "sha512-pIdsD2jwlUGf/U38Jv97t8lq6HpaU/G9NKbYmpWpZGw3LdTNhZLbJePqxOXGB5+JEKfOPU/XLxYxFh03nr1KTg==",
|
||||
"version": "8.39.0",
|
||||
"resolved": "https://registry.npmjs.org/eslint/-/eslint-8.39.0.tgz",
|
||||
"integrity": "sha512-mwiok6cy7KTW7rBpo05k6+p4YVZByLNjAZ/ACB9DRCu4YDRwjXI01tWHp6KAUWelsBetTxKK/2sHB0vdS8Z2Og==",
|
||||
"dev": true,
|
||||
"requires": {
|
||||
"@eslint-community/eslint-utils": "^4.2.0",
|
||||
"@eslint-community/regexpp": "^4.4.0",
|
||||
"@eslint/eslintrc": "^2.0.2",
|
||||
"@eslint/js": "8.38.0",
|
||||
"@eslint/js": "8.39.0",
|
||||
"@humanwhocodes/config-array": "^0.11.8",
|
||||
"@humanwhocodes/module-importer": "^1.0.1",
|
||||
"@nodelib/fs.walk": "^1.2.8",
|
||||
@ -10581,7 +10584,7 @@
|
||||
"debug": "^4.3.2",
|
||||
"doctrine": "^3.0.0",
|
||||
"escape-string-regexp": "^4.0.0",
|
||||
"eslint-scope": "^7.1.1",
|
||||
"eslint-scope": "^7.2.0",
|
||||
"eslint-visitor-keys": "^3.4.0",
|
||||
"espree": "^9.5.1",
|
||||
"esquery": "^1.4.2",
|
||||
@ -10661,9 +10664,9 @@
|
||||
}
|
||||
},
|
||||
"eslint-scope": {
|
||||
"version": "7.1.1",
|
||||
"resolved": "https://registry.npmjs.org/eslint-scope/-/eslint-scope-7.1.1.tgz",
|
||||
"integrity": "sha512-QKQM/UXpIiHcLqJ5AOyIW7XZmzjkzQXYE54n1++wb0u9V/abW3l9uQnxX8Z5Xd18xyKIMTUAyQ0k1e8pz6LUrw==",
|
||||
"version": "7.2.0",
|
||||
"resolved": "https://registry.npmjs.org/eslint-scope/-/eslint-scope-7.2.0.tgz",
|
||||
"integrity": "sha512-DYj5deGlHBfMt15J7rdtyKNq/Nqlv5KfU4iodrQ019XESsRnwXH9KAE0y3cwtUHDo2ob7CypAnCqefh6vioWRw==",
|
||||
"dev": true,
|
||||
"requires": {
|
||||
"esrecurse": "^4.3.0",
|
||||
@ -12477,9 +12480,9 @@
|
||||
"dev": true
|
||||
},
|
||||
"prettier": {
|
||||
"version": "2.8.7",
|
||||
"resolved": "https://registry.npmjs.org/prettier/-/prettier-2.8.7.tgz",
|
||||
"integrity": "sha512-yPngTo3aXUUmyuTjeTUT75txrf+aMh9FiD7q9ZE/i6r0bPb22g4FsE6Y338PQX1bmfy08i9QQCB7/rcUAVntfw==",
|
||||
"version": "2.8.8",
|
||||
"resolved": "https://registry.npmjs.org/prettier/-/prettier-2.8.8.tgz",
|
||||
"integrity": "sha512-tdN8qQGvNjw4CHbY+XXk0JgCXn9QiF21a55rBe5LJAU+kDyC4WQn4+awm2Xfk2lQMk5fKup9XgzTZtGkjBdP9Q==",
|
||||
"dev": true
|
||||
},
|
||||
"prettier-linter-helpers": {
|
||||
|
@ -1,6 +1,6 @@
|
||||
{
|
||||
"name": "@dannadori/voice-changer-client-js",
|
||||
"version": "1.0.114",
|
||||
"version": "1.0.115",
|
||||
"description": "",
|
||||
"main": "dist/index.js",
|
||||
"directories": {
|
||||
@ -26,17 +26,17 @@
|
||||
"author": "wataru.okada@flect.co.jp",
|
||||
"license": "ISC",
|
||||
"devDependencies": {
|
||||
"@types/audioworklet": "^0.0.41",
|
||||
"@types/node": "^18.15.13",
|
||||
"@types/audioworklet": "^0.0.42",
|
||||
"@types/node": "^18.16.0",
|
||||
"@types/react": "18.0.38",
|
||||
"@types/react-dom": "18.0.11",
|
||||
"eslint": "^8.38.0",
|
||||
"eslint": "^8.39.0",
|
||||
"eslint-config-prettier": "^8.8.0",
|
||||
"eslint-plugin-prettier": "^4.2.1",
|
||||
"eslint-plugin-react": "^7.32.2",
|
||||
"eslint-webpack-plugin": "^4.0.1",
|
||||
"npm-run-all": "^4.1.5",
|
||||
"prettier": "^2.8.7",
|
||||
"prettier": "^2.8.8",
|
||||
"raw-loader": "^4.0.2",
|
||||
"rimraf": "^5.0.0",
|
||||
"ts-loader": "^9.4.2",
|
||||
|
@ -110,6 +110,10 @@ export class ServerConfigurator {
|
||||
}
|
||||
|
||||
loadModel = async (slot: number, configFilename: string, pyTorchModelFilename: string | null, onnxModelFilename: string | null, clusterTorchModelFilename: string | null, featureFilename: string | null, indexFilename: string | null, isHalf: boolean, params: string = "{}") => {
|
||||
if (isHalf == undefined || isHalf == null) {
|
||||
console.warn("isHalf is invalid value", isHalf)
|
||||
isHalf = false
|
||||
}
|
||||
const url = this.serverUrl + "/load_model"
|
||||
const info = new Promise<ServerInfo>(async (resolve) => {
|
||||
const formData = new FormData();
|
||||
|
@ -138,13 +138,28 @@ export type VoiceChangerServerSetting = {
|
||||
inputSampleRate: InputSampleRate
|
||||
}
|
||||
|
||||
type ModelSlot = {
|
||||
onnxModelFile: string,
|
||||
pyTorchModelFile: string
|
||||
featureFile: string,
|
||||
indexFile: string,
|
||||
|
||||
defaultTrans: number,
|
||||
|
||||
modelType: number,
|
||||
embChannels: number,
|
||||
f0: boolean,
|
||||
samplingRate: number
|
||||
deprecated: boolean
|
||||
}
|
||||
|
||||
export type ServerInfo = VoiceChangerServerSetting & {
|
||||
status: string
|
||||
configFile: string,
|
||||
pyTorchModelFile: string,
|
||||
onnxModelFile: string,
|
||||
onnxExecutionProviders: OnnxExecutionProvider[]
|
||||
modelSlots: any[]
|
||||
modelSlots: ModelSlot[]
|
||||
}
|
||||
|
||||
export type ServerInfoSoVitsSVC = ServerInfo & {
|
||||
|
@ -1,5 +1,5 @@
|
||||
import { useState, useMemo, useEffect } from "react"
|
||||
import { VoiceChangerServerSetting, ServerInfo, ServerSettingKey, INDEXEDDB_KEY_SERVER, INDEXEDDB_KEY_MODEL_DATA, ClientType, DefaultServerSetting_MMVCv13, DefaultServerSetting_MMVCv15, DefaultServerSetting_so_vits_svc_40v2, DefaultServerSetting_so_vits_svc_40, DefaultServerSetting_so_vits_svc_40_c, DefaultServerSetting_RVC, OnnxExporterInfo, DefaultServerSetting_DDSP_SVC, MAX_MODEL_SLOT_NUM } from "../const"
|
||||
import { VoiceChangerServerSetting, ServerInfo, ServerSettingKey, INDEXEDDB_KEY_SERVER, INDEXEDDB_KEY_MODEL_DATA, ClientType, DefaultServerSetting_MMVCv13, DefaultServerSetting_MMVCv15, DefaultServerSetting_so_vits_svc_40v2, DefaultServerSetting_so_vits_svc_40, DefaultServerSetting_so_vits_svc_40_c, DefaultServerSetting_RVC, OnnxExporterInfo, DefaultServerSetting_DDSP_SVC, MAX_MODEL_SLOT_NUM, Framework } from "../const"
|
||||
import { VoiceChangerClient } from "../VoiceChangerClient"
|
||||
import { useIndexedDB } from "./useIndexedDB"
|
||||
|
||||
@ -22,6 +22,7 @@ export type FileUploadSetting = {
|
||||
isHalf: boolean
|
||||
uploaded: boolean
|
||||
defaultTune: number
|
||||
framework: Framework
|
||||
params: string
|
||||
|
||||
}
|
||||
@ -38,6 +39,7 @@ const InitialFileUploadSetting: FileUploadSetting = {
|
||||
isHalf: true,
|
||||
uploaded: false,
|
||||
defaultTune: 0,
|
||||
framework: Framework.PyTorch,
|
||||
params: "{}"
|
||||
}
|
||||
|
||||
@ -267,8 +269,11 @@ export const useServerSetting = (props: UseServerSettingProps): ServerSettingSta
|
||||
|
||||
const configFileName = fileUploadSetting.configFile ? fileUploadSetting.configFile.filename || "-" : "-"
|
||||
const params = JSON.stringify({
|
||||
trans: fileUploadSetting.defaultTune
|
||||
trans: fileUploadSetting.defaultTune || 0
|
||||
})
|
||||
if (fileUploadSetting.isHalf == undefined) {
|
||||
fileUploadSetting.isHalf = false
|
||||
}
|
||||
const loadPromise = props.voiceChangerClient.loadModel(
|
||||
slot,
|
||||
configFileName,
|
||||
@ -279,7 +284,6 @@ export const useServerSetting = (props: UseServerSettingProps): ServerSettingSta
|
||||
fileUploadSetting.index?.filename || null,
|
||||
fileUploadSetting.isHalf,
|
||||
params,
|
||||
|
||||
)
|
||||
|
||||
// サーバでロード中にキャッシュにセーブ
|
||||
@ -322,6 +326,7 @@ export const useServerSetting = (props: UseServerSettingProps): ServerSettingSta
|
||||
isHalf: fileUploadSetting.isHalf, // キャッシュとしては不使用。guiで上書きされる。
|
||||
uploaded: false, // キャッシュから読み込まれるときには、まだuploadされていないから。
|
||||
defaultTune: fileUploadSetting.defaultTune,
|
||||
framework: fileUploadSetting.framework,
|
||||
params: fileUploadSetting.params
|
||||
}
|
||||
setItem(`${INDEXEDDB_KEY_MODEL_DATA}_${slot}`, saveData)
|
||||
|
@ -61,6 +61,9 @@ RUN pip install einops==0.6.0
|
||||
RUN pip install local_attention==1.8.5
|
||||
RUN pip install websockets==11.0.2
|
||||
|
||||
WORKDIR /
|
||||
ADD dummy /
|
||||
|
||||
RUN git clone https://github.com/w-okada/voice-changer.git
|
||||
|
||||
ADD /setup.sh /voice-changer/server
|
||||
|
@ -7,7 +7,7 @@
|
||||
"build:docker": "date +%Y%m%d%H%M%S > docker/dummy && DOCKER_BUILDKIT=1 docker build -f docker/Dockerfile docker/ -t voice-changer",
|
||||
"build:docker:onnx": "DOCKER_BUILDKIT=1 docker build -f docker_onnx/Dockerfile docker/ -t onnx-converter",
|
||||
"build:docker:trainer": "date +%Y%m%d%H%M%S > docker_trainer/dummy && DOCKER_BUILDKIT=1 docker build -f docker_trainer/Dockerfile docker_trainer/ -t trainer",
|
||||
"build:docker:vcclient": "date +%Y%m%d%H%M%S > docker/dummy && DOCKER_BUILDKIT=1 docker build -f docker_vcclient/Dockerfile docker_vcclient/ -t vcclient",
|
||||
"build:docker:vcclient": "date +%Y%m%d%H%M%S > docker_vcclient/dummy && DOCKER_BUILDKIT=1 docker build -f docker_vcclient/Dockerfile docker_vcclient/ -t vcclient",
|
||||
"push:docker": "bash script/001_pushDocker.sh",
|
||||
"push:docker:trainer": "bash script/002_pushDockerTrainer.sh",
|
||||
"push:docker:vcclient": "bash script/003_pushDockerVCClient.sh",
|
||||
|
16
server/.vscode/settings.json
vendored
Normal file
16
server/.vscode/settings.json
vendored
Normal file
@ -0,0 +1,16 @@
|
||||
{
|
||||
"workbench.colorCustomizations": {
|
||||
"tab.activeBackground": "#65952acc"
|
||||
},
|
||||
"python.formatting.provider": "black",
|
||||
"python.linting.mypyEnabled": true,
|
||||
"[python]": {
|
||||
"editor.defaultFormatter": null, // Prettier を使わないようにする
|
||||
"editor.formatOnSave": true // ファイル保存時に自動フォーマット
|
||||
},
|
||||
"flake8.args": [
|
||||
"--ignore=E501,E402,E722,E741,W503"
|
||||
// "--max-line-length=150",
|
||||
// "--max-complexity=20"
|
||||
]
|
||||
}
|
@ -1,12 +1,13 @@
|
||||
|
||||
class NoModeLoadedException(Exception):
|
||||
def __init__(self, framework):
|
||||
self.framework = framework
|
||||
|
||||
def __str__(self):
|
||||
return repr(f"No model for {self.framework} loaded. Please confirm the model uploaded.")
|
||||
return repr(
|
||||
f"No model for {self.framework} loaded. Please confirm the model uploaded."
|
||||
)
|
||||
|
||||
|
||||
class ONNXInputArgumentException(Exception):
|
||||
def __str__(self):
|
||||
return repr(f"ONNX received invalid argument.")
|
||||
return repr("ONNX received invalid argument.")
|
||||
|
@ -2,12 +2,12 @@ import sys
|
||||
|
||||
from distutils.util import strtobool
|
||||
from datetime import datetime
|
||||
from dataclasses import dataclass
|
||||
import misc.log_control
|
||||
import socket
|
||||
import platform
|
||||
import os
|
||||
import argparse
|
||||
from voice_changer.utils.VoiceChangerParams import VoiceChangerParams
|
||||
|
||||
import uvicorn
|
||||
from mods.ssl import create_self_signed_cert
|
||||
from voice_changer.VoiceChangerManager import VoiceChangerManager
|
||||
@ -16,35 +16,56 @@ from restapi.MMVC_Rest import MMVC_Rest
|
||||
from const import NATIVE_CLIENT_FILE_MAC, NATIVE_CLIENT_FILE_WIN, SSL_KEY_DIR
|
||||
import subprocess
|
||||
import multiprocessing as mp
|
||||
from misc.log_control import setup_loggers
|
||||
|
||||
setup_loggers()
|
||||
|
||||
|
||||
def setupArgParser():
|
||||
parser = argparse.ArgumentParser()
|
||||
parser.add_argument("-p", type=int, default=18888, help="port")
|
||||
parser.add_argument("--https", type=strtobool,
|
||||
default=False, help="use https")
|
||||
parser.add_argument("--httpsKey", type=str,
|
||||
default="ssl.key", help="path for the key of https")
|
||||
parser.add_argument("--httpsCert", type=str,
|
||||
default="ssl.cert", help="path for the cert of https")
|
||||
parser.add_argument("--httpsSelfSigned", type=strtobool,
|
||||
default=True, help="generate self-signed certificate")
|
||||
parser.add_argument("--https", type=strtobool, default=False, help="use https")
|
||||
parser.add_argument(
|
||||
"--httpsKey", type=str, default="ssl.key", help="path for the key of https"
|
||||
)
|
||||
parser.add_argument(
|
||||
"--httpsCert", type=str, default="ssl.cert", help="path for the cert of https"
|
||||
)
|
||||
parser.add_argument(
|
||||
"--httpsSelfSigned",
|
||||
type=strtobool,
|
||||
default=True,
|
||||
help="generate self-signed certificate",
|
||||
)
|
||||
|
||||
# parser.add_argument("--internal", type=strtobool, default=False, help="各種パスをmac appの中身に変換")
|
||||
|
||||
parser.add_argument("--content_vec_500", type=str, help="path to content_vec_500 model(pytorch)")
|
||||
parser.add_argument("--content_vec_500_onnx", type=str, help="path to content_vec_500 model(onnx)")
|
||||
parser.add_argument("--content_vec_500_onnx_on", type=strtobool, default=False, help="use or not onnx for content_vec_500")
|
||||
parser.add_argument("--hubert_base", type=str, help="path to hubert_base model(pytorch)")
|
||||
parser.add_argument("--hubert_soft", type=str, help="path to hubert_soft model(pytorch)")
|
||||
parser.add_argument("--nsf_hifigan", type=str, help="path to nsf_hifigan model(pytorch)")
|
||||
parser.add_argument(
|
||||
"--content_vec_500", type=str, help="path to content_vec_500 model(pytorch)"
|
||||
)
|
||||
parser.add_argument(
|
||||
"--content_vec_500_onnx", type=str, help="path to content_vec_500 model(onnx)"
|
||||
)
|
||||
parser.add_argument(
|
||||
"--content_vec_500_onnx_on",
|
||||
type=strtobool,
|
||||
default=False,
|
||||
help="use or not onnx for content_vec_500",
|
||||
)
|
||||
parser.add_argument(
|
||||
"--hubert_base", type=str, help="path to hubert_base model(pytorch)"
|
||||
)
|
||||
parser.add_argument(
|
||||
"--hubert_soft", type=str, help="path to hubert_soft model(pytorch)"
|
||||
)
|
||||
parser.add_argument(
|
||||
"--nsf_hifigan", type=str, help="path to nsf_hifigan model(pytorch)"
|
||||
)
|
||||
|
||||
return parser
|
||||
|
||||
|
||||
def printMessage(message, level=0):
|
||||
pf = platform.system()
|
||||
if pf == 'Windows':
|
||||
if pf == "Windows":
|
||||
if level == 0:
|
||||
print(f"{message}")
|
||||
elif level == 1:
|
||||
@ -78,37 +99,38 @@ def localServer():
|
||||
host="0.0.0.0",
|
||||
port=int(PORT),
|
||||
reload=False if hasattr(sys, "_MEIPASS") else True,
|
||||
log_level="warning"
|
||||
log_level="warning",
|
||||
)
|
||||
|
||||
|
||||
if __name__ == 'MMVCServerSIO':
|
||||
voiceChangerManager = VoiceChangerManager.get_instance({
|
||||
"content_vec_500": args.content_vec_500,
|
||||
"content_vec_500_onnx": args.content_vec_500_onnx,
|
||||
"content_vec_500_onnx_on": args.content_vec_500_onnx_on,
|
||||
"hubert_base": args.hubert_base,
|
||||
"hubert_soft": args.hubert_soft,
|
||||
"nsf_hifigan": args.nsf_hifigan,
|
||||
})
|
||||
if __name__ == "MMVCServerSIO":
|
||||
voiceChangerParams = VoiceChangerParams(
|
||||
content_vec_500=args.content_vec_500,
|
||||
content_vec_500_onnx=args.content_vec_500_onnx,
|
||||
content_vec_500_onnx_on=args.content_vec_500_onnx_on,
|
||||
hubert_base=args.hubert_base,
|
||||
hubert_soft=args.hubert_soft,
|
||||
nsf_hifigan=args.nsf_hifigan,
|
||||
)
|
||||
voiceChangerManager = VoiceChangerManager.get_instance(voiceChangerParams)
|
||||
print("voiceChangerManager", voiceChangerManager)
|
||||
|
||||
app_fastapi = MMVC_Rest.get_instance(voiceChangerManager)
|
||||
app_socketio = MMVC_SocketIOApp.get_instance(app_fastapi, voiceChangerManager)
|
||||
|
||||
|
||||
if __name__ == '__mp_main__':
|
||||
printMessage(f"サーバプロセスを起動しています。", level=2)
|
||||
if __name__ == "__mp_main__":
|
||||
printMessage("サーバプロセスを起動しています。", level=2)
|
||||
|
||||
if __name__ == '__main__':
|
||||
if __name__ == "__main__":
|
||||
mp.freeze_support()
|
||||
|
||||
printMessage(f"Voice Changerを起動しています。", level=2)
|
||||
printMessage("Voice Changerを起動しています。", level=2)
|
||||
PORT = args.p
|
||||
|
||||
if os.getenv("EX_PORT"):
|
||||
EX_PORT = os.environ["EX_PORT"]
|
||||
printMessage(
|
||||
f"External_Port:{EX_PORT} Internal_Port:{PORT}", level=1)
|
||||
printMessage(f"External_Port:{EX_PORT} Internal_Port:{PORT}", level=1)
|
||||
else:
|
||||
printMessage(f"Internal_Port:{PORT}", level=1)
|
||||
|
||||
@ -123,38 +145,42 @@ if __name__ == '__main__':
|
||||
key_base_name = f"{datetime.now().strftime('%Y%m%d_%H%M%S')}"
|
||||
keyname = f"{key_base_name}.key"
|
||||
certname = f"{key_base_name}.cert"
|
||||
create_self_signed_cert(certname, keyname, certargs={"Country": "JP",
|
||||
create_self_signed_cert(
|
||||
certname,
|
||||
keyname,
|
||||
certargs={
|
||||
"Country": "JP",
|
||||
"State": "Tokyo",
|
||||
"City": "Chuo-ku",
|
||||
"Organization": "F",
|
||||
"Org. Unit": "F"}, cert_dir=SSL_KEY_DIR)
|
||||
"Org. Unit": "F",
|
||||
},
|
||||
cert_dir=SSL_KEY_DIR,
|
||||
)
|
||||
key_path = os.path.join(SSL_KEY_DIR, keyname)
|
||||
cert_path = os.path.join(SSL_KEY_DIR, certname)
|
||||
printMessage(
|
||||
f"protocol: HTTPS(self-signed), key:{key_path}, cert:{cert_path}", level=1)
|
||||
f"protocol: HTTPS(self-signed), key:{key_path}, cert:{cert_path}", level=1
|
||||
)
|
||||
|
||||
elif args.https and args.httpsSelfSigned == 0:
|
||||
# HTTPS
|
||||
key_path = args.httpsKey
|
||||
cert_path = args.httpsCert
|
||||
printMessage(
|
||||
f"protocol: HTTPS, key:{key_path}, cert:{cert_path}", level=1)
|
||||
printMessage(f"protocol: HTTPS, key:{key_path}, cert:{cert_path}", level=1)
|
||||
else:
|
||||
# HTTP
|
||||
printMessage(f"protocol: HTTP", level=1)
|
||||
printMessage(f"-- ---- -- ", level=1)
|
||||
printMessage("protocol: HTTP", level=1)
|
||||
printMessage("-- ---- -- ", level=1)
|
||||
|
||||
# アドレス表示
|
||||
printMessage(
|
||||
f"ブラウザで次のURLを開いてください.", level=2)
|
||||
printMessage("ブラウザで次のURLを開いてください.", level=2)
|
||||
if args.https == 1:
|
||||
printMessage(
|
||||
f"https://<IP>:<PORT>/", level=1)
|
||||
printMessage("https://<IP>:<PORT>/", level=1)
|
||||
else:
|
||||
printMessage(
|
||||
f"http://<IP>:<PORT>/", level=1)
|
||||
printMessage("http://<IP>:<PORT>/", level=1)
|
||||
|
||||
printMessage(f"多くの場合は次のいずれかのURLにアクセスすると起動します。", level=2)
|
||||
printMessage("多くの場合は次のいずれかのURLにアクセスすると起動します。", level=2)
|
||||
if "EX_PORT" in locals() and "EX_IP" in locals(): # シェルスクリプト経由起動(docker)
|
||||
if args.https == 1:
|
||||
printMessage(f"https://localhost:{EX_PORT}/", level=1)
|
||||
@ -175,7 +201,7 @@ if __name__ == '__main__':
|
||||
# サーバ起動
|
||||
if args.https:
|
||||
# HTTPS サーバ起動
|
||||
res = uvicorn.run(
|
||||
uvicorn.run(
|
||||
f"{os.path.basename(__file__)[:-3]}:app_socketio",
|
||||
host="0.0.0.0",
|
||||
port=int(PORT),
|
||||
@ -188,13 +214,17 @@ if __name__ == '__main__':
|
||||
p = mp.Process(name="p", target=localServer)
|
||||
p.start()
|
||||
try:
|
||||
if sys.platform.startswith('win'):
|
||||
process = subprocess.Popen([NATIVE_CLIENT_FILE_WIN, "-u", f"http://localhost:{PORT}/"])
|
||||
if sys.platform.startswith("win"):
|
||||
process = subprocess.Popen(
|
||||
[NATIVE_CLIENT_FILE_WIN, "-u", f"http://localhost:{PORT}/"]
|
||||
)
|
||||
return_code = process.wait()
|
||||
print("client closed.")
|
||||
p.terminate()
|
||||
elif sys.platform.startswith('darwin'):
|
||||
process = subprocess.Popen([NATIVE_CLIENT_FILE_MAC, "-u", f"http://localhost:{PORT}/"])
|
||||
elif sys.platform.startswith("darwin"):
|
||||
process = subprocess.Popen(
|
||||
[NATIVE_CLIENT_FILE_MAC, "-u", f"http://localhost:{PORT}/"]
|
||||
)
|
||||
return_code = process.wait()
|
||||
print("client closed.")
|
||||
p.terminate()
|
||||
|
@ -4,7 +4,15 @@ import tempfile
|
||||
from typing import Literal, TypeAlias
|
||||
|
||||
|
||||
ModelType: TypeAlias = Literal['MMVCv15', 'MMVCv13', 'so-vits-svc-40v2', 'so-vits-svc-40', 'so-vits-svc-40_c', 'DDSP-SVC', 'RVC']
|
||||
ModelType: TypeAlias = Literal[
|
||||
"MMVCv15",
|
||||
"MMVCv13",
|
||||
"so-vits-svc-40v2",
|
||||
"so-vits-svc-40",
|
||||
"so-vits-svc-40_c",
|
||||
"DDSP-SVC",
|
||||
"RVC",
|
||||
]
|
||||
|
||||
ERROR_NO_ONNX_SESSION = "ERROR_NO_ONNX_SESSION"
|
||||
|
||||
@ -13,27 +21,45 @@ tmpdir = tempfile.TemporaryDirectory()
|
||||
# print("generate tmpdir:::",tmpdir)
|
||||
SSL_KEY_DIR = os.path.join(tmpdir.name, "keys") if hasattr(sys, "_MEIPASS") else "keys"
|
||||
MODEL_DIR = os.path.join(tmpdir.name, "logs") if hasattr(sys, "_MEIPASS") else "logs"
|
||||
UPLOAD_DIR = os.path.join(tmpdir.name, "upload_dir") if hasattr(sys, "_MEIPASS") else "upload_dir"
|
||||
NATIVE_CLIENT_FILE_WIN = os.path.join(sys._MEIPASS, "voice-changer-native-client.exe") if hasattr(sys, "_MEIPASS") else "voice-changer-native-client"
|
||||
NATIVE_CLIENT_FILE_MAC = os.path.join(sys._MEIPASS, "voice-changer-native-client.app", "Contents", "MacOS",
|
||||
"voice-changer-native-client") if hasattr(sys, "_MEIPASS") else "voice-changer-native-client"
|
||||
UPLOAD_DIR = (
|
||||
os.path.join(tmpdir.name, "upload_dir")
|
||||
if hasattr(sys, "_MEIPASS")
|
||||
else "upload_dir"
|
||||
)
|
||||
NATIVE_CLIENT_FILE_WIN = (
|
||||
os.path.join(sys._MEIPASS, "voice-changer-native-client.exe") # type: ignore
|
||||
if hasattr(sys, "_MEIPASS")
|
||||
else "voice-changer-native-client"
|
||||
)
|
||||
NATIVE_CLIENT_FILE_MAC = (
|
||||
os.path.join(
|
||||
sys._MEIPASS, # type: ignore
|
||||
"voice-changer-native-client.app",
|
||||
"Contents",
|
||||
"MacOS",
|
||||
"voice-changer-native-client",
|
||||
)
|
||||
if hasattr(sys, "_MEIPASS")
|
||||
else "voice-changer-native-client"
|
||||
)
|
||||
|
||||
HUBERT_ONNX_MODEL_PATH = os.path.join(sys._MEIPASS, "model_hubert/hubert_simple.onnx") if hasattr(sys,
|
||||
"_MEIPASS") else "model_hubert/hubert_simple.onnx"
|
||||
HUBERT_ONNX_MODEL_PATH = (
|
||||
os.path.join(sys._MEIPASS, "model_hubert/hubert_simple.onnx") # type: ignore
|
||||
if hasattr(sys, "_MEIPASS")
|
||||
else "model_hubert/hubert_simple.onnx"
|
||||
)
|
||||
|
||||
|
||||
TMP_DIR = os.path.join(tmpdir.name, "tmp_dir") if hasattr(sys, "_MEIPASS") else "tmp_dir"
|
||||
TMP_DIR = (
|
||||
os.path.join(tmpdir.name, "tmp_dir") if hasattr(sys, "_MEIPASS") else "tmp_dir"
|
||||
)
|
||||
os.makedirs(TMP_DIR, exist_ok=True)
|
||||
|
||||
|
||||
# modelType: ModelType = "MMVCv15"
|
||||
# def getModelType() -> ModelType:
|
||||
# return modelType
|
||||
# def setModelType(_modelType: ModelType):
|
||||
# global modelType
|
||||
# modelType = _modelType
|
||||
|
||||
|
||||
def getFrontendPath():
|
||||
frontend_path = os.path.join(sys._MEIPASS, "dist") if hasattr(sys, "_MEIPASS") else "../client/demo/dist"
|
||||
frontend_path = (
|
||||
os.path.join(sys._MEIPASS, "dist")
|
||||
if hasattr(sys, "_MEIPASS")
|
||||
else "../client/demo/dist"
|
||||
)
|
||||
return frontend_path
|
||||
|
@ -8,32 +8,31 @@ class UvicornSuppressFilter(logging.Filter):
|
||||
return False
|
||||
|
||||
|
||||
# logger = logging.getLogger("uvicorn.error")
|
||||
# logger.addFilter(UvicornSuppressFilter())
|
||||
def setup_loggers():
|
||||
# logger = logging.getLogger("uvicorn.error")
|
||||
# logger.addFilter(UvicornSuppressFilter())
|
||||
|
||||
logger = logging.getLogger("fairseq.tasks.hubert_pretraining")
|
||||
logger.addFilter(UvicornSuppressFilter())
|
||||
logger = logging.getLogger("fairseq.tasks.hubert_pretraining")
|
||||
logger.addFilter(UvicornSuppressFilter())
|
||||
|
||||
logger = logging.getLogger("fairseq.models.hubert.hubert")
|
||||
logger.addFilter(UvicornSuppressFilter())
|
||||
logger = logging.getLogger("fairseq.models.hubert.hubert")
|
||||
logger.addFilter(UvicornSuppressFilter())
|
||||
|
||||
logger = logging.getLogger("fairseq.tasks.text_to_speech")
|
||||
logger.addFilter(UvicornSuppressFilter())
|
||||
logger = logging.getLogger("fairseq.tasks.text_to_speech")
|
||||
logger.addFilter(UvicornSuppressFilter())
|
||||
|
||||
logger = logging.getLogger("numba.core.ssa")
|
||||
logger.addFilter(UvicornSuppressFilter())
|
||||
|
||||
logger = logging.getLogger("numba.core.ssa")
|
||||
logger.addFilter(UvicornSuppressFilter())
|
||||
logger = logging.getLogger("numba.core.interpreter")
|
||||
logger.addFilter(UvicornSuppressFilter())
|
||||
|
||||
logger = logging.getLogger("numba.core.interpreter")
|
||||
logger.addFilter(UvicornSuppressFilter())
|
||||
logger = logging.getLogger("numba.core.byteflow")
|
||||
logger.addFilter(UvicornSuppressFilter())
|
||||
|
||||
logger = logging.getLogger("numba.core.byteflow")
|
||||
logger.addFilter(UvicornSuppressFilter())
|
||||
# logger.propagate = False
|
||||
|
||||
logger = logging.getLogger("multipart.multipart")
|
||||
logger.propagate = False
|
||||
|
||||
# logger.propagate = False
|
||||
|
||||
logger = logging.getLogger("multipart.multipart")
|
||||
logger.propagate = False
|
||||
|
||||
logging.getLogger('asyncio').setLevel(logging.WARNING)
|
||||
logging.getLogger("asyncio").setLevel(logging.WARNING)
|
||||
|
@ -17,7 +17,6 @@ scipy==1.10.1
|
||||
matplotlib==3.7.1
|
||||
fairseq==0.12.2
|
||||
websockets==11.0.2
|
||||
praat-parselmouth==0.4.3
|
||||
faiss-cpu==1.7.3
|
||||
torchcrepe==0.0.18
|
||||
librosa==0.9.1
|
||||
|
@ -1,7 +1,8 @@
|
||||
from fastapi import FastAPI, Request, Response
|
||||
from fastapi import FastAPI, Request, Response, HTTPException
|
||||
from fastapi.routing import APIRoute
|
||||
from fastapi.middleware.cors import CORSMiddleware
|
||||
from fastapi.staticfiles import StaticFiles
|
||||
from fastapi.exceptions import RequestValidationError
|
||||
from typing import Callable
|
||||
from voice_changer.VoiceChangerManager import VoiceChangerManager
|
||||
|
||||
@ -18,7 +19,7 @@ class ValidationErrorLoggingRoute(APIRoute):
|
||||
async def custom_route_handler(request: Request) -> Response:
|
||||
try:
|
||||
return await original_route_handler(request)
|
||||
except Exception as exc:
|
||||
except RequestValidationError as exc:
|
||||
print("Exception", request.url, str(exc))
|
||||
body = await request.body()
|
||||
detail = {"errors": exc.errors(), "body": body.decode()}
|
||||
@ -28,10 +29,11 @@ class ValidationErrorLoggingRoute(APIRoute):
|
||||
|
||||
|
||||
class MMVC_Rest:
|
||||
_instance = None
|
||||
|
||||
@classmethod
|
||||
def get_instance(cls, voiceChangerManager: VoiceChangerManager):
|
||||
if not hasattr(cls, "_instance"):
|
||||
if cls._instance is None:
|
||||
app_fastapi = FastAPI()
|
||||
app_fastapi.router.route_class = ValidationErrorLoggingRoute
|
||||
app_fastapi.add_middleware(
|
||||
@ -43,15 +45,25 @@ class MMVC_Rest:
|
||||
)
|
||||
|
||||
app_fastapi.mount(
|
||||
"/front", StaticFiles(directory=f'{getFrontendPath()}', html=True), name="static")
|
||||
"/front",
|
||||
StaticFiles(directory=f"{getFrontendPath()}", html=True),
|
||||
name="static",
|
||||
)
|
||||
|
||||
app_fastapi.mount(
|
||||
"/trainer", StaticFiles(directory=f'{getFrontendPath()}', html=True), name="static")
|
||||
"/trainer",
|
||||
StaticFiles(directory=f"{getFrontendPath()}", html=True),
|
||||
name="static",
|
||||
)
|
||||
|
||||
app_fastapi.mount(
|
||||
"/recorder", StaticFiles(directory=f'{getFrontendPath()}', html=True), name="static")
|
||||
"/recorder",
|
||||
StaticFiles(directory=f"{getFrontendPath()}", html=True),
|
||||
name="static",
|
||||
)
|
||||
app_fastapi.mount(
|
||||
"/tmp", StaticFiles(directory=f'{TMP_DIR}'), name="static")
|
||||
"/tmp", StaticFiles(directory=f"{TMP_DIR}"), name="static"
|
||||
)
|
||||
|
||||
restHello = MMVC_Rest_Hello()
|
||||
app_fastapi.include_router(restHello.router)
|
||||
|
@ -4,12 +4,16 @@ from typing import Union
|
||||
from fastapi import APIRouter
|
||||
from fastapi.encoders import jsonable_encoder
|
||||
from fastapi.responses import JSONResponse
|
||||
from fastapi import HTTPException, FastAPI, UploadFile, File, Form
|
||||
from fastapi import UploadFile, File, Form
|
||||
|
||||
from restapi.mods.FileUploader import upload_file, concat_file_chunks
|
||||
from voice_changer.VoiceChangerManager import VoiceChangerManager
|
||||
|
||||
from const import MODEL_DIR, UPLOAD_DIR, ModelType
|
||||
from voice_changer.utils.LoadModelParams import FilePaths, LoadModelParams
|
||||
|
||||
from dataclasses import fields
|
||||
|
||||
os.makedirs(UPLOAD_DIR, exist_ok=True)
|
||||
os.makedirs(MODEL_DIR, exist_ok=True)
|
||||
|
||||
@ -19,12 +23,16 @@ class MMVC_Rest_Fileuploader:
|
||||
self.voiceChangerManager = voiceChangerManager
|
||||
self.router = APIRouter()
|
||||
self.router.add_api_route("/info", self.get_info, methods=["GET"])
|
||||
self.router.add_api_route("/upload_file", self.post_upload_file, methods=["POST"])
|
||||
self.router.add_api_route("/concat_uploaded_file", self.post_concat_uploaded_file, methods=["POST"])
|
||||
self.router.add_api_route("/update_settings", self.post_update_settings, methods=["POST"])
|
||||
self.router.add_api_route(
|
||||
"/upload_file", self.post_upload_file, methods=["POST"]
|
||||
)
|
||||
self.router.add_api_route(
|
||||
"/concat_uploaded_file", self.post_concat_uploaded_file, methods=["POST"]
|
||||
)
|
||||
self.router.add_api_route(
|
||||
"/update_settings", self.post_update_settings, methods=["POST"]
|
||||
)
|
||||
self.router.add_api_route("/load_model", self.post_load_model, methods=["POST"])
|
||||
self.router.add_api_route("/load_model_for_train", self.post_load_model_for_train, methods=["POST"])
|
||||
self.router.add_api_route("/extract_voices", self.post_extract_voices, methods=["POST"])
|
||||
self.router.add_api_route("/model_type", self.post_model_type, methods=["POST"])
|
||||
self.router.add_api_route("/model_type", self.get_model_type, methods=["GET"])
|
||||
self.router.add_api_route("/onnx", self.get_onnx, methods=["GET"])
|
||||
@ -34,9 +42,13 @@ class MMVC_Rest_Fileuploader:
|
||||
json_compatible_item_data = jsonable_encoder(res)
|
||||
return JSONResponse(content=json_compatible_item_data)
|
||||
|
||||
def post_concat_uploaded_file(self, filename: str = Form(...), filenameChunkNum: int = Form(...)):
|
||||
def post_concat_uploaded_file(
|
||||
self, filename: str = Form(...), filenameChunkNum: int = Form(...)
|
||||
):
|
||||
slot = 0
|
||||
res = concat_file_chunks(slot, UPLOAD_DIR, filename, filenameChunkNum, UPLOAD_DIR)
|
||||
res = concat_file_chunks(
|
||||
slot, UPLOAD_DIR, filename, filenameChunkNum, UPLOAD_DIR
|
||||
)
|
||||
json_compatible_item_data = jsonable_encoder(res)
|
||||
return JSONResponse(content=json_compatible_item_data)
|
||||
|
||||
@ -45,7 +57,9 @@ class MMVC_Rest_Fileuploader:
|
||||
json_compatible_item_data = jsonable_encoder(info)
|
||||
return JSONResponse(content=json_compatible_item_data)
|
||||
|
||||
def post_update_settings(self, key: str = Form(...), val: Union[int, str, float] = Form(...)):
|
||||
def post_update_settings(
|
||||
self, key: str = Form(...), val: Union[int, str, float] = Form(...)
|
||||
):
|
||||
print("post_update_settings", key, val)
|
||||
info = self.voiceChangerManager.update_settings(key, val)
|
||||
json_compatible_item_data = jsonable_encoder(info)
|
||||
@ -63,72 +77,42 @@ class MMVC_Rest_Fileuploader:
|
||||
isHalf: bool = Form(...),
|
||||
params: str = Form(...),
|
||||
):
|
||||
files = FilePaths(
|
||||
configFilename=configFilename,
|
||||
pyTorchModelFilename=pyTorchModelFilename,
|
||||
onnxModelFilename=onnxModelFilename,
|
||||
clusterTorchModelFilename=clusterTorchModelFilename,
|
||||
featureFilename=featureFilename,
|
||||
indexFilename=indexFilename,
|
||||
)
|
||||
props: LoadModelParams = LoadModelParams(
|
||||
slot=slot, isHalf=isHalf, params=params, files=files
|
||||
)
|
||||
|
||||
props = {
|
||||
"slot": slot,
|
||||
"isHalf": isHalf,
|
||||
"files": {
|
||||
"configFilename": configFilename,
|
||||
"pyTorchModelFilename": pyTorchModelFilename,
|
||||
"onnxModelFilename": onnxModelFilename,
|
||||
"clusterTorchModelFilename": clusterTorchModelFilename,
|
||||
"featureFilename": featureFilename,
|
||||
"indexFilename": indexFilename
|
||||
},
|
||||
"params": params
|
||||
}
|
||||
# Change Filepath
|
||||
for key, val in props["files"].items():
|
||||
for field in fields(props.files):
|
||||
key = field.name
|
||||
val = getattr(props.files, key)
|
||||
if val != "-":
|
||||
uploadPath = os.path.join(UPLOAD_DIR, val)
|
||||
storeDir = os.path.join(UPLOAD_DIR, f"{slot}")
|
||||
os.makedirs(storeDir, exist_ok=True)
|
||||
storePath = os.path.join(storeDir, val)
|
||||
shutil.move(uploadPath, storePath)
|
||||
props["files"][key] = storePath
|
||||
setattr(props.files, key, storePath)
|
||||
else:
|
||||
props["files"][key] = None
|
||||
# print("---------------------------------------------------2>", props)
|
||||
setattr(props.files, key, None)
|
||||
|
||||
info = self.voiceChangerManager.loadModel(props)
|
||||
json_compatible_item_data = jsonable_encoder(info)
|
||||
return JSONResponse(content=json_compatible_item_data)
|
||||
# return {"load": f"{configFilePath}, {pyTorchModelFilePath}, {onnxModelFilePath}"}
|
||||
|
||||
def post_load_model_for_train(
|
||||
self,
|
||||
modelGFilename: str = Form(...),
|
||||
modelGFilenameChunkNum: int = Form(...),
|
||||
modelDFilename: str = Form(...),
|
||||
modelDFilenameChunkNum: int = Form(...),
|
||||
):
|
||||
modelGFilePath = concat_file_chunks(
|
||||
UPLOAD_DIR, modelGFilename, modelGFilenameChunkNum, MODEL_DIR)
|
||||
modelDFilePath = concat_file_chunks(
|
||||
UPLOAD_DIR, modelDFilename, modelDFilenameChunkNum, MODEL_DIR)
|
||||
return {"File saved": f"{modelGFilePath}, {modelDFilePath}"}
|
||||
|
||||
def post_extract_voices(
|
||||
self,
|
||||
zipFilename: str = Form(...),
|
||||
zipFileChunkNum: int = Form(...),
|
||||
):
|
||||
zipFilePath = concat_file_chunks(
|
||||
UPLOAD_DIR, zipFilename, zipFileChunkNum, UPLOAD_DIR)
|
||||
shutil.unpack_archive(zipFilePath, "MMVC_Trainer/dataset/textful/")
|
||||
return {"Zip file unpacked": f"{zipFilePath}"}
|
||||
|
||||
def post_model_type(
|
||||
self,
|
||||
modelType: ModelType = Form(...),
|
||||
):
|
||||
def post_model_type(self, modelType: ModelType = Form(...)):
|
||||
info = self.voiceChangerManager.switchModelType(modelType)
|
||||
json_compatible_item_data = jsonable_encoder(info)
|
||||
return JSONResponse(content=json_compatible_item_data)
|
||||
|
||||
def get_model_type(
|
||||
self,
|
||||
):
|
||||
def get_model_type(self):
|
||||
info = self.voiceChangerManager.getModelType()
|
||||
json_compatible_item_data = jsonable_encoder(info)
|
||||
return JSONResponse(content=json_compatible_item_data)
|
||||
|
@ -1,6 +1,6 @@
|
||||
from fastapi import APIRouter
|
||||
from fastapi.encoders import jsonable_encoder
|
||||
from fastapi.responses import JSONResponse
|
||||
|
||||
|
||||
class MMVC_Rest_Hello:
|
||||
def __init__(self):
|
||||
self.router = APIRouter()
|
||||
@ -8,6 +8,3 @@ class MMVC_Rest_Hello:
|
||||
|
||||
def hello(self):
|
||||
return {"result": "Index"}
|
||||
|
||||
|
||||
|
||||
|
@ -31,24 +31,24 @@ class MMVC_Rest_VoiceChanger:
|
||||
buffer = voice.buffer
|
||||
wav = base64.b64decode(buffer)
|
||||
|
||||
if wav == 0:
|
||||
samplerate, data = read("dummy.wav")
|
||||
unpackedData = data
|
||||
else:
|
||||
unpackedData = np.array(struct.unpack(
|
||||
'<%sh' % (len(wav) // struct.calcsize('<h')), wav))
|
||||
# write("logs/received_data.wav", 24000,
|
||||
# unpackedData.astype(np.int16))
|
||||
# if wav == 0:
|
||||
# samplerate, data = read("dummy.wav")
|
||||
# unpackedData = data
|
||||
# else:
|
||||
# unpackedData = np.array(
|
||||
# struct.unpack("<%sh" % (len(wav) // struct.calcsize("<h")), wav)
|
||||
# )
|
||||
|
||||
unpackedData = np.array(
|
||||
struct.unpack("<%sh" % (len(wav) // struct.calcsize("<h")), wav)
|
||||
)
|
||||
|
||||
self.tlock.acquire()
|
||||
changedVoice = self.voiceChangerManager.changeVoice(unpackedData)
|
||||
self.tlock.release()
|
||||
|
||||
changedVoiceBase64 = base64.b64encode(changedVoice[0]).decode('utf-8')
|
||||
data = {
|
||||
"timestamp": timestamp,
|
||||
"changedVoiceBase64": changedVoiceBase64
|
||||
}
|
||||
changedVoiceBase64 = base64.b64encode(changedVoice[0]).decode("utf-8")
|
||||
data = {"timestamp": timestamp, "changedVoiceBase64": changedVoiceBase64}
|
||||
|
||||
json_compatible_item_data = jsonable_encoder(data)
|
||||
return JSONResponse(content=json_compatible_item_data)
|
||||
|
@ -30,7 +30,6 @@ class MMVC_Namespace(socketio.AsyncNamespace):
|
||||
else:
|
||||
unpackedData = np.array(struct.unpack('<%sh' % (len(data) // struct.calcsize('<h')), data)).astype(np.int16)
|
||||
|
||||
# audio1, perf = self.voiceChangerManager.changeVoice(unpackedData)
|
||||
res = self.voiceChangerManager.changeVoice(unpackedData)
|
||||
audio1 = res[0]
|
||||
perf = res[1] if len(res) == 2 else [0, 0, 0]
|
||||
|
BIN
server/tmp.wav
BIN
server/tmp.wav
Binary file not shown.
BIN
server/tmp2.wav
BIN
server/tmp2.wav
Binary file not shown.
@ -1,6 +1,11 @@
|
||||
import sys
|
||||
import os
|
||||
if sys.platform.startswith('darwin'):
|
||||
from voice_changer.utils.LoadModelParams import LoadModelParams
|
||||
|
||||
from voice_changer.utils.VoiceChangerModel import AudioInOut
|
||||
from voice_changer.utils.VoiceChangerParams import VoiceChangerParams
|
||||
|
||||
if sys.platform.startswith("darwin"):
|
||||
baseDir = [x for x in sys.path if x.endswith("Contents/MacOS")]
|
||||
if len(baseDir) != 1:
|
||||
print("baseDir should be only one ", baseDir)
|
||||
@ -10,24 +15,25 @@ if sys.platform.startswith('darwin'):
|
||||
else:
|
||||
sys.path.append("DDSP-SVC")
|
||||
|
||||
import io
|
||||
from dataclasses import dataclass, asdict, field
|
||||
from functools import reduce
|
||||
import numpy as np
|
||||
import torch
|
||||
import onnxruntime
|
||||
import pyworld as pw
|
||||
import ddsp.vocoder as vo
|
||||
from ddsp.core import upsample
|
||||
from enhancer import Enhancer
|
||||
import ddsp.vocoder as vo # type:ignore
|
||||
from ddsp.core import upsample # type:ignore
|
||||
from enhancer import Enhancer # type:ignore
|
||||
|
||||
from Exceptions import NoModeLoadedException
|
||||
|
||||
providers = ['OpenVINOExecutionProvider', "CUDAExecutionProvider", "DmlExecutionProvider", "CPUExecutionProvider"]
|
||||
providers = [
|
||||
"OpenVINOExecutionProvider",
|
||||
"CUDAExecutionProvider",
|
||||
"DmlExecutionProvider",
|
||||
"CPUExecutionProvider",
|
||||
]
|
||||
|
||||
|
||||
@dataclass
|
||||
class DDSP_SVCSettings():
|
||||
class DDSP_SVCSettings:
|
||||
gpu: int = 0
|
||||
dstId: int = 0
|
||||
|
||||
@ -45,18 +51,26 @@ class DDSP_SVCSettings():
|
||||
onnxModelFile: str = ""
|
||||
configFile: str = ""
|
||||
|
||||
speakers: dict[str, int] = field(
|
||||
default_factory=lambda: {}
|
||||
)
|
||||
speakers: dict[str, int] = field(default_factory=lambda: {})
|
||||
|
||||
# ↓mutableな物だけ列挙
|
||||
intData = ["gpu", "dstId", "tran", "predictF0", "extraConvertSize", "enableEnhancer", "enhancerTune"]
|
||||
intData = [
|
||||
"gpu",
|
||||
"dstId",
|
||||
"tran",
|
||||
"predictF0",
|
||||
"extraConvertSize",
|
||||
"enableEnhancer",
|
||||
"enhancerTune",
|
||||
]
|
||||
floatData = ["silentThreshold", "clusterInferRatio"]
|
||||
strData = ["framework", "f0Detector"]
|
||||
|
||||
|
||||
class DDSP_SVC:
|
||||
def __init__(self, params):
|
||||
audio_buffer: AudioInOut | None = None
|
||||
|
||||
def __init__(self, params: VoiceChangerParams):
|
||||
self.settings = DDSP_SVCSettings()
|
||||
self.net_g = None
|
||||
self.onnx_session = None
|
||||
@ -72,24 +86,30 @@ class DDSP_SVC:
|
||||
else:
|
||||
return torch.device("cpu")
|
||||
|
||||
def loadModel(self, props):
|
||||
# self.settings.configFile = props["files"]["configFilename"] # 同じフォルダにあるyamlを使う
|
||||
self.settings.pyTorchModelFile = props["files"]["pyTorchModelFilename"]
|
||||
def loadModel(self, props: LoadModelParams):
|
||||
self.settings.pyTorchModelFile = props.files.pyTorchModelFilename
|
||||
# model
|
||||
model, args = vo.load_model(self.settings.pyTorchModelFile, device=self.useDevice())
|
||||
model, args = vo.load_model(
|
||||
self.settings.pyTorchModelFile, device=self.useDevice()
|
||||
)
|
||||
self.model = model
|
||||
self.args = args
|
||||
self.sampling_rate = args.data.sampling_rate
|
||||
self.hop_size = int(self.args.data.block_size * self.sampling_rate / self.args.data.sampling_rate)
|
||||
self.hop_size = int(
|
||||
self.args.data.block_size
|
||||
* self.sampling_rate
|
||||
/ self.args.data.sampling_rate
|
||||
)
|
||||
|
||||
# hubert
|
||||
self.vec_path = self.params["hubert_soft"]
|
||||
self.vec_path = self.params.hubert_soft
|
||||
self.encoder = vo.Units_Encoder(
|
||||
self.args.data.encoder,
|
||||
self.vec_path,
|
||||
self.args.data.encoder_sample_rate,
|
||||
self.args.data.encoder_hop_size,
|
||||
device=self.useDevice())
|
||||
device=self.useDevice(),
|
||||
)
|
||||
|
||||
# ort_options = onnxruntime.SessionOptions()
|
||||
# ort_options.intra_op_num_threads = 8
|
||||
@ -111,36 +131,59 @@ class DDSP_SVC:
|
||||
self.sampling_rate,
|
||||
self.hop_size,
|
||||
float(50),
|
||||
float(1100))
|
||||
float(1100),
|
||||
)
|
||||
|
||||
self.volume_extractor = vo.Volume_Extractor(self.hop_size)
|
||||
self.enhancer_path = self.params["nsf_hifigan"]
|
||||
self.enhancer = Enhancer(self.args.enhancer.type, self.enhancer_path, device=self.useDevice())
|
||||
self.enhancer_path = self.params.nsf_hifigan
|
||||
self.enhancer = Enhancer(
|
||||
self.args.enhancer.type, self.enhancer_path, device=self.useDevice()
|
||||
)
|
||||
return self.get_info()
|
||||
|
||||
def update_settings(self, key: str, val: any):
|
||||
if key == "onnxExecutionProvider" and self.onnx_session != None:
|
||||
def update_settings(self, key: str, val: int | float | str):
|
||||
if key == "onnxExecutionProvider" and self.onnx_session is not None:
|
||||
if val == "CUDAExecutionProvider":
|
||||
if self.settings.gpu < 0 or self.settings.gpu >= self.gpu_num:
|
||||
self.settings.gpu = 0
|
||||
provider_options = [{'device_id': self.settings.gpu}]
|
||||
self.onnx_session.set_providers(providers=[val], provider_options=provider_options)
|
||||
provider_options = [{"device_id": self.settings.gpu}]
|
||||
self.onnx_session.set_providers(
|
||||
providers=[val], provider_options=provider_options
|
||||
)
|
||||
else:
|
||||
self.onnx_session.set_providers(providers=[val])
|
||||
elif key in self.settings.intData:
|
||||
setattr(self.settings, key, int(val))
|
||||
if key == "gpu" and val >= 0 and val < self.gpu_num and self.onnx_session != None:
|
||||
val = int(val)
|
||||
setattr(self.settings, key, val)
|
||||
if (
|
||||
key == "gpu"
|
||||
and val >= 0
|
||||
and val < self.gpu_num
|
||||
and self.onnx_session is not None
|
||||
):
|
||||
providers = self.onnx_session.get_providers()
|
||||
print("Providers:", providers)
|
||||
if "CUDAExecutionProvider" in providers:
|
||||
provider_options = [{'device_id': self.settings.gpu}]
|
||||
self.onnx_session.set_providers(providers=["CUDAExecutionProvider"], provider_options=provider_options)
|
||||
provider_options = [{"device_id": self.settings.gpu}]
|
||||
self.onnx_session.set_providers(
|
||||
providers=["CUDAExecutionProvider"],
|
||||
provider_options=provider_options,
|
||||
)
|
||||
if key == "gpu" and len(self.settings.pyTorchModelFile) > 0:
|
||||
model, _args = vo.load_model(self.settings.pyTorchModelFile, device=self.useDevice())
|
||||
model, _args = vo.load_model(
|
||||
self.settings.pyTorchModelFile, device=self.useDevice()
|
||||
)
|
||||
self.model = model
|
||||
self.enhancer = Enhancer(self.args.enhancer.type, self.enhancer_path, device=self.useDevice())
|
||||
self.encoder = vo.Units_Encoder(self.args.data.encoder, self.vec_path, self.args.data.encoder_sample_rate,
|
||||
self.args.data.encoder_hop_size, device=self.useDevice())
|
||||
self.enhancer = Enhancer(
|
||||
self.args.enhancer.type, self.enhancer_path, device=self.useDevice()
|
||||
)
|
||||
self.encoder = vo.Units_Encoder(
|
||||
self.args.data.encoder,
|
||||
self.vec_path,
|
||||
self.args.data.encoder_sample_rate,
|
||||
self.args.data.encoder_hop_size,
|
||||
device=self.useDevice(),
|
||||
)
|
||||
|
||||
elif key in self.settings.floatData:
|
||||
setattr(self.settings, key, float(val))
|
||||
@ -148,19 +191,16 @@ class DDSP_SVC:
|
||||
setattr(self.settings, key, str(val))
|
||||
if key == "f0Detector":
|
||||
print("f0Detector update", val)
|
||||
if val == "dio":
|
||||
val = "parselmouth"
|
||||
# if val == "dio":
|
||||
# val = "parselmouth"
|
||||
|
||||
if hasattr(self, "sampling_rate") == False:
|
||||
if hasattr(self, "sampling_rate") is False:
|
||||
self.sampling_rate = 44100
|
||||
self.hop_size = 512
|
||||
|
||||
self.f0_detector = vo.F0_Extractor(
|
||||
val,
|
||||
self.sampling_rate,
|
||||
self.hop_size,
|
||||
float(50),
|
||||
float(1100))
|
||||
val, self.sampling_rate, self.hop_size, float(50), float(1100)
|
||||
)
|
||||
else:
|
||||
return False
|
||||
|
||||
@ -169,10 +209,12 @@ class DDSP_SVC:
|
||||
def get_info(self):
|
||||
data = asdict(self.settings)
|
||||
|
||||
data["onnxExecutionProviders"] = self.onnx_session.get_providers() if self.onnx_session != None else []
|
||||
data["onnxExecutionProviders"] = (
|
||||
self.onnx_session.get_providers() if self.onnx_session is not None else []
|
||||
)
|
||||
files = ["configFile", "pyTorchModelFile", "onnxModelFile"]
|
||||
for f in files:
|
||||
if data[f] != None and os.path.exists(data[f]):
|
||||
if data[f] is not None and os.path.exists(data[f]):
|
||||
data[f] = os.path.basename(data[f])
|
||||
else:
|
||||
data[f] = ""
|
||||
@ -182,41 +224,64 @@ class DDSP_SVC:
|
||||
def get_processing_sampling_rate(self):
|
||||
return self.sampling_rate
|
||||
|
||||
def generate_input(self, newData: any, inputSize: int, crossfadeSize: int, solaSearchFrame: int = 0):
|
||||
def generate_input(
|
||||
self,
|
||||
newData: AudioInOut,
|
||||
inputSize: int,
|
||||
crossfadeSize: int,
|
||||
solaSearchFrame: int = 0,
|
||||
):
|
||||
newData = newData.astype(np.float32) / 32768.0
|
||||
|
||||
if hasattr(self, "audio_buffer"):
|
||||
self.audio_buffer = np.concatenate([self.audio_buffer, newData], 0) # 過去のデータに連結
|
||||
if self.audio_buffer is not None:
|
||||
self.audio_buffer = np.concatenate(
|
||||
[self.audio_buffer, newData], 0
|
||||
) # 過去のデータに連結
|
||||
else:
|
||||
self.audio_buffer = newData
|
||||
|
||||
convertSize = inputSize + crossfadeSize + solaSearchFrame + self.settings.extraConvertSize
|
||||
convertSize = (
|
||||
inputSize + crossfadeSize + solaSearchFrame + self.settings.extraConvertSize
|
||||
)
|
||||
|
||||
if convertSize % self.hop_size != 0: # モデルの出力のホップサイズで切り捨てが発生するので補う。
|
||||
convertSize = convertSize + (self.hop_size - (convertSize % self.hop_size))
|
||||
|
||||
self.audio_buffer = self.audio_buffer[-1 * convertSize:] # 変換対象の部分だけ抽出
|
||||
convertOffset = -1 * convertSize
|
||||
self.audio_buffer = self.audio_buffer[convertOffset:] # 変換対象の部分だけ抽出
|
||||
|
||||
# f0
|
||||
f0 = self.f0_detector.extract(self.audio_buffer * 32768.0, uv_interp=True,
|
||||
silence_front=self.settings.extraConvertSize / self.sampling_rate)
|
||||
f0 = self.f0_detector.extract(
|
||||
self.audio_buffer * 32768.0,
|
||||
uv_interp=True,
|
||||
silence_front=self.settings.extraConvertSize / self.sampling_rate,
|
||||
)
|
||||
f0 = torch.from_numpy(f0).float().unsqueeze(-1).unsqueeze(0)
|
||||
f0 = f0 * 2 ** (float(self.settings.tran) / 12)
|
||||
|
||||
# volume, mask
|
||||
volume = self.volume_extractor.extract(self.audio_buffer)
|
||||
mask = (volume > 10 ** (float(-60) / 20)).astype('float')
|
||||
mask = (volume > 10 ** (float(-60) / 20)).astype("float")
|
||||
mask = np.pad(mask, (4, 4), constant_values=(mask[0], mask[-1]))
|
||||
mask = np.array([np.max(mask[n: n + 9]) for n in range(len(mask) - 8)])
|
||||
mask = np.array(
|
||||
[np.max(mask[n : n + 9]) for n in range(len(mask) - 8)] # noqa: E203
|
||||
)
|
||||
mask = torch.from_numpy(mask).float().unsqueeze(-1).unsqueeze(0)
|
||||
mask = upsample(mask, self.args.data.block_size).squeeze(-1)
|
||||
volume = torch.from_numpy(volume).float().unsqueeze(-1).unsqueeze(0)
|
||||
|
||||
# embed
|
||||
audio = torch.from_numpy(self.audio_buffer).float().to(self.useDevice()).unsqueeze(0)
|
||||
audio = (
|
||||
torch.from_numpy(self.audio_buffer)
|
||||
.float()
|
||||
.to(self.useDevice())
|
||||
.unsqueeze(0)
|
||||
)
|
||||
seg_units = self.encoder.encode(audio, self.sampling_rate, self.hop_size)
|
||||
|
||||
crop = self.audio_buffer[-1 * (inputSize + crossfadeSize):-1 * (crossfadeSize)]
|
||||
cropOffset = -1 * (inputSize + crossfadeSize)
|
||||
cropEnd = -1 * (crossfadeSize)
|
||||
crop = self.audio_buffer[cropOffset:cropEnd]
|
||||
|
||||
rms = np.sqrt(np.square(crop).mean(axis=0))
|
||||
vol = max(rms, self.prevVol * 0.0)
|
||||
@ -225,15 +290,14 @@ class DDSP_SVC:
|
||||
return (seg_units, f0, volume, mask, convertSize, vol)
|
||||
|
||||
def _onnx_inference(self, data):
|
||||
if hasattr(self, "onnx_session") == False or self.onnx_session == None:
|
||||
if hasattr(self, "onnx_session") is False or self.onnx_session is None:
|
||||
print("[Voice Changer] No onnx session.")
|
||||
raise NoModeLoadedException("ONNX")
|
||||
|
||||
raise NoModeLoadedException("ONNX")
|
||||
|
||||
def _pyTorch_inference(self, data):
|
||||
|
||||
if hasattr(self, "model") == False or self.model == None:
|
||||
if hasattr(self, "model") is False or self.model is None:
|
||||
print("[Voice Changer] No pyTorch session.")
|
||||
raise NoModeLoadedException("pytorch")
|
||||
|
||||
@ -242,15 +306,19 @@ class DDSP_SVC:
|
||||
volume = data[2].to(self.useDevice())
|
||||
mask = data[3].to(self.useDevice())
|
||||
|
||||
convertSize = data[4]
|
||||
vol = data[5]
|
||||
# convertSize = data[4]
|
||||
# vol = data[5]
|
||||
# if vol < self.settings.silentThreshold:
|
||||
# print("threshold")
|
||||
# return np.zeros(convertSize).astype(np.int16)
|
||||
|
||||
with torch.no_grad():
|
||||
spk_id = torch.LongTensor(np.array([[self.settings.dstId]])).to(self.useDevice())
|
||||
seg_output, _, (s_h, s_n) = self.model(c, f0, volume, spk_id=spk_id, spk_mix_dict=None)
|
||||
spk_id = torch.LongTensor(np.array([[self.settings.dstId]])).to(
|
||||
self.useDevice()
|
||||
)
|
||||
seg_output, _, (s_h, s_n) = self.model(
|
||||
c, f0, volume, spk_id=spk_id, spk_mix_dict=None
|
||||
)
|
||||
seg_output *= mask
|
||||
|
||||
if self.settings.enableEnhancer:
|
||||
@ -260,8 +328,9 @@ class DDSP_SVC:
|
||||
f0,
|
||||
self.args.data.block_size,
|
||||
# adaptive_key=float(self.settings.enhancerTune),
|
||||
adaptive_key='auto',
|
||||
silence_front=self.settings.extraConvertSize / self.sampling_rate)
|
||||
adaptive_key="auto",
|
||||
silence_front=self.settings.extraConvertSize / self.sampling_rate,
|
||||
)
|
||||
|
||||
result = seg_output.squeeze().cpu().numpy() * 32768.0
|
||||
return np.array(result).astype(np.int16)
|
||||
@ -282,7 +351,7 @@ class DDSP_SVC:
|
||||
del self.onnx_session
|
||||
|
||||
remove_path = os.path.join("DDSP-SVC")
|
||||
sys.path = [x for x in sys.path if x.endswith(remove_path) == False]
|
||||
sys.path = [x for x in sys.path if x.endswith(remove_path) is False]
|
||||
|
||||
for key in list(sys.modules):
|
||||
val = sys.modules.get(key)
|
||||
@ -291,5 +360,5 @@ class DDSP_SVC:
|
||||
if file_path.find("DDSP-SVC" + os.path.sep) >= 0:
|
||||
print("remove", key, file_path)
|
||||
sys.modules.pop(key)
|
||||
except Exception as e:
|
||||
except: # type:ignore
|
||||
pass
|
||||
|
@ -1,40 +0,0 @@
|
||||
import os
|
||||
import numpy as np
|
||||
import pylab
|
||||
import librosa
|
||||
import librosa.display
|
||||
import pyworld as pw
|
||||
|
||||
|
||||
class IOAnalyzer:
|
||||
|
||||
def _get_f0_dio(self, y, sr):
|
||||
_f0, time = pw.dio(y, sr, frame_period=5)
|
||||
f0 = pw.stonemask(y, _f0, time, sr)
|
||||
time = np.linspace(0, y.shape[0] / sr, len(time))
|
||||
return f0, time
|
||||
|
||||
def _get_f0_harvest(self, y, sr):
|
||||
_f0, time = pw.harvest(y, sr, frame_period=5)
|
||||
f0 = pw.stonemask(y, _f0, time, sr)
|
||||
time = np.linspace(0, y.shape[0] / sr, len(time))
|
||||
return f0, time
|
||||
|
||||
def analyze(self, inputDataFile: str, dioImageFile: str, harvestImageFile: str, samplingRate: int):
|
||||
y, sr = librosa.load(inputDataFile, samplingRate)
|
||||
y = y.astype(np.float64)
|
||||
spec = librosa.amplitude_to_db(np.abs(librosa.stft(y, n_fft=2048, win_length=2048, hop_length=128)), ref=np.max)
|
||||
f0_dio, times = self._get_f0_dio(y, sr=samplingRate)
|
||||
f0_harvest, times = self._get_f0_harvest(y, sr=samplingRate)
|
||||
|
||||
pylab.close()
|
||||
HOP_LENGTH = 128
|
||||
img = librosa.display.specshow(spec, sr=samplingRate, hop_length=HOP_LENGTH, x_axis='time', y_axis='log', )
|
||||
pylab.plot(times, f0_dio, label='f0', color=(0, 1, 1, 0.6), linewidth=3)
|
||||
pylab.savefig(dioImageFile)
|
||||
|
||||
pylab.close()
|
||||
HOP_LENGTH = 128
|
||||
img = librosa.display.specshow(spec, sr=samplingRate, hop_length=HOP_LENGTH, x_axis='time', y_axis='log', )
|
||||
pylab.plot(times, f0_harvest, label='f0', color=(0, 1, 1, 0.6), linewidth=3)
|
||||
pylab.savefig(harvestImageFile)
|
@ -1,6 +1,10 @@
|
||||
import sys
|
||||
import os
|
||||
if sys.platform.startswith('darwin'):
|
||||
|
||||
from voice_changer.utils.LoadModelParams import LoadModelParams
|
||||
from voice_changer.utils.VoiceChangerModel import AudioInOut
|
||||
|
||||
if sys.platform.startswith("darwin"):
|
||||
baseDir = [x for x in sys.path if x.endswith("Contents/MacOS")]
|
||||
if len(baseDir) != 1:
|
||||
print("baseDir should be only one ", baseDir)
|
||||
@ -12,23 +16,32 @@ else:
|
||||
sys.path.append(modulePath)
|
||||
|
||||
|
||||
from dataclasses import dataclass, asdict
|
||||
from dataclasses import dataclass, asdict, field
|
||||
import numpy as np
|
||||
import torch
|
||||
import onnxruntime
|
||||
import pyworld as pw
|
||||
|
||||
from symbols import symbols
|
||||
from models import SynthesizerTrn
|
||||
from voice_changer.MMVCv13.TrainerFunctions import TextAudioSpeakerCollate, spectrogram_torch, load_checkpoint, get_hparams_from_file
|
||||
from symbols import symbols # type:ignore
|
||||
from models import SynthesizerTrn # type:ignore
|
||||
from voice_changer.MMVCv13.TrainerFunctions import (
|
||||
TextAudioSpeakerCollate,
|
||||
spectrogram_torch,
|
||||
load_checkpoint,
|
||||
get_hparams_from_file,
|
||||
)
|
||||
|
||||
from Exceptions import NoModeLoadedException
|
||||
|
||||
providers = ['OpenVINOExecutionProvider', "CUDAExecutionProvider", "DmlExecutionProvider", "CPUExecutionProvider"]
|
||||
providers = [
|
||||
"OpenVINOExecutionProvider",
|
||||
"CUDAExecutionProvider",
|
||||
"DmlExecutionProvider",
|
||||
"CPUExecutionProvider",
|
||||
]
|
||||
|
||||
|
||||
@dataclass
|
||||
class MMVCv13Settings():
|
||||
class MMVCv13Settings:
|
||||
gpu: int = 0
|
||||
srcId: int = 0
|
||||
dstId: int = 101
|
||||
@ -40,11 +53,13 @@ class MMVCv13Settings():
|
||||
|
||||
# ↓mutableな物だけ列挙
|
||||
intData = ["gpu", "srcId", "dstId"]
|
||||
floatData = []
|
||||
floatData: list[str] = field(default_factory=lambda: [])
|
||||
strData = ["framework"]
|
||||
|
||||
|
||||
class MMVCv13:
|
||||
audio_buffer: AudioInOut | None = None
|
||||
|
||||
def __init__(self):
|
||||
self.settings = MMVCv13Settings()
|
||||
self.net_g = None
|
||||
@ -53,51 +68,62 @@ class MMVCv13:
|
||||
self.gpu_num = torch.cuda.device_count()
|
||||
self.text_norm = torch.LongTensor([0, 6, 0])
|
||||
|
||||
def loadModel(self, props):
|
||||
self.settings.configFile = props["files"]["configFilename"]
|
||||
def loadModel(self, props: LoadModelParams):
|
||||
self.settings.configFile = props.files.configFilename
|
||||
self.hps = get_hparams_from_file(self.settings.configFile)
|
||||
|
||||
self.settings.pyTorchModelFile = props["files"]["pyTorchModelFilename"]
|
||||
self.settings.onnxModelFile = props["files"]["onnxModelFilename"]
|
||||
self.settings.pyTorchModelFile = props.files.pyTorchModelFilename
|
||||
self.settings.onnxModelFile = props.files.onnxModelFilename
|
||||
|
||||
# PyTorchモデル生成
|
||||
if self.settings.pyTorchModelFile != None:
|
||||
if self.settings.pyTorchModelFile is not None:
|
||||
self.net_g = SynthesizerTrn(
|
||||
len(symbols),
|
||||
self.hps.data.filter_length // 2 + 1,
|
||||
self.hps.train.segment_size // self.hps.data.hop_length,
|
||||
n_speakers=self.hps.data.n_speakers,
|
||||
**self.hps.model)
|
||||
**self.hps.model
|
||||
)
|
||||
self.net_g.eval()
|
||||
load_checkpoint(self.settings.pyTorchModelFile, self.net_g, None)
|
||||
|
||||
# ONNXモデル生成
|
||||
if self.settings.onnxModelFile != None:
|
||||
if self.settings.onnxModelFile is not None:
|
||||
ort_options = onnxruntime.SessionOptions()
|
||||
ort_options.intra_op_num_threads = 8
|
||||
self.onnx_session = onnxruntime.InferenceSession(
|
||||
self.settings.onnxModelFile,
|
||||
providers=providers
|
||||
self.settings.onnxModelFile, providers=providers
|
||||
)
|
||||
return self.get_info()
|
||||
|
||||
def update_settings(self, key: str, val: any):
|
||||
if key == "onnxExecutionProvider" and self.onnx_session != None:
|
||||
def update_settings(self, key: str, val: int | float | str):
|
||||
if key == "onnxExecutionProvider" and self.onnx_session is not None:
|
||||
if val == "CUDAExecutionProvider":
|
||||
if self.settings.gpu < 0 or self.settings.gpu >= self.gpu_num:
|
||||
self.settings.gpu = 0
|
||||
provider_options = [{'device_id': self.settings.gpu}]
|
||||
self.onnx_session.set_providers(providers=[val], provider_options=provider_options)
|
||||
provider_options = [{"device_id": self.settings.gpu}]
|
||||
self.onnx_session.set_providers(
|
||||
providers=[val], provider_options=provider_options
|
||||
)
|
||||
else:
|
||||
self.onnx_session.set_providers(providers=[val])
|
||||
elif key in self.settings.intData:
|
||||
setattr(self.settings, key, int(val))
|
||||
if key == "gpu" and val >= 0 and val < self.gpu_num and self.onnx_session != None:
|
||||
val = int(val)
|
||||
setattr(self.settings, key, val)
|
||||
if (
|
||||
key == "gpu"
|
||||
and val >= 0
|
||||
and val < self.gpu_num
|
||||
and self.onnx_session is not None
|
||||
):
|
||||
providers = self.onnx_session.get_providers()
|
||||
print("Providers:", providers)
|
||||
if "CUDAExecutionProvider" in providers:
|
||||
provider_options = [{'device_id': self.settings.gpu}]
|
||||
self.onnx_session.set_providers(providers=["CUDAExecutionProvider"], provider_options=provider_options)
|
||||
provider_options = [{"device_id": self.settings.gpu}]
|
||||
self.onnx_session.set_providers(
|
||||
providers=["CUDAExecutionProvider"],
|
||||
provider_options=provider_options,
|
||||
)
|
||||
elif key in self.settings.floatData:
|
||||
setattr(self.settings, key, float(val))
|
||||
elif key in self.settings.strData:
|
||||
@ -110,10 +136,12 @@ class MMVCv13:
|
||||
def get_info(self):
|
||||
data = asdict(self.settings)
|
||||
|
||||
data["onnxExecutionProviders"] = self.onnx_session.get_providers() if self.onnx_session != None else []
|
||||
data["onnxExecutionProviders"] = (
|
||||
self.onnx_session.get_providers() if self.onnx_session is not None else []
|
||||
)
|
||||
files = ["configFile", "pyTorchModelFile", "onnxModelFile"]
|
||||
for f in files:
|
||||
if data[f] != None and os.path.exists(data[f]):
|
||||
if data[f] is not None and os.path.exists(data[f]):
|
||||
data[f] = os.path.basename(data[f])
|
||||
else:
|
||||
data[f] = ""
|
||||
@ -121,22 +149,35 @@ class MMVCv13:
|
||||
return data
|
||||
|
||||
def get_processing_sampling_rate(self):
|
||||
if hasattr(self, "hps") == False:
|
||||
if hasattr(self, "hps") is False:
|
||||
raise NoModeLoadedException("config")
|
||||
return self.hps.data.sampling_rate
|
||||
|
||||
def _get_spec(self, audio: any):
|
||||
spec = spectrogram_torch(audio, self.hps.data.filter_length,
|
||||
self.hps.data.sampling_rate, self.hps.data.hop_length, self.hps.data.win_length,
|
||||
center=False)
|
||||
def _get_spec(self, audio: AudioInOut):
|
||||
spec = spectrogram_torch(
|
||||
audio,
|
||||
self.hps.data.filter_length,
|
||||
self.hps.data.sampling_rate,
|
||||
self.hps.data.hop_length,
|
||||
self.hps.data.win_length,
|
||||
center=False,
|
||||
)
|
||||
spec = torch.squeeze(spec, 0)
|
||||
return spec
|
||||
|
||||
def generate_input(self, newData: any, inputSize: int, crossfadeSize: int, solaSearchFrame: int = 0):
|
||||
def generate_input(
|
||||
self,
|
||||
newData: AudioInOut,
|
||||
inputSize: int,
|
||||
crossfadeSize: int,
|
||||
solaSearchFrame: int = 0,
|
||||
):
|
||||
newData = newData.astype(np.float32) / self.hps.data.max_wav_value
|
||||
|
||||
if hasattr(self, "audio_buffer"):
|
||||
self.audio_buffer = np.concatenate([self.audio_buffer, newData], 0) # 過去のデータに連結
|
||||
if self.audio_buffer is not None:
|
||||
self.audio_buffer = np.concatenate(
|
||||
[self.audio_buffer, newData], 0
|
||||
) # 過去のデータに連結
|
||||
else:
|
||||
self.audio_buffer = newData
|
||||
|
||||
@ -145,9 +186,12 @@ class MMVCv13:
|
||||
if convertSize < 8192:
|
||||
convertSize = 8192
|
||||
if convertSize % self.hps.data.hop_length != 0: # モデルの出力のホップサイズで切り捨てが発生するので補う。
|
||||
convertSize = convertSize + (self.hps.data.hop_length - (convertSize % self.hps.data.hop_length))
|
||||
convertSize = convertSize + (
|
||||
self.hps.data.hop_length - (convertSize % self.hps.data.hop_length)
|
||||
)
|
||||
|
||||
self.audio_buffer = self.audio_buffer[-1 * convertSize:] # 変換対象の部分だけ抽出
|
||||
convertOffset = -1 * convertSize
|
||||
self.audio_buffer = self.audio_buffer[convertOffset:] # 変換対象の部分だけ抽出
|
||||
|
||||
audio = torch.FloatTensor(self.audio_buffer)
|
||||
audio_norm = audio.unsqueeze(0) # unsqueeze
|
||||
@ -160,25 +204,29 @@ class MMVCv13:
|
||||
return data
|
||||
|
||||
def _onnx_inference(self, data):
|
||||
if hasattr(self, "onnx_session") == False or self.onnx_session == None:
|
||||
if hasattr(self, "onnx_session") is False or self.onnx_session is None:
|
||||
print("[Voice Changer] No ONNX session.")
|
||||
raise NoModeLoadedException("ONNX")
|
||||
|
||||
x, x_lengths, spec, spec_lengths, y, y_lengths, sid_src = [x for x in data]
|
||||
sid_tgt1 = torch.LongTensor([self.settings.dstId])
|
||||
# if spec.size()[2] >= 8:
|
||||
audio1 = self.onnx_session.run(
|
||||
audio1 = (
|
||||
self.onnx_session.run(
|
||||
["audio"],
|
||||
{
|
||||
"specs": spec.numpy(),
|
||||
"lengths": spec_lengths.numpy(),
|
||||
"sid_src": sid_src.numpy(),
|
||||
"sid_tgt": sid_tgt1.numpy()
|
||||
})[0][0, 0] * self.hps.data.max_wav_value
|
||||
"sid_tgt": sid_tgt1.numpy(),
|
||||
},
|
||||
)[0][0, 0]
|
||||
* self.hps.data.max_wav_value
|
||||
)
|
||||
return audio1
|
||||
|
||||
def _pyTorch_inference(self, data):
|
||||
if hasattr(self, "net_g") == False or self.net_g == None:
|
||||
if hasattr(self, "net_g") is False or self.net_g is None:
|
||||
print("[Voice Changer] No pyTorch session.")
|
||||
raise NoModeLoadedException("pytorch")
|
||||
|
||||
@ -188,11 +236,19 @@ class MMVCv13:
|
||||
dev = torch.device("cuda", index=self.settings.gpu)
|
||||
|
||||
with torch.no_grad():
|
||||
x, x_lengths, spec, spec_lengths, y, y_lengths, sid_src = [x.to(dev) for x in data]
|
||||
x, x_lengths, spec, spec_lengths, y, y_lengths, sid_src = [
|
||||
x.to(dev) for x in data
|
||||
]
|
||||
sid_target = torch.LongTensor([self.settings.dstId]).to(dev)
|
||||
|
||||
audio1 = (self.net_g.to(dev).voice_conversion(spec, spec_lengths, sid_src=sid_src,
|
||||
sid_tgt=sid_target)[0, 0].data * self.hps.data.max_wav_value)
|
||||
audio1 = (
|
||||
self.net_g.to(dev)
|
||||
.voice_conversion(
|
||||
spec, spec_lengths, sid_src=sid_src, sid_tgt=sid_target
|
||||
)[0, 0]
|
||||
.data
|
||||
* self.hps.data.max_wav_value
|
||||
)
|
||||
result = audio1.float().cpu().numpy()
|
||||
|
||||
return result
|
||||
@ -208,7 +264,7 @@ class MMVCv13:
|
||||
del self.net_g
|
||||
del self.onnx_session
|
||||
remove_path = os.path.join("MMVC_Client_v13", "python")
|
||||
sys.path = [x for x in sys.path if x.endswith(remove_path) == False]
|
||||
sys.path = [x for x in sys.path if x.endswith(remove_path) is False]
|
||||
|
||||
for key in list(sys.modules):
|
||||
val = sys.modules.get(key)
|
||||
@ -217,5 +273,5 @@ class MMVCv13:
|
||||
if file_path.find(remove_path + os.path.sep) >= 0:
|
||||
print("remove", key, file_path)
|
||||
sys.modules.pop(key)
|
||||
except Exception as e:
|
||||
except: # type:ignore
|
||||
pass
|
||||
|
@ -1,36 +1,58 @@
|
||||
import torch
|
||||
import os, sys, json
|
||||
import os
|
||||
import sys
|
||||
import json
|
||||
import logging
|
||||
|
||||
logging.basicConfig(stream=sys.stdout, level=logging.DEBUG)
|
||||
logger = logging
|
||||
|
||||
hann_window = {}
|
||||
|
||||
|
||||
def spectrogram_torch(y, n_fft, sampling_rate, hop_size, win_size, center=False):
|
||||
if torch.min(y) < -1.:
|
||||
print('min value is ', torch.min(y))
|
||||
if torch.max(y) > 1.:
|
||||
print('max value is ', torch.max(y))
|
||||
if torch.min(y) < -1.0:
|
||||
print("min value is ", torch.min(y))
|
||||
if torch.max(y) > 1.0:
|
||||
print("max value is ", torch.max(y))
|
||||
|
||||
global hann_window
|
||||
dtype_device = str(y.dtype) + '_' + str(y.device)
|
||||
wnsize_dtype_device = str(win_size) + '_' + dtype_device
|
||||
dtype_device = str(y.dtype) + "_" + str(y.device)
|
||||
wnsize_dtype_device = str(win_size) + "_" + dtype_device
|
||||
if wnsize_dtype_device not in hann_window:
|
||||
hann_window[wnsize_dtype_device] = torch.hann_window(win_size).to(dtype=y.dtype, device=y.device)
|
||||
hann_window[wnsize_dtype_device] = torch.hann_window(win_size).to(
|
||||
dtype=y.dtype, device=y.device
|
||||
)
|
||||
|
||||
y = torch.nn.functional.pad(y.unsqueeze(1), (int((n_fft-hop_size)/2), int((n_fft-hop_size)/2)), mode='reflect')
|
||||
y = torch.nn.functional.pad(
|
||||
y.unsqueeze(1),
|
||||
(int((n_fft - hop_size) / 2), int((n_fft - hop_size) / 2)),
|
||||
mode="reflect",
|
||||
)
|
||||
y = y.squeeze(1)
|
||||
|
||||
spec = torch.stft(y, n_fft, hop_length=hop_size, win_length=win_size, window=hann_window[wnsize_dtype_device],
|
||||
center=center, pad_mode='reflect', normalized=False, onesided=True, return_complex=True)
|
||||
spec = torch.stft(
|
||||
y,
|
||||
n_fft,
|
||||
hop_length=hop_size,
|
||||
win_length=win_size,
|
||||
window=hann_window[wnsize_dtype_device],
|
||||
center=center,
|
||||
pad_mode="reflect",
|
||||
normalized=False,
|
||||
onesided=True,
|
||||
return_complex=True,
|
||||
)
|
||||
spec = torch.view_as_real(spec)
|
||||
|
||||
spec = torch.sqrt(spec.pow(2).sum(-1) + 1e-6)
|
||||
return spec
|
||||
|
||||
class TextAudioSpeakerCollate():
|
||||
""" Zero-pads model inputs and targets
|
||||
"""
|
||||
def __init__(self, return_ids=False, no_text = False):
|
||||
|
||||
class TextAudioSpeakerCollate:
|
||||
"""Zero-pads model inputs and targets"""
|
||||
|
||||
def __init__(self, return_ids=False, no_text=False):
|
||||
self.return_ids = return_ids
|
||||
self.no_text = no_text
|
||||
|
||||
@ -42,8 +64,8 @@ class TextAudioSpeakerCollate():
|
||||
"""
|
||||
# Right zero-pad all one-hot text sequences to max input length
|
||||
_, ids_sorted_decreasing = torch.sort(
|
||||
torch.LongTensor([x[1].size(1) for x in batch]),
|
||||
dim=0, descending=True)
|
||||
torch.LongTensor([x[1].size(1) for x in batch]), dim=0, descending=True
|
||||
)
|
||||
|
||||
max_text_len = max([len(x[0]) for x in batch])
|
||||
max_spec_len = max([x[1].size(1) for x in batch])
|
||||
@ -64,49 +86,69 @@ class TextAudioSpeakerCollate():
|
||||
row = batch[ids_sorted_decreasing[i]]
|
||||
|
||||
text = row[0]
|
||||
text_padded[i, :text.size(0)] = text
|
||||
text_padded[i, : text.size(0)] = text
|
||||
text_lengths[i] = text.size(0)
|
||||
|
||||
spec = row[1]
|
||||
spec_padded[i, :, :spec.size(1)] = spec
|
||||
spec_padded[i, :, : spec.size(1)] = spec
|
||||
spec_lengths[i] = spec.size(1)
|
||||
|
||||
wav = row[2]
|
||||
wav_padded[i, :, :wav.size(1)] = wav
|
||||
wav_padded[i, :, : wav.size(1)] = wav
|
||||
wav_lengths[i] = wav.size(1)
|
||||
|
||||
sid[i] = row[3]
|
||||
|
||||
if self.return_ids:
|
||||
return text_padded, text_lengths, spec_padded, spec_lengths, wav_padded, wav_lengths, sid, ids_sorted_decreasing
|
||||
return text_padded, text_lengths, spec_padded, spec_lengths, wav_padded, wav_lengths, sid
|
||||
return (
|
||||
text_padded,
|
||||
text_lengths,
|
||||
spec_padded,
|
||||
spec_lengths,
|
||||
wav_padded,
|
||||
wav_lengths,
|
||||
sid,
|
||||
ids_sorted_decreasing,
|
||||
)
|
||||
return (
|
||||
text_padded,
|
||||
text_lengths,
|
||||
spec_padded,
|
||||
spec_lengths,
|
||||
wav_padded,
|
||||
wav_lengths,
|
||||
sid,
|
||||
)
|
||||
|
||||
|
||||
def load_checkpoint(checkpoint_path, model, optimizer=None):
|
||||
assert os.path.isfile(checkpoint_path), f"No such file or directory: {checkpoint_path}"
|
||||
checkpoint_dict = torch.load(checkpoint_path, map_location='cpu')
|
||||
iteration = checkpoint_dict['iteration']
|
||||
learning_rate = checkpoint_dict['learning_rate']
|
||||
assert os.path.isfile(
|
||||
checkpoint_path
|
||||
), f"No such file or directory: {checkpoint_path}"
|
||||
checkpoint_dict = torch.load(checkpoint_path, map_location="cpu")
|
||||
iteration = checkpoint_dict["iteration"]
|
||||
learning_rate = checkpoint_dict["learning_rate"]
|
||||
if optimizer is not None:
|
||||
optimizer.load_state_dict(checkpoint_dict['optimizer'])
|
||||
saved_state_dict = checkpoint_dict['model']
|
||||
if hasattr(model, 'module'):
|
||||
optimizer.load_state_dict(checkpoint_dict["optimizer"])
|
||||
saved_state_dict = checkpoint_dict["model"]
|
||||
if hasattr(model, "module"):
|
||||
state_dict = model.module.state_dict()
|
||||
else:
|
||||
state_dict = model.state_dict()
|
||||
new_state_dict= {}
|
||||
new_state_dict = {}
|
||||
for k, v in state_dict.items():
|
||||
try:
|
||||
new_state_dict[k] = saved_state_dict[k]
|
||||
except:
|
||||
logger.info("%s is not in the checkpoint" % k)
|
||||
new_state_dict[k] = v
|
||||
if hasattr(model, 'module'):
|
||||
if hasattr(model, "module"):
|
||||
model.module.load_state_dict(new_state_dict)
|
||||
else:
|
||||
model.load_state_dict(new_state_dict)
|
||||
logger.info("Loaded checkpoint '{}' (iteration {})" .format(
|
||||
checkpoint_path, iteration))
|
||||
logger.info(
|
||||
"Loaded checkpoint '{}' (iteration {})".format(checkpoint_path, iteration)
|
||||
)
|
||||
return model, optimizer, learning_rate, iteration
|
||||
|
||||
|
||||
@ -115,10 +157,11 @@ def get_hparams_from_file(config_path):
|
||||
data = f.read()
|
||||
config = json.loads(data)
|
||||
|
||||
hparams =HParams(**config)
|
||||
hparams = HParams(**config)
|
||||
return hparams
|
||||
|
||||
class HParams():
|
||||
|
||||
class HParams:
|
||||
def __init__(self, **kwargs):
|
||||
for k, v in kwargs.items():
|
||||
if type(v) == dict:
|
||||
@ -148,4 +191,3 @@ class HParams():
|
||||
|
||||
def __repr__(self):
|
||||
return self.__dict__.__repr__()
|
||||
|
||||
|
@ -1,6 +1,10 @@
|
||||
import sys
|
||||
import os
|
||||
if sys.platform.startswith('darwin'):
|
||||
|
||||
from voice_changer.utils.LoadModelParams import LoadModelParams
|
||||
from voice_changer.utils.VoiceChangerModel import AudioInOut
|
||||
|
||||
if sys.platform.startswith("darwin"):
|
||||
baseDir = [x for x in sys.path if x.endswith("Contents/MacOS")]
|
||||
if len(baseDir) != 1:
|
||||
print("baseDir should be only one ", baseDir)
|
||||
@ -17,16 +21,26 @@ import torch
|
||||
import onnxruntime
|
||||
import pyworld as pw
|
||||
|
||||
from models import SynthesizerTrn
|
||||
from voice_changer.MMVCv15.client_modules import convert_continuos_f0, spectrogram_torch, get_hparams_from_file, load_checkpoint
|
||||
from models import SynthesizerTrn # type:ignore
|
||||
from voice_changer.MMVCv15.client_modules import (
|
||||
convert_continuos_f0,
|
||||
spectrogram_torch,
|
||||
get_hparams_from_file,
|
||||
load_checkpoint,
|
||||
)
|
||||
|
||||
from Exceptions import NoModeLoadedException, ONNXInputArgumentException
|
||||
|
||||
providers = ['OpenVINOExecutionProvider', "CUDAExecutionProvider", "DmlExecutionProvider", "CPUExecutionProvider"]
|
||||
providers = [
|
||||
"OpenVINOExecutionProvider",
|
||||
"CUDAExecutionProvider",
|
||||
"DmlExecutionProvider",
|
||||
"CPUExecutionProvider",
|
||||
]
|
||||
|
||||
|
||||
@dataclass
|
||||
class MMVCv15Settings():
|
||||
class MMVCv15Settings:
|
||||
gpu: int = 0
|
||||
srcId: int = 0
|
||||
dstId: int = 101
|
||||
@ -46,6 +60,8 @@ class MMVCv15Settings():
|
||||
|
||||
|
||||
class MMVCv15:
|
||||
audio_buffer: AudioInOut | None = None
|
||||
|
||||
def __init__(self):
|
||||
self.settings = MMVCv15Settings()
|
||||
self.net_g = None
|
||||
@ -53,13 +69,12 @@ class MMVCv15:
|
||||
|
||||
self.gpu_num = torch.cuda.device_count()
|
||||
|
||||
def loadModel(self, props):
|
||||
|
||||
self.settings.configFile = props["files"]["configFilename"]
|
||||
def loadModel(self, props: LoadModelParams):
|
||||
self.settings.configFile = props.files.configFilename
|
||||
self.hps = get_hparams_from_file(self.settings.configFile)
|
||||
|
||||
self.settings.pyTorchModelFile = props["files"]["pyTorchModelFilename"]
|
||||
self.settings.onnxModelFile = props["files"]["onnxModelFilename"]
|
||||
self.settings.pyTorchModelFile = props.files.pyTorchModelFilename
|
||||
self.settings.onnxModelFile = props.files.onnxModelFilename
|
||||
|
||||
# PyTorchモデル生成
|
||||
self.net_g = SynthesizerTrn(
|
||||
@ -78,20 +93,19 @@ class MMVCv15:
|
||||
requires_grad_pe=self.hps.requires_grad.pe,
|
||||
requires_grad_flow=self.hps.requires_grad.flow,
|
||||
requires_grad_text_enc=self.hps.requires_grad.text_enc,
|
||||
requires_grad_dec=self.hps.requires_grad.dec
|
||||
requires_grad_dec=self.hps.requires_grad.dec,
|
||||
)
|
||||
if self.settings.pyTorchModelFile != None:
|
||||
if self.settings.pyTorchModelFile is not None:
|
||||
self.net_g.eval()
|
||||
load_checkpoint(self.settings.pyTorchModelFile, self.net_g, None)
|
||||
|
||||
# ONNXモデル生成
|
||||
self.onxx_input_length = 8192
|
||||
if self.settings.onnxModelFile != None:
|
||||
if self.settings.onnxModelFile is not None:
|
||||
ort_options = onnxruntime.SessionOptions()
|
||||
ort_options.intra_op_num_threads = 8
|
||||
self.onnx_session = onnxruntime.InferenceSession(
|
||||
self.settings.onnxModelFile,
|
||||
providers=providers
|
||||
self.settings.onnxModelFile, providers=providers
|
||||
)
|
||||
inputs_info = self.onnx_session.get_inputs()
|
||||
for i in inputs_info:
|
||||
@ -100,23 +114,39 @@ class MMVCv15:
|
||||
self.onxx_input_length = i.shape[2]
|
||||
return self.get_info()
|
||||
|
||||
def update_settings(self, key: str, val: any):
|
||||
if key == "onnxExecutionProvider" and self.settings.onnxModelFile != "": # self.onnx_session != None:
|
||||
def update_settings(self, key: str, val: int | float | str):
|
||||
if (
|
||||
key == "onnxExecutionProvider"
|
||||
and self.settings.onnxModelFile != ""
|
||||
and self.settings.onnxModelFile is not None
|
||||
):
|
||||
if val == "CUDAExecutionProvider":
|
||||
if self.settings.gpu < 0 or self.settings.gpu >= self.gpu_num:
|
||||
self.settings.gpu = 0
|
||||
provider_options = [{'device_id': self.settings.gpu}]
|
||||
self.onnx_session.set_providers(providers=[val], provider_options=provider_options)
|
||||
provider_options = [{"device_id": self.settings.gpu}]
|
||||
self.onnx_session.set_providers(
|
||||
providers=[val], provider_options=provider_options
|
||||
)
|
||||
else:
|
||||
self.onnx_session.set_providers(providers=[val])
|
||||
elif key in self.settings.intData:
|
||||
setattr(self.settings, key, int(val))
|
||||
if key == "gpu" and val >= 0 and val < self.gpu_num and self.settings.onnxModelFile != "": # self.onnx_session != None:
|
||||
val = int(val)
|
||||
setattr(self.settings, key, val)
|
||||
if (
|
||||
key == "gpu"
|
||||
and val >= 0
|
||||
and val < self.gpu_num
|
||||
and self.settings.onnxModelFile != ""
|
||||
and self.settings.onnxModelFile is not None
|
||||
):
|
||||
providers = self.onnx_session.get_providers()
|
||||
print("Providers:", providers)
|
||||
if "CUDAExecutionProvider" in providers:
|
||||
provider_options = [{'device_id': self.settings.gpu}]
|
||||
self.onnx_session.set_providers(providers=["CUDAExecutionProvider"], provider_options=provider_options)
|
||||
provider_options = [{"device_id": self.settings.gpu}]
|
||||
self.onnx_session.set_providers(
|
||||
providers=["CUDAExecutionProvider"],
|
||||
provider_options=provider_options,
|
||||
)
|
||||
elif key in self.settings.floatData:
|
||||
setattr(self.settings, key, float(val))
|
||||
elif key in self.settings.strData:
|
||||
@ -129,10 +159,15 @@ class MMVCv15:
|
||||
def get_info(self):
|
||||
data = asdict(self.settings)
|
||||
|
||||
data["onnxExecutionProviders"] = self.onnx_session.get_providers() if self.settings.onnxModelFile != "" else []
|
||||
data["onnxExecutionProviders"] = (
|
||||
self.onnx_session.get_providers()
|
||||
if self.settings.onnxModelFile != ""
|
||||
and self.settings.onnxModelFile is not None
|
||||
else []
|
||||
)
|
||||
files = ["configFile", "pyTorchModelFile", "onnxModelFile"]
|
||||
for f in files:
|
||||
if data[f] != None and os.path.exists(data[f]):
|
||||
if data[f] is not None and os.path.exists(data[f]):
|
||||
data[f] = os.path.basename(data[f])
|
||||
else:
|
||||
data[f] = ""
|
||||
@ -140,36 +175,58 @@ class MMVCv15:
|
||||
return data
|
||||
|
||||
def get_processing_sampling_rate(self):
|
||||
if hasattr(self, "hps") == False:
|
||||
if hasattr(self, "hps") is False:
|
||||
raise NoModeLoadedException("config")
|
||||
return self.hps.data.sampling_rate
|
||||
|
||||
def _get_f0(self, detector: str, newData: any):
|
||||
|
||||
def _get_f0(self, detector: str, newData: AudioInOut):
|
||||
audio_norm_np = newData.astype(np.float64)
|
||||
if detector == "dio":
|
||||
_f0, _time = pw.dio(audio_norm_np, self.hps.data.sampling_rate, frame_period=5.5)
|
||||
_f0, _time = pw.dio(
|
||||
audio_norm_np, self.hps.data.sampling_rate, frame_period=5.5
|
||||
)
|
||||
f0 = pw.stonemask(audio_norm_np, _f0, _time, self.hps.data.sampling_rate)
|
||||
else:
|
||||
f0, t = pw.harvest(audio_norm_np, self.hps.data.sampling_rate, frame_period=5.5, f0_floor=71.0, f0_ceil=1000.0)
|
||||
f0 = convert_continuos_f0(f0, int(audio_norm_np.shape[0] / self.hps.data.hop_length))
|
||||
f0, t = pw.harvest(
|
||||
audio_norm_np,
|
||||
self.hps.data.sampling_rate,
|
||||
frame_period=5.5,
|
||||
f0_floor=71.0,
|
||||
f0_ceil=1000.0,
|
||||
)
|
||||
f0 = convert_continuos_f0(
|
||||
f0, int(audio_norm_np.shape[0] / self.hps.data.hop_length)
|
||||
)
|
||||
f0 = torch.from_numpy(f0.astype(np.float32))
|
||||
return f0
|
||||
|
||||
def _get_spec(self, newData: any):
|
||||
def _get_spec(self, newData: AudioInOut):
|
||||
audio = torch.FloatTensor(newData)
|
||||
audio_norm = audio.unsqueeze(0) # unsqueeze
|
||||
spec = spectrogram_torch(audio_norm, self.hps.data.filter_length,
|
||||
self.hps.data.sampling_rate, self.hps.data.hop_length, self.hps.data.win_length,
|
||||
center=False)
|
||||
spec = spectrogram_torch(
|
||||
audio_norm,
|
||||
self.hps.data.filter_length,
|
||||
self.hps.data.sampling_rate,
|
||||
self.hps.data.hop_length,
|
||||
self.hps.data.win_length,
|
||||
center=False,
|
||||
)
|
||||
spec = torch.squeeze(spec, 0)
|
||||
return spec
|
||||
|
||||
def generate_input(self, newData: any, inputSize: int, crossfadeSize: int, solaSearchFrame: int = 0):
|
||||
def generate_input(
|
||||
self,
|
||||
newData: AudioInOut,
|
||||
inputSize: int,
|
||||
crossfadeSize: int,
|
||||
solaSearchFrame: int = 0,
|
||||
):
|
||||
newData = newData.astype(np.float32) / self.hps.data.max_wav_value
|
||||
|
||||
if hasattr(self, "audio_buffer"):
|
||||
self.audio_buffer = np.concatenate([self.audio_buffer, newData], 0) # 過去のデータに連結
|
||||
if self.audio_buffer is not None:
|
||||
self.audio_buffer = np.concatenate(
|
||||
[self.audio_buffer, newData], 0
|
||||
) # 過去のデータに連結
|
||||
else:
|
||||
self.audio_buffer = newData
|
||||
|
||||
@ -178,13 +235,16 @@ class MMVCv15:
|
||||
if convertSize < 8192:
|
||||
convertSize = 8192
|
||||
if convertSize % self.hps.data.hop_length != 0: # モデルの出力のホップサイズで切り捨てが発生するので補う。
|
||||
convertSize = convertSize + (self.hps.data.hop_length - (convertSize % self.hps.data.hop_length))
|
||||
convertSize = convertSize + (
|
||||
self.hps.data.hop_length - (convertSize % self.hps.data.hop_length)
|
||||
)
|
||||
|
||||
# ONNX は固定長
|
||||
if self.settings.framework == "ONNX":
|
||||
convertSize = self.onxx_input_length
|
||||
|
||||
self.audio_buffer = self.audio_buffer[-1 * convertSize:] # 変換対象の部分だけ抽出
|
||||
convertOffset = -1 * convertSize
|
||||
self.audio_buffer = self.audio_buffer[convertOffset:] # 変換対象の部分だけ抽出
|
||||
|
||||
f0 = self._get_f0(self.settings.f0Detector, self.audio_buffer) # torch
|
||||
f0 = (f0 * self.settings.f0Factor).unsqueeze(0).unsqueeze(0)
|
||||
@ -193,7 +253,7 @@ class MMVCv15:
|
||||
return [spec, f0, sid]
|
||||
|
||||
def _onnx_inference(self, data):
|
||||
if self.settings.onnxModelFile == "" or self.settings.onnxModelFile == None:
|
||||
if self.settings.onnxModelFile == "" and self.settings.onnxModelFile is None:
|
||||
print("[Voice Changer] No ONNX session.")
|
||||
raise NoModeLoadedException("ONNX")
|
||||
|
||||
@ -203,7 +263,8 @@ class MMVCv15:
|
||||
sid_tgt1 = torch.LongTensor([self.settings.dstId])
|
||||
sin, d = self.net_g.make_sin_d(f0)
|
||||
(d0, d1, d2, d3) = d
|
||||
audio1 = self.onnx_session.run(
|
||||
audio1 = (
|
||||
self.onnx_session.run(
|
||||
["audio"],
|
||||
{
|
||||
"specs": spec.numpy(),
|
||||
@ -214,12 +275,18 @@ class MMVCv15:
|
||||
"d2": d2.numpy(),
|
||||
"d3": d3.numpy(),
|
||||
"sid_src": sid_src.numpy(),
|
||||
"sid_tgt": sid_tgt1.numpy()
|
||||
})[0][0, 0] * self.hps.data.max_wav_value
|
||||
"sid_tgt": sid_tgt1.numpy(),
|
||||
},
|
||||
)[0][0, 0]
|
||||
* self.hps.data.max_wav_value
|
||||
)
|
||||
return audio1
|
||||
|
||||
def _pyTorch_inference(self, data):
|
||||
if self.settings.pyTorchModelFile == "" or self.settings.pyTorchModelFile == None:
|
||||
if (
|
||||
self.settings.pyTorchModelFile == ""
|
||||
or self.settings.pyTorchModelFile is None
|
||||
):
|
||||
print("[Voice Changer] No pyTorch session.")
|
||||
raise NoModeLoadedException("pytorch")
|
||||
|
||||
@ -236,7 +303,12 @@ class MMVCv15:
|
||||
sid_src = sid_src.to(dev)
|
||||
sid_target = torch.LongTensor([self.settings.dstId]).to(dev)
|
||||
|
||||
audio1 = self.net_g.to(dev).voice_conversion(spec, spec_lengths, f0, sid_src, sid_target)[0, 0].data * self.hps.data.max_wav_value
|
||||
audio1 = (
|
||||
self.net_g.to(dev)
|
||||
.voice_conversion(spec, spec_lengths, f0, sid_src, sid_target)[0, 0]
|
||||
.data
|
||||
* self.hps.data.max_wav_value
|
||||
)
|
||||
result = audio1.float().cpu().numpy()
|
||||
return result
|
||||
|
||||
@ -256,7 +328,7 @@ class MMVCv15:
|
||||
del self.onnx_session
|
||||
|
||||
remove_path = os.path.join("MMVC_Client_v15", "python")
|
||||
sys.path = [x for x in sys.path if x.endswith(remove_path) == False]
|
||||
sys.path = [x for x in sys.path if x.endswith(remove_path) is False]
|
||||
|
||||
for key in list(sys.modules):
|
||||
val = sys.modules.get(key)
|
||||
@ -265,5 +337,5 @@ class MMVCv15:
|
||||
if file_path.find(remove_path + os.path.sep) >= 0:
|
||||
print("remove", key, file_path)
|
||||
sys.modules.pop(key)
|
||||
except Exception as e:
|
||||
except: # type:ignore
|
||||
pass
|
||||
|
17
server/voice_changer/RVC/ModelSlot.py
Normal file
17
server/voice_changer/RVC/ModelSlot.py
Normal file
@ -0,0 +1,17 @@
|
||||
from dataclasses import dataclass
|
||||
from voice_changer.RVC.const import RVC_MODEL_TYPE_RVC
|
||||
|
||||
|
||||
@dataclass
|
||||
class ModelSlot:
|
||||
pyTorchModelFile: str = ""
|
||||
onnxModelFile: str = ""
|
||||
featureFile: str = ""
|
||||
indexFile: str = ""
|
||||
defaultTrans: int = 0
|
||||
modelType: int = RVC_MODEL_TYPE_RVC
|
||||
samplingRate: int = -1
|
||||
f0: bool = True
|
||||
embChannels: int = 256
|
||||
deprecated: bool = False
|
||||
embedder: str = "hubert_base" # "hubert_base", "contentvec", "distilhubert"
|
@ -1,6 +1,8 @@
|
||||
import onnxruntime
|
||||
import torch
|
||||
import numpy as np
|
||||
import json
|
||||
|
||||
# providers = ['OpenVINOExecutionProvider', "CUDAExecutionProvider", "DmlExecutionProvider", "CPUExecutionProvider"]
|
||||
providers = ["CPUExecutionProvider"]
|
||||
|
||||
@ -12,8 +14,7 @@ class ModelWrapper:
|
||||
# ort_options = onnxruntime.SessionOptions()
|
||||
# ort_options.intra_op_num_threads = 8
|
||||
self.onnx_session = onnxruntime.InferenceSession(
|
||||
self.onnx_model,
|
||||
providers=providers
|
||||
self.onnx_model, providers=providers
|
||||
)
|
||||
# input_info = s
|
||||
first_input_type = self.onnx_session.get_inputs()[0].type
|
||||
@ -21,21 +22,89 @@ class ModelWrapper:
|
||||
self.is_half = False
|
||||
else:
|
||||
self.is_half = True
|
||||
modelmeta = self.onnx_session.get_modelmeta()
|
||||
try:
|
||||
metadata = json.loads(modelmeta.custom_metadata_map["metadata"])
|
||||
self.samplingRate = metadata["samplingRate"]
|
||||
self.f0 = metadata["f0"]
|
||||
self.embChannels = metadata["embChannels"]
|
||||
self.modelType = metadata["modelType"]
|
||||
self.deprecated = False
|
||||
self.embedder = (
|
||||
metadata["embedder"] if "embedder" in metadata else "hubert_base"
|
||||
)
|
||||
print(
|
||||
f"[Voice Changer] Onnx metadata: sr:{self.samplingRate}, f0:{self.f0}, embedder:{self.embedder}"
|
||||
)
|
||||
except:
|
||||
self.samplingRate = 48000
|
||||
self.f0 = True
|
||||
self.embChannels = 256
|
||||
self.modelType = 0
|
||||
self.deprecated = True
|
||||
self.embedder = "hubert_base"
|
||||
print(
|
||||
"[Voice Changer] ############## !!!! CAUTION !!!! ####################"
|
||||
)
|
||||
print(
|
||||
"[Voice Changer] This onnx's version is depricated. Please regenerate onnxfile. Fallback to default"
|
||||
)
|
||||
print(
|
||||
f"[Voice Changer] Onnx metadata: sr:{self.samplingRate}, f0:{self.f0}"
|
||||
)
|
||||
print(
|
||||
"[Voice Changer] ############## !!!! CAUTION !!!! ####################"
|
||||
)
|
||||
|
||||
def getSamplingRate(self):
|
||||
return self.samplingRate
|
||||
|
||||
def getF0(self):
|
||||
return self.f0
|
||||
|
||||
def getEmbChannels(self):
|
||||
return self.embChannels
|
||||
|
||||
def getModelType(self):
|
||||
return self.modelType
|
||||
|
||||
def getDeprecated(self):
|
||||
return self.deprecated
|
||||
|
||||
def getEmbedder(self):
|
||||
return self.embedder
|
||||
|
||||
def set_providers(self, providers, provider_options=[{}]):
|
||||
self.onnx_session.set_providers(providers=providers, provider_options=provider_options)
|
||||
self.onnx_session.set_providers(
|
||||
providers=providers, provider_options=provider_options
|
||||
)
|
||||
|
||||
def get_providers(self):
|
||||
return self.onnx_session.get_providers()
|
||||
|
||||
def infer_pitchless(self, feats, p_len, sid):
|
||||
if self.is_half:
|
||||
audio1 = self.onnx_session.run(
|
||||
["audio"],
|
||||
{
|
||||
"feats": feats.cpu().numpy().astype(np.float16),
|
||||
"p_len": p_len.cpu().numpy().astype(np.int64),
|
||||
"sid": sid.cpu().numpy().astype(np.int64),
|
||||
},
|
||||
)
|
||||
else:
|
||||
audio1 = self.onnx_session.run(
|
||||
["audio"],
|
||||
{
|
||||
"feats": feats.cpu().numpy().astype(np.float32),
|
||||
"p_len": p_len.cpu().numpy().astype(np.int64),
|
||||
"sid": sid.cpu().numpy().astype(np.int64),
|
||||
},
|
||||
)
|
||||
return torch.tensor(np.array(audio1))
|
||||
|
||||
def infer(self, feats, p_len, pitch, pitchf, sid):
|
||||
if self.is_half:
|
||||
# print("feats", feats.cpu().numpy().dtype)
|
||||
# print("p_len", p_len.cpu().numpy().dtype)
|
||||
# print("pitch", pitch.cpu().numpy().dtype)
|
||||
# print("pitchf", pitchf.cpu().numpy().dtype)
|
||||
# print("sid", sid.cpu().numpy().dtype)
|
||||
|
||||
audio1 = self.onnx_session.run(
|
||||
["audio"],
|
||||
{
|
||||
@ -44,7 +113,8 @@ class ModelWrapper:
|
||||
"pitch": pitch.cpu().numpy().astype(np.int64),
|
||||
"pitchf": pitchf.cpu().numpy().astype(np.float32),
|
||||
"sid": sid.cpu().numpy().astype(np.int64),
|
||||
})
|
||||
},
|
||||
)
|
||||
else:
|
||||
audio1 = self.onnx_session.run(
|
||||
["audio"],
|
||||
@ -54,6 +124,7 @@ class ModelWrapper:
|
||||
"pitch": pitch.cpu().numpy().astype(np.int64),
|
||||
"pitchf": pitchf.cpu().numpy().astype(np.float32),
|
||||
"sid": sid.cpu().numpy().astype(np.int64),
|
||||
})
|
||||
},
|
||||
)
|
||||
|
||||
return torch.tensor(np.array(audio1))
|
||||
|
@ -4,11 +4,27 @@ import json
|
||||
import resampy
|
||||
from voice_changer.RVC.ModelWrapper import ModelWrapper
|
||||
from Exceptions import NoModeLoadedException
|
||||
from voice_changer.RVC.RVCSettings import RVCSettings
|
||||
from voice_changer.utils.LoadModelParams import LoadModelParams
|
||||
from voice_changer.utils.VoiceChangerModel import AudioInOut
|
||||
from voice_changer.utils.VoiceChangerParams import VoiceChangerParams
|
||||
|
||||
from dataclasses import asdict
|
||||
from typing import cast
|
||||
import numpy as np
|
||||
import torch
|
||||
|
||||
from fairseq import checkpoint_utils
|
||||
import traceback
|
||||
import faiss
|
||||
|
||||
from const import TMP_DIR # type:ignore
|
||||
|
||||
|
||||
# avoiding parse arg error in RVC
|
||||
sys.argv = ["MMVCServerSIO.py"]
|
||||
|
||||
if sys.platform.startswith('darwin'):
|
||||
if sys.platform.startswith("darwin"):
|
||||
baseDir = [x for x in sys.path if x.endswith("Contents/MacOS")]
|
||||
if len(baseDir) != 1:
|
||||
print("baseDir should be only one ", baseDir)
|
||||
@ -18,112 +34,93 @@ if sys.platform.startswith('darwin'):
|
||||
else:
|
||||
sys.path.append("RVC")
|
||||
|
||||
import io
|
||||
from dataclasses import dataclass, asdict, field
|
||||
from functools import reduce
|
||||
import numpy as np
|
||||
import torch
|
||||
import onnxruntime
|
||||
# onnxruntime.set_default_logger_severity(3)
|
||||
from const import HUBERT_ONNX_MODEL_PATH, TMP_DIR
|
||||
|
||||
import pyworld as pw
|
||||
|
||||
from .models import SynthesizerTrnMsNSFsid as SynthesizerTrnMsNSFsid_webui
|
||||
from .models import SynthesizerTrnMsNSFsidNono as SynthesizerTrnMsNSFsidNono_webui
|
||||
from .const import RVC_MODEL_TYPE_RVC, RVC_MODEL_TYPE_WEBUI
|
||||
from voice_changer.RVC.custom_vc_infer_pipeline import VC
|
||||
from infer_pack.models import SynthesizerTrnMs256NSFsid
|
||||
from fairseq import checkpoint_utils
|
||||
providers = ['OpenVINOExecutionProvider', "CUDAExecutionProvider", "DmlExecutionProvider", "CPUExecutionProvider"]
|
||||
from infer_pack.models import ( # type:ignore
|
||||
SynthesizerTrnMs256NSFsid,
|
||||
SynthesizerTrnMs256NSFsid_nono,
|
||||
)
|
||||
|
||||
|
||||
@dataclass
|
||||
class ModelSlot():
|
||||
pyTorchModelFile: str = ""
|
||||
onnxModelFile: str = ""
|
||||
featureFile: str = ""
|
||||
indexFile: str = ""
|
||||
defaultTrans: int = ""
|
||||
|
||||
|
||||
@dataclass
|
||||
class RVCSettings():
|
||||
gpu: int = 0
|
||||
dstId: int = 0
|
||||
|
||||
f0Detector: str = "pm" # pm or harvest
|
||||
tran: int = 20
|
||||
silentThreshold: float = 0.00001
|
||||
extraConvertSize: int = 1024 * 32
|
||||
clusterInferRatio: float = 0.1
|
||||
|
||||
framework: str = "PyTorch" # PyTorch or ONNX
|
||||
pyTorchModelFile: str = ""
|
||||
onnxModelFile: str = ""
|
||||
configFile: str = ""
|
||||
modelSlots: list[ModelSlot] = field(
|
||||
default_factory=lambda: [
|
||||
ModelSlot(), ModelSlot(), ModelSlot()
|
||||
]
|
||||
)
|
||||
indexRatio: float = 0
|
||||
rvcQuality: int = 0
|
||||
silenceFront: int = 1 # 0:off, 1:on
|
||||
modelSamplingRate: int = 48000
|
||||
modelSlotIndex: int = 0
|
||||
|
||||
speakers: dict[str, int] = field(
|
||||
default_factory=lambda: {}
|
||||
)
|
||||
|
||||
# ↓mutableな物だけ列挙
|
||||
intData = ["gpu", "dstId", "tran", "extraConvertSize", "rvcQuality", "modelSamplingRate", "silenceFront", "modelSlotIndex"]
|
||||
floatData = ["silentThreshold", "indexRatio"]
|
||||
strData = ["framework", "f0Detector"]
|
||||
providers = [
|
||||
"OpenVINOExecutionProvider",
|
||||
"CUDAExecutionProvider",
|
||||
"DmlExecutionProvider",
|
||||
"CPUExecutionProvider",
|
||||
]
|
||||
|
||||
|
||||
class RVC:
|
||||
def __init__(self, params):
|
||||
audio_buffer: AudioInOut | None = None
|
||||
|
||||
def __init__(self, params: VoiceChangerParams):
|
||||
self.initialLoad = True
|
||||
self.settings = RVCSettings()
|
||||
|
||||
self.inferenceing: bool = False
|
||||
|
||||
self.net_g = None
|
||||
self.onnx_session = None
|
||||
self.feature_file = None
|
||||
self.index_file = None
|
||||
|
||||
# self.net_g2 = None
|
||||
# self.onnx_session2 = None
|
||||
# self.feature_file2 = None
|
||||
# self.index_file2 = None
|
||||
|
||||
self.gpu_num = torch.cuda.device_count()
|
||||
self.prevVol = 0
|
||||
self.params = params
|
||||
self.mps_enabled: bool = getattr(torch.backends, "mps", None) is not None and torch.backends.mps.is_available()
|
||||
|
||||
self.mps_enabled: bool = (
|
||||
getattr(torch.backends, "mps", None) is not None
|
||||
and torch.backends.mps.is_available()
|
||||
)
|
||||
self.currentSlot = -1
|
||||
print("RVC initialization: ", params)
|
||||
print("mps: ", self.mps_enabled)
|
||||
|
||||
def loadModel(self, props):
|
||||
self.is_half = props["isHalf"]
|
||||
self.tmp_slot = props["slot"]
|
||||
params_str = props["params"]
|
||||
def loadModel(self, props: LoadModelParams):
|
||||
"""
|
||||
loadModelはスロットへのエントリ(推論向けにはロードしない)。
|
||||
例外的に、まだ一つも推論向けにロードされていない場合は、ロードする。
|
||||
"""
|
||||
self.is_half = props.isHalf
|
||||
tmp_slot = props.slot
|
||||
params_str = props.params
|
||||
params = json.loads(params_str)
|
||||
|
||||
self.settings.modelSlots[self.tmp_slot] = ModelSlot(
|
||||
pyTorchModelFile=props["files"]["pyTorchModelFilename"],
|
||||
onnxModelFile=props["files"]["onnxModelFilename"],
|
||||
featureFile=props["files"]["featureFilename"],
|
||||
indexFile=props["files"]["indexFilename"],
|
||||
defaultTrans=params["trans"]
|
||||
self.settings.modelSlots[
|
||||
tmp_slot
|
||||
].pyTorchModelFile = props.files.pyTorchModelFilename
|
||||
self.settings.modelSlots[tmp_slot].onnxModelFile = props.files.onnxModelFilename
|
||||
self.settings.modelSlots[tmp_slot].featureFile = props.files.featureFilename
|
||||
self.settings.modelSlots[tmp_slot].indexFile = props.files.indexFilename
|
||||
self.settings.modelSlots[tmp_slot].defaultTrans = params["trans"]
|
||||
|
||||
isONNX = (
|
||||
True
|
||||
if self.settings.modelSlots[tmp_slot].onnxModelFile is not None
|
||||
else False
|
||||
)
|
||||
|
||||
print("[Voice Changer] RVC loading... slot:", self.tmp_slot)
|
||||
# メタデータ設定
|
||||
if isONNX:
|
||||
self._setInfoByONNX(
|
||||
tmp_slot, self.settings.modelSlots[tmp_slot].onnxModelFile
|
||||
)
|
||||
else:
|
||||
self._setInfoByPytorch(
|
||||
tmp_slot, self.settings.modelSlots[tmp_slot].pyTorchModelFile
|
||||
)
|
||||
|
||||
print(
|
||||
f"[Voice Changer] RVC loading... slot:{tmp_slot}",
|
||||
asdict(self.settings.modelSlots[tmp_slot]),
|
||||
)
|
||||
# hubertロード
|
||||
try:
|
||||
hubert_path = self.params["hubert_base"]
|
||||
models, saved_cfg, task = checkpoint_utils.load_model_ensemble_and_task([hubert_path], suffix="",)
|
||||
hubert_path = self.params.hubert_base
|
||||
models, saved_cfg, task = checkpoint_utils.load_model_ensemble_and_task(
|
||||
[hubert_path],
|
||||
suffix="",
|
||||
)
|
||||
model = models[0]
|
||||
model.eval()
|
||||
if self.is_half:
|
||||
@ -133,85 +130,194 @@ class RVC:
|
||||
except Exception as e:
|
||||
print("EXCEPTION during loading hubert/contentvec model", e)
|
||||
|
||||
# self.switchModel(self.slot)
|
||||
if self.initialLoad:
|
||||
self.prepareModel(self.tmp_slot)
|
||||
self.slot = self.tmp_slot
|
||||
self.currentSlot = self.slot
|
||||
# 初回のみロード
|
||||
if self.initialLoad or tmp_slot == self.currentSlot:
|
||||
self.prepareModel(tmp_slot)
|
||||
self.settings.modelSlotIndex = tmp_slot
|
||||
self.currentSlot = self.settings.modelSlotIndex
|
||||
self.switchModel()
|
||||
self.initialLoad = False
|
||||
|
||||
return self.get_info()
|
||||
|
||||
def _setInfoByPytorch(self, slot, file):
|
||||
cpt = torch.load(file, map_location="cpu")
|
||||
config_len = len(cpt["config"])
|
||||
if config_len == 18:
|
||||
self.settings.modelSlots[slot].modelType = RVC_MODEL_TYPE_RVC
|
||||
self.settings.modelSlots[slot].embChannels = 256
|
||||
self.settings.modelSlots[slot].embedder = "hubert_base"
|
||||
else:
|
||||
self.settings.modelSlots[slot].modelType = RVC_MODEL_TYPE_WEBUI
|
||||
self.settings.modelSlots[slot].embChannels = cpt["config"][17]
|
||||
self.settings.modelSlots[slot].embedder = cpt["embedder_name"]
|
||||
if self.settings.modelSlots[slot].embedder.endswith("768"):
|
||||
self.settings.modelSlots[slot].embedder = self.settings.modelSlots[
|
||||
slot
|
||||
].embedder[:-3]
|
||||
|
||||
self.settings.modelSlots[slot].f0 = True if cpt["f0"] == 1 else False
|
||||
self.settings.modelSlots[slot].samplingRate = cpt["config"][-1]
|
||||
|
||||
# self.settings.modelSamplingRate = cpt["config"][-1]
|
||||
|
||||
def _setInfoByONNX(self, slot, file):
|
||||
tmp_onnx_session = ModelWrapper(file)
|
||||
self.settings.modelSlots[slot].modelType = tmp_onnx_session.getModelType()
|
||||
self.settings.modelSlots[slot].embChannels = tmp_onnx_session.getEmbChannels()
|
||||
self.settings.modelSlots[slot].embedder = tmp_onnx_session.getEmbedder()
|
||||
self.settings.modelSlots[slot].f0 = tmp_onnx_session.getF0()
|
||||
self.settings.modelSlots[slot].samplingRate = tmp_onnx_session.getSamplingRate()
|
||||
self.settings.modelSlots[slot].deprecated = tmp_onnx_session.getDeprecated()
|
||||
|
||||
def prepareModel(self, slot: int):
|
||||
if slot < 0:
|
||||
return self.get_info()
|
||||
print("[Voice Changer] Prepare Model of slot:", slot)
|
||||
pyTorchModelFile = self.settings.modelSlots[slot].pyTorchModelFile
|
||||
onnxModelFile = self.settings.modelSlots[slot].onnxModelFile
|
||||
# PyTorchモデル生成
|
||||
if pyTorchModelFile != None and pyTorchModelFile != "":
|
||||
cpt = torch.load(pyTorchModelFile, map_location="cpu")
|
||||
self.settings.modelSamplingRate = cpt["config"][-1]
|
||||
isONNX = (
|
||||
True if self.settings.modelSlots[slot].onnxModelFile is not None else False
|
||||
)
|
||||
|
||||
# モデルのロード
|
||||
if isONNX:
|
||||
print("[Voice Changer] Loading ONNX Model...")
|
||||
self.next_onnx_session = ModelWrapper(onnxModelFile)
|
||||
self.next_net_g = None
|
||||
else:
|
||||
print("[Voice Changer] Loading Pytorch Model...")
|
||||
torchModelSlot = self.settings.modelSlots[slot]
|
||||
cpt = torch.load(torchModelSlot.pyTorchModelFile, map_location="cpu")
|
||||
|
||||
if (
|
||||
torchModelSlot.modelType == RVC_MODEL_TYPE_RVC
|
||||
and torchModelSlot.f0 is True
|
||||
):
|
||||
net_g = SynthesizerTrnMs256NSFsid(*cpt["config"], is_half=self.is_half)
|
||||
elif (
|
||||
torchModelSlot.modelType == RVC_MODEL_TYPE_RVC
|
||||
and torchModelSlot.f0 is False
|
||||
):
|
||||
net_g = SynthesizerTrnMs256NSFsid_nono(*cpt["config"])
|
||||
elif (
|
||||
torchModelSlot.modelType == RVC_MODEL_TYPE_WEBUI
|
||||
and torchModelSlot.f0 is True
|
||||
):
|
||||
net_g = SynthesizerTrnMsNSFsid_webui(
|
||||
**cpt["params"], is_half=self.is_half
|
||||
)
|
||||
else:
|
||||
net_g = SynthesizerTrnMsNSFsidNono_webui(
|
||||
**cpt["params"], is_half=self.is_half
|
||||
)
|
||||
net_g.eval()
|
||||
net_g.load_state_dict(cpt["weight"], strict=False)
|
||||
|
||||
if self.is_half:
|
||||
net_g = net_g.half()
|
||||
self.next_net_g = net_g
|
||||
else:
|
||||
self.next_net_g = None
|
||||
|
||||
# ONNXモデル生成
|
||||
if onnxModelFile != None and onnxModelFile != "":
|
||||
self.next_onnx_session = ModelWrapper(onnxModelFile)
|
||||
else:
|
||||
self.next_net_g = net_g
|
||||
self.next_onnx_session = None
|
||||
|
||||
# Indexのロード
|
||||
print("[Voice Changer] Loading index...")
|
||||
self.next_feature_file = self.settings.modelSlots[slot].featureFile
|
||||
self.next_index_file = self.settings.modelSlots[slot].indexFile
|
||||
self.next_trans = self.settings.modelSlots[slot].defaultTrans
|
||||
|
||||
if (
|
||||
self.settings.modelSlots[slot].featureFile is not None
|
||||
and self.settings.modelSlots[slot].indexFile is not None
|
||||
):
|
||||
if (
|
||||
os.path.exists(self.settings.modelSlots[slot].featureFile) is True
|
||||
and os.path.exists(self.settings.modelSlots[slot].indexFile) is True
|
||||
):
|
||||
try:
|
||||
self.next_index = faiss.read_index(
|
||||
self.settings.modelSlots[slot].indexFile
|
||||
)
|
||||
self.next_feature = np.load(
|
||||
self.settings.modelSlots[slot].featureFile
|
||||
)
|
||||
except:
|
||||
print("[Voice Changer] load index failed. Use no index.")
|
||||
traceback.print_exc()
|
||||
self.next_index = self.next_feature = None
|
||||
else:
|
||||
print("[Voice Changer] Index file is not found. Use no index.")
|
||||
self.next_index = self.next_feature = None
|
||||
else:
|
||||
self.next_index = self.next_feature = None
|
||||
|
||||
self.next_trans = self.settings.modelSlots[slot].defaultTrans
|
||||
self.next_samplingRate = self.settings.modelSlots[slot].samplingRate
|
||||
self.next_framework = (
|
||||
"ONNX" if self.next_onnx_session is not None else "PyTorch"
|
||||
)
|
||||
print("[Voice Changer] Prepare done.")
|
||||
return self.get_info()
|
||||
|
||||
def switchModel(self):
|
||||
print("[Voice Changer] Switching model..")
|
||||
# del self.net_g
|
||||
# del self.onnx_session
|
||||
self.net_g = self.next_net_g
|
||||
self.onnx_session = self.next_onnx_session
|
||||
self.feature_file = self.next_feature_file
|
||||
self.index_file = self.next_index_file
|
||||
self.feature = self.next_feature
|
||||
self.index = self.next_index
|
||||
self.settings.tran = self.next_trans
|
||||
self.settings.framework = self.next_framework
|
||||
self.settings.modelSamplingRate = self.next_samplingRate
|
||||
self.next_net_g = None
|
||||
self.next_onnx_session = None
|
||||
print(
|
||||
"[Voice Changer] Switching model..done",
|
||||
)
|
||||
|
||||
def update_settings(self, key: str, val: any):
|
||||
if key == "onnxExecutionProvider" and self.onnx_session != None:
|
||||
def update_settings(self, key: str, val: int | float | str):
|
||||
if key == "onnxExecutionProvider" and self.onnx_session is not None:
|
||||
if val == "CUDAExecutionProvider":
|
||||
if self.settings.gpu < 0 or self.settings.gpu >= self.gpu_num:
|
||||
self.settings.gpu = 0
|
||||
provider_options = [{'device_id': self.settings.gpu}]
|
||||
self.onnx_session.set_providers(providers=[val], provider_options=provider_options)
|
||||
provider_options = [{"device_id": self.settings.gpu}]
|
||||
self.onnx_session.set_providers(
|
||||
providers=[val], provider_options=provider_options
|
||||
)
|
||||
if hasattr(self, "hubert_onnx"):
|
||||
self.hubert_onnx.set_providers(providers=[val], provider_options=provider_options)
|
||||
self.hubert_onnx.set_providers(
|
||||
providers=[val], provider_options=provider_options
|
||||
)
|
||||
else:
|
||||
self.onnx_session.set_providers(providers=[val])
|
||||
if hasattr(self, "hubert_onnx"):
|
||||
self.hubert_onnx.set_providers(providers=[val])
|
||||
elif key == "onnxExecutionProvider" and self.onnx_session == None:
|
||||
elif key == "onnxExecutionProvider" and self.onnx_session is None:
|
||||
print("Onnx is not enabled. Please load model.")
|
||||
return False
|
||||
elif key in self.settings.intData:
|
||||
setattr(self.settings, key, int(val))
|
||||
if key == "gpu" and val >= 0 and val < self.gpu_num and self.onnx_session != None:
|
||||
val = cast(int, val)
|
||||
if (
|
||||
key == "gpu"
|
||||
and val >= 0
|
||||
and val < self.gpu_num
|
||||
and self.onnx_session is not None
|
||||
):
|
||||
providers = self.onnx_session.get_providers()
|
||||
print("Providers:", providers)
|
||||
if "CUDAExecutionProvider" in providers:
|
||||
provider_options = [{'device_id': self.settings.gpu}]
|
||||
self.onnx_session.set_providers(providers=["CUDAExecutionProvider"], provider_options=provider_options)
|
||||
provider_options = [{"device_id": self.settings.gpu}]
|
||||
self.onnx_session.set_providers(
|
||||
providers=["CUDAExecutionProvider"],
|
||||
provider_options=provider_options,
|
||||
)
|
||||
if key == "modelSlotIndex":
|
||||
# self.switchModel(int(val))
|
||||
self.tmp_slot = int(val)
|
||||
self.prepareModel(self.tmp_slot)
|
||||
self.slot = self.tmp_slot
|
||||
val = int(val) % 1000 # Quick hack for same slot is selected
|
||||
self.prepareModel(val)
|
||||
self.currentSlot = -1
|
||||
setattr(self.settings, key, int(val))
|
||||
elif key in self.settings.floatData:
|
||||
setattr(self.settings, key, float(val))
|
||||
elif key in self.settings.strData:
|
||||
@ -224,10 +330,12 @@ class RVC:
|
||||
def get_info(self):
|
||||
data = asdict(self.settings)
|
||||
|
||||
data["onnxExecutionProviders"] = self.onnx_session.get_providers() if self.onnx_session != None else []
|
||||
data["onnxExecutionProviders"] = (
|
||||
self.onnx_session.get_providers() if self.onnx_session is not None else []
|
||||
)
|
||||
files = ["configFile", "pyTorchModelFile", "onnxModelFile"]
|
||||
for f in files:
|
||||
if data[f] != None and os.path.exists(data[f]):
|
||||
if data[f] is not None and os.path.exists(data[f]):
|
||||
data[f] = os.path.basename(data[f])
|
||||
else:
|
||||
data[f] = ""
|
||||
@ -237,22 +345,35 @@ class RVC:
|
||||
def get_processing_sampling_rate(self):
|
||||
return self.settings.modelSamplingRate
|
||||
|
||||
def generate_input(self, newData: any, inputSize: int, crossfadeSize: int, solaSearchFrame: int = 0):
|
||||
def generate_input(
|
||||
self,
|
||||
newData: AudioInOut,
|
||||
inputSize: int,
|
||||
crossfadeSize: int,
|
||||
solaSearchFrame: int = 0,
|
||||
):
|
||||
newData = newData.astype(np.float32) / 32768.0
|
||||
|
||||
if hasattr(self, "audio_buffer"):
|
||||
self.audio_buffer = np.concatenate([self.audio_buffer, newData], 0) # 過去のデータに連結
|
||||
if self.audio_buffer is not None:
|
||||
# 過去のデータに連結
|
||||
self.audio_buffer = np.concatenate([self.audio_buffer, newData], 0)
|
||||
else:
|
||||
self.audio_buffer = newData
|
||||
|
||||
convertSize = inputSize + crossfadeSize + solaSearchFrame + self.settings.extraConvertSize
|
||||
convertSize = (
|
||||
inputSize + crossfadeSize + solaSearchFrame + self.settings.extraConvertSize
|
||||
)
|
||||
|
||||
if convertSize % 128 != 0: # モデルの出力のホップサイズで切り捨てが発生するので補う。
|
||||
convertSize = convertSize + (128 - (convertSize % 128))
|
||||
|
||||
self.audio_buffer = self.audio_buffer[-1 * convertSize:] # 変換対象の部分だけ抽出
|
||||
convertOffset = -1 * convertSize
|
||||
self.audio_buffer = self.audio_buffer[convertOffset:] # 変換対象の部分だけ抽出
|
||||
|
||||
crop = self.audio_buffer[-1 * (inputSize + crossfadeSize):-1 * (crossfadeSize)] # 出力部分だけ切り出して音量を確認。(solaとの関係性について、現状は無考慮)
|
||||
# 出力部分だけ切り出して音量を確認。(TODO:段階的消音にする)
|
||||
cropOffset = -1 * (inputSize + crossfadeSize)
|
||||
cropEnd = -1 * (crossfadeSize)
|
||||
crop = self.audio_buffer[cropOffset:cropEnd]
|
||||
rms = np.sqrt(np.square(crop).mean(axis=0))
|
||||
vol = max(rms, self.prevVol * 0.0)
|
||||
self.prevVol = vol
|
||||
@ -260,7 +381,7 @@ class RVC:
|
||||
return (self.audio_buffer, convertSize, vol)
|
||||
|
||||
def _onnx_inference(self, data):
|
||||
if hasattr(self, "onnx_session") == False or self.onnx_session == None:
|
||||
if hasattr(self, "onnx_session") is False or self.onnx_session is None:
|
||||
print("[Voice Changer] No onnx session.")
|
||||
raise NoModeLoadedException("ONNX")
|
||||
|
||||
@ -285,41 +406,54 @@ class RVC:
|
||||
repeat *= self.settings.rvcQuality # 0 or 3
|
||||
vc = VC(self.settings.modelSamplingRate, dev, self.is_half, repeat)
|
||||
sid = 0
|
||||
times = [0, 0, 0]
|
||||
f0_up_key = self.settings.tran
|
||||
f0_method = self.settings.f0Detector
|
||||
file_index = self.index_file if self.index_file != None else ""
|
||||
file_big_npy = self.feature_file if self.feature_file != None else ""
|
||||
index_rate = self.settings.indexRatio
|
||||
if_f0 = 1
|
||||
f0_file = None
|
||||
if_f0 = 1 if self.settings.modelSlots[self.currentSlot].f0 else 0
|
||||
|
||||
audio_out = vc.pipeline(self.hubert_model, self.onnx_session, sid, audio, times, f0_up_key, f0_method,
|
||||
file_index, file_big_npy, index_rate, if_f0, f0_file=f0_file)
|
||||
embChannels = self.settings.modelSlots[self.currentSlot].embChannels
|
||||
audio_out = vc.pipeline(
|
||||
self.hubert_model,
|
||||
self.onnx_session,
|
||||
sid,
|
||||
audio,
|
||||
f0_up_key,
|
||||
f0_method,
|
||||
self.index,
|
||||
self.feature,
|
||||
index_rate,
|
||||
if_f0,
|
||||
silence_front=self.settings.extraConvertSize
|
||||
/ self.settings.modelSamplingRate,
|
||||
embChannels=embChannels,
|
||||
)
|
||||
result = audio_out * np.sqrt(vol)
|
||||
|
||||
return result
|
||||
|
||||
def _pyTorch_inference(self, data):
|
||||
if hasattr(self, "net_g") == False or self.net_g == None:
|
||||
print("[Voice Changer] No pyTorch session.")
|
||||
if hasattr(self, "net_g") is False or self.net_g is None:
|
||||
print(
|
||||
"[Voice Changer] No pyTorch session.",
|
||||
hasattr(self, "net_g"),
|
||||
self.net_g,
|
||||
)
|
||||
raise NoModeLoadedException("pytorch")
|
||||
|
||||
if self.settings.gpu < 0 or (self.gpu_num == 0 and self.mps_enabled == False):
|
||||
if self.settings.gpu < 0 or (self.gpu_num == 0 and self.mps_enabled is False):
|
||||
dev = torch.device("cpu")
|
||||
elif self.mps_enabled:
|
||||
dev = torch.device("mps")
|
||||
else:
|
||||
dev = torch.device("cuda", index=self.settings.gpu)
|
||||
|
||||
# print("device:", dev)
|
||||
|
||||
self.hubert_model = self.hubert_model.to(dev)
|
||||
self.net_g = self.net_g.to(dev)
|
||||
|
||||
audio = data[0]
|
||||
convertSize = data[1]
|
||||
vol = data[2]
|
||||
|
||||
audio = resampy.resample(audio, self.settings.modelSamplingRate, 16000)
|
||||
|
||||
if vol < self.settings.silentThreshold:
|
||||
@ -330,29 +464,44 @@ class RVC:
|
||||
repeat *= self.settings.rvcQuality # 0 or 3
|
||||
vc = VC(self.settings.modelSamplingRate, dev, self.is_half, repeat)
|
||||
sid = 0
|
||||
times = [0, 0, 0]
|
||||
f0_up_key = self.settings.tran
|
||||
f0_method = self.settings.f0Detector
|
||||
file_index = self.index_file if self.index_file != None else ""
|
||||
file_big_npy = self.feature_file if self.feature_file != None else ""
|
||||
index_rate = self.settings.indexRatio
|
||||
if_f0 = 1
|
||||
f0_file = None
|
||||
if_f0 = 1 if self.settings.modelSlots[self.currentSlot].f0 else 0
|
||||
|
||||
if self.settings.silenceFront == 0:
|
||||
audio_out = vc.pipeline(self.hubert_model, self.net_g, sid, audio, times, f0_up_key, f0_method,
|
||||
file_index, file_big_npy, index_rate, if_f0, f0_file=f0_file, silence_front=0)
|
||||
else:
|
||||
audio_out = vc.pipeline(self.hubert_model, self.net_g, sid, audio, times, f0_up_key, f0_method,
|
||||
file_index, file_big_npy, index_rate, if_f0, f0_file=f0_file, silence_front=self.settings.extraConvertSize / self.settings.modelSamplingRate)
|
||||
embChannels = self.settings.modelSlots[self.currentSlot].embChannels
|
||||
audio_out = vc.pipeline(
|
||||
self.hubert_model,
|
||||
self.net_g,
|
||||
sid,
|
||||
audio,
|
||||
f0_up_key,
|
||||
f0_method,
|
||||
self.index,
|
||||
self.feature,
|
||||
index_rate,
|
||||
if_f0,
|
||||
silence_front=self.settings.extraConvertSize
|
||||
/ self.settings.modelSamplingRate,
|
||||
embChannels=embChannels,
|
||||
)
|
||||
|
||||
result = audio_out * np.sqrt(vol)
|
||||
|
||||
return result
|
||||
|
||||
def inference(self, data):
|
||||
if self.currentSlot != self.slot:
|
||||
self.currentSlot = self.slot
|
||||
if self.settings.modelSlotIndex < 0:
|
||||
print(
|
||||
"[Voice Changer] wait for loading model...",
|
||||
self.settings.modelSlotIndex,
|
||||
self.currentSlot,
|
||||
)
|
||||
raise NoModeLoadedException("model_common")
|
||||
|
||||
if self.currentSlot != self.settings.modelSlotIndex:
|
||||
print(f"Switch model {self.currentSlot} -> {self.settings.modelSlotIndex}")
|
||||
self.currentSlot = self.settings.modelSlotIndex
|
||||
self.switchModel()
|
||||
|
||||
if self.settings.framework == "ONNX":
|
||||
@ -367,7 +516,7 @@ class RVC:
|
||||
del self.onnx_session
|
||||
|
||||
remove_path = os.path.join("RVC")
|
||||
sys.path = [x for x in sys.path if x.endswith(remove_path) == False]
|
||||
sys.path = [x for x in sys.path if x.endswith(remove_path) is False]
|
||||
|
||||
for key in list(sys.modules):
|
||||
val = sys.modules.get(key)
|
||||
@ -377,29 +526,63 @@ class RVC:
|
||||
print("remove", key, file_path)
|
||||
sys.modules.pop(key)
|
||||
except Exception as e:
|
||||
print(e)
|
||||
pass
|
||||
|
||||
def export2onnx(self):
|
||||
if hasattr(self, "net_g") == False or self.net_g == None:
|
||||
if hasattr(self, "net_g") is False or self.net_g is None:
|
||||
print("[Voice Changer] export2onnx, No pyTorch session.")
|
||||
return {"status": "ng", "path": f""}
|
||||
return {"status": "ng", "path": ""}
|
||||
|
||||
pyTorchModelFile = self.settings.modelSlots[self.slot].pyTorchModelFile # inference前にexportできるようにcurrentSlotではなくslot
|
||||
pyTorchModelFile = self.settings.modelSlots[
|
||||
self.settings.modelSlotIndex
|
||||
].pyTorchModelFile # inference前にexportできるようにcurrentSlotではなくslot
|
||||
|
||||
if pyTorchModelFile == None:
|
||||
if pyTorchModelFile is None:
|
||||
print("[Voice Changer] export2onnx, No pyTorch filepath.")
|
||||
return {"status": "ng", "path": f""}
|
||||
return {"status": "ng", "path": ""}
|
||||
import voice_changer.RVC.export2onnx as onnxExporter
|
||||
|
||||
output_file = os.path.splitext(os.path.basename(pyTorchModelFile))[0] + ".onnx"
|
||||
output_file_simple = os.path.splitext(os.path.basename(pyTorchModelFile))[0] + "_simple.onnx"
|
||||
output_file_simple = (
|
||||
os.path.splitext(os.path.basename(pyTorchModelFile))[0] + "_simple.onnx"
|
||||
)
|
||||
output_path = os.path.join(TMP_DIR, output_file)
|
||||
output_path_simple = os.path.join(TMP_DIR, output_file_simple)
|
||||
print(
|
||||
"embChannels",
|
||||
self.settings.modelSlots[self.settings.modelSlotIndex].embChannels,
|
||||
)
|
||||
metadata = {
|
||||
"application": "VC_CLIENT",
|
||||
"version": "1",
|
||||
"modelType": self.settings.modelSlots[
|
||||
self.settings.modelSlotIndex
|
||||
].modelType,
|
||||
"samplingRate": self.settings.modelSlots[
|
||||
self.settings.modelSlotIndex
|
||||
].samplingRate,
|
||||
"f0": self.settings.modelSlots[self.settings.modelSlotIndex].f0,
|
||||
"embChannels": self.settings.modelSlots[
|
||||
self.settings.modelSlotIndex
|
||||
].embChannels,
|
||||
"embedder": self.settings.modelSlots[self.settings.modelSlotIndex].embedder,
|
||||
}
|
||||
|
||||
if torch.cuda.device_count() > 0:
|
||||
onnxExporter.export2onnx(pyTorchModelFile, output_path, output_path_simple, True)
|
||||
onnxExporter.export2onnx(
|
||||
pyTorchModelFile, output_path, output_path_simple, True, metadata
|
||||
)
|
||||
else:
|
||||
print("[Voice Changer] Warning!!! onnx export with float32. maybe size is doubled.")
|
||||
onnxExporter.export2onnx(pyTorchModelFile, output_path, output_path_simple, False)
|
||||
print(
|
||||
"[Voice Changer] Warning!!! onnx export with float32. maybe size is doubled."
|
||||
)
|
||||
onnxExporter.export2onnx(
|
||||
pyTorchModelFile, output_path, output_path_simple, False, metadata
|
||||
)
|
||||
|
||||
return {"status": "ok", "path": f"/tmp/{output_file_simple}", "filename": output_file_simple}
|
||||
return {
|
||||
"status": "ok",
|
||||
"path": f"/tmp/{output_file_simple}",
|
||||
"filename": output_file_simple,
|
||||
}
|
||||
|
44
server/voice_changer/RVC/RVCSettings.py
Normal file
44
server/voice_changer/RVC/RVCSettings.py
Normal file
@ -0,0 +1,44 @@
|
||||
from dataclasses import dataclass, field
|
||||
|
||||
from voice_changer.RVC.ModelSlot import ModelSlot
|
||||
|
||||
|
||||
@dataclass
|
||||
class RVCSettings:
|
||||
gpu: int = 0
|
||||
dstId: int = 0
|
||||
|
||||
f0Detector: str = "pm" # pm or harvest
|
||||
tran: int = 20
|
||||
silentThreshold: float = 0.00001
|
||||
extraConvertSize: int = 1024 * 32
|
||||
clusterInferRatio: float = 0.1
|
||||
|
||||
framework: str = "PyTorch" # PyTorch or ONNX
|
||||
pyTorchModelFile: str = ""
|
||||
onnxModelFile: str = ""
|
||||
configFile: str = ""
|
||||
modelSlots: list[ModelSlot] = field(
|
||||
default_factory=lambda: [ModelSlot(), ModelSlot(), ModelSlot()]
|
||||
)
|
||||
indexRatio: float = 0
|
||||
rvcQuality: int = 0
|
||||
silenceFront: int = 1 # 0:off, 1:on
|
||||
modelSamplingRate: int = 48000
|
||||
modelSlotIndex: int = -1
|
||||
|
||||
speakers: dict[str, int] = field(default_factory=lambda: {})
|
||||
|
||||
# ↓mutableな物だけ列挙
|
||||
intData = [
|
||||
"gpu",
|
||||
"dstId",
|
||||
"tran",
|
||||
"extraConvertSize",
|
||||
"rvcQuality",
|
||||
"modelSamplingRate",
|
||||
"silenceFront",
|
||||
"modelSlotIndex",
|
||||
]
|
||||
floatData = ["silentThreshold", "indexRatio"]
|
||||
strData = ["framework", "f0Detector"]
|
2
server/voice_changer/RVC/const.py
Normal file
2
server/voice_changer/RVC/const.py
Normal file
@ -0,0 +1,2 @@
|
||||
RVC_MODEL_TYPE_RVC = 0
|
||||
RVC_MODEL_TYPE_WEBUI = 1
|
@ -1,15 +1,11 @@
|
||||
import numpy as np
|
||||
import parselmouth
|
||||
|
||||
# import parselmouth
|
||||
import torch
|
||||
import pdb
|
||||
from time import time as ttime
|
||||
import torch.nn.functional as F
|
||||
from config import x_pad, x_query, x_center, x_max
|
||||
from config import x_query, x_center, x_max # type:ignore
|
||||
import scipy.signal as signal
|
||||
import pyworld
|
||||
import os
|
||||
import traceback
|
||||
import faiss
|
||||
|
||||
|
||||
class VC(object):
|
||||
@ -18,34 +14,27 @@ class VC(object):
|
||||
self.window = 160 # 每帧点数
|
||||
self.t_pad = self.sr * x_pad # 每条前后pad时间
|
||||
self.t_pad_tgt = tgt_sr * x_pad
|
||||
self.t_pad2 = self.t_pad * 2
|
||||
self.t_query = self.sr * x_query # 查询切点前后查询时间
|
||||
self.t_center = self.sr * x_center # 查询切点位置
|
||||
self.t_max = self.sr * x_max # 免查询时长阈值
|
||||
self.device = device
|
||||
self.is_half = is_half
|
||||
|
||||
def get_f0(self, audio, p_len, f0_up_key, f0_method, inp_f0=None, silence_front=0):
|
||||
|
||||
def get_f0(self, audio, p_len, f0_up_key, f0_method, silence_front=0):
|
||||
n_frames = int(len(audio) // self.window) + 1
|
||||
start_frame = int(silence_front * self.sr / self.window)
|
||||
real_silence_front = start_frame * self.window / self.sr
|
||||
|
||||
audio = audio[int(np.round(real_silence_front * self.sr)):]
|
||||
silence_front_offset = int(np.round(real_silence_front * self.sr))
|
||||
audio = audio[silence_front_offset:]
|
||||
|
||||
time_step = self.window / self.sr * 1000
|
||||
# time_step = self.window / self.sr * 1000
|
||||
f0_min = 50
|
||||
f0_max = 1100
|
||||
f0_mel_min = 1127 * np.log(1 + f0_min / 700)
|
||||
f0_mel_max = 1127 * np.log(1 + f0_max / 700)
|
||||
if (f0_method == "pm"):
|
||||
f0 = parselmouth.Sound(audio, self.sr).to_pitch_ac(
|
||||
time_step=time_step / 1000, voicing_threshold=0.6,
|
||||
pitch_floor=f0_min, pitch_ceiling=f0_max).selected_array['frequency']
|
||||
pad_size = (p_len - len(f0) + 1) // 2
|
||||
if (pad_size > 0 or p_len - len(f0) - pad_size > 0):
|
||||
f0 = np.pad(f0, [[pad_size, p_len - len(f0) - pad_size]], mode='constant')
|
||||
elif (f0_method == "harvest"):
|
||||
if f0_method == "pm":
|
||||
print("not implemented. use harvest")
|
||||
f0, t = pyworld.harvest(
|
||||
audio.astype(np.double),
|
||||
fs=self.sr,
|
||||
@ -55,36 +44,98 @@ class VC(object):
|
||||
f0 = pyworld.stonemask(audio.astype(np.double), f0, t, self.sr)
|
||||
f0 = signal.medfilt(f0, 3)
|
||||
|
||||
f0 = np.pad(f0.astype('float'), (start_frame, n_frames - len(f0) - start_frame))
|
||||
f0 = np.pad(
|
||||
f0.astype("float"), (start_frame, n_frames - len(f0) - start_frame)
|
||||
)
|
||||
else:
|
||||
print("[Voice Changer] invalid f0 detector, use pm.", f0_method)
|
||||
f0 = parselmouth.Sound(audio, self.sr).to_pitch_ac(
|
||||
time_step=time_step / 1000, voicing_threshold=0.6,
|
||||
pitch_floor=f0_min, pitch_ceiling=f0_max).selected_array['frequency']
|
||||
pad_size = (p_len - len(f0) + 1) // 2
|
||||
if (pad_size > 0 or p_len - len(f0) - pad_size > 0):
|
||||
f0 = np.pad(f0, [[pad_size, p_len - len(f0) - pad_size]], mode='constant')
|
||||
f0, t = pyworld.harvest(
|
||||
audio.astype(np.double),
|
||||
fs=self.sr,
|
||||
f0_ceil=f0_max,
|
||||
frame_period=10,
|
||||
)
|
||||
f0 = pyworld.stonemask(audio.astype(np.double), f0, t, self.sr)
|
||||
f0 = signal.medfilt(f0, 3)
|
||||
|
||||
f0 = np.pad(
|
||||
f0.astype("float"), (start_frame, n_frames - len(f0) - start_frame)
|
||||
)
|
||||
|
||||
f0 *= pow(2, f0_up_key / 12)
|
||||
# with open("test.txt","w")as f:f.write("\n".join([str(i)for i in f0.tolist()]))
|
||||
tf0 = self.sr // self.window # 每秒f0点数
|
||||
if (inp_f0 is not None):
|
||||
delta_t = np.round((inp_f0[:, 0].max() - inp_f0[:, 0].min()) * tf0 + 1).astype("int16")
|
||||
replace_f0 = np.interp(list(range(delta_t)), inp_f0[:, 0] * 100, inp_f0[:, 1])
|
||||
shape = f0[x_pad * tf0:x_pad * tf0 + len(replace_f0)].shape[0]
|
||||
f0[x_pad * tf0:x_pad * tf0 + len(replace_f0)] = replace_f0[:shape]
|
||||
# with open("test_opt.txt","w")as f:f.write("\n".join([str(i)for i in f0.tolist()]))
|
||||
f0bak = f0.copy()
|
||||
f0_mel = 1127 * np.log(1 + f0 / 700)
|
||||
f0_mel[f0_mel > 0] = (f0_mel[f0_mel > 0] - f0_mel_min) * 254 / (f0_mel_max - f0_mel_min) + 1
|
||||
f0_mel[f0_mel > 0] = (f0_mel[f0_mel > 0] - f0_mel_min) * 254 / (
|
||||
f0_mel_max - f0_mel_min
|
||||
) + 1
|
||||
f0_mel[f0_mel <= 1] = 1
|
||||
f0_mel[f0_mel > 255] = 255
|
||||
f0_coarse = np.rint(f0_mel).astype(np.int)
|
||||
return f0_coarse, f0bak # 1-0
|
||||
|
||||
def vc(self, model, net_g, sid, audio0, pitch, pitchf, times, index, big_npy, index_rate): # ,file_index,file_big_npy
|
||||
feats = torch.from_numpy(audio0)
|
||||
if (self.is_half == True):
|
||||
# Volume Extract
|
||||
# volume = self.extractVolume(audio, 512)
|
||||
# volume = np.pad(
|
||||
# volume.astype("float"), (start_frame, n_frames - len(volume) - start_frame)
|
||||
# )
|
||||
|
||||
# return f0_coarse, f0bak, volume # 1-0
|
||||
return f0_coarse, f0bak
|
||||
|
||||
# def extractVolume(self, audio, hopsize):
|
||||
# n_frames = int(len(audio) // hopsize) + 1
|
||||
# audio2 = audio**2
|
||||
# audio2 = np.pad(
|
||||
# audio2,
|
||||
# (int(hopsize // 2), int((hopsize + 1) // 2)),
|
||||
# mode="reflect",
|
||||
# )
|
||||
# volume = np.array(
|
||||
# [
|
||||
# np.mean(audio2[int(n * hopsize) : int((n + 1) * hopsize)]) # noqa:E203
|
||||
# for n in range(n_frames)
|
||||
# ]
|
||||
# )
|
||||
# volume = np.sqrt(volume)
|
||||
# return volume
|
||||
|
||||
def pipeline(
|
||||
self,
|
||||
embedder,
|
||||
model,
|
||||
sid,
|
||||
audio,
|
||||
f0_up_key,
|
||||
f0_method,
|
||||
index,
|
||||
big_npy,
|
||||
index_rate,
|
||||
if_f0,
|
||||
silence_front=0,
|
||||
embChannels=256,
|
||||
):
|
||||
audio_pad = np.pad(audio, (self.t_pad, self.t_pad), mode="reflect")
|
||||
p_len = audio_pad.shape[0] // self.window
|
||||
sid = torch.tensor(sid, device=self.device).unsqueeze(0).long()
|
||||
|
||||
# ピッチ検出
|
||||
pitch, pitchf = None, None
|
||||
if if_f0 == 1:
|
||||
pitch, pitchf = self.get_f0(
|
||||
audio_pad,
|
||||
p_len,
|
||||
f0_up_key,
|
||||
f0_method,
|
||||
silence_front=silence_front,
|
||||
)
|
||||
pitch = pitch[:p_len]
|
||||
pitchf = pitchf[:p_len]
|
||||
pitch = torch.tensor(pitch, device=self.device).unsqueeze(0).long()
|
||||
pitchf = torch.tensor(
|
||||
pitchf, device=self.device, dtype=torch.float
|
||||
).unsqueeze(0)
|
||||
|
||||
# tensor
|
||||
feats = torch.from_numpy(audio_pad)
|
||||
if self.is_half is True:
|
||||
feats = feats.half()
|
||||
else:
|
||||
feats = feats.float()
|
||||
@ -92,86 +143,95 @@ class VC(object):
|
||||
feats = feats.mean(-1)
|
||||
assert feats.dim() == 1, feats.dim()
|
||||
feats = feats.view(1, -1)
|
||||
padding_mask = torch.BoolTensor(feats.shape).to(self.device).fill_(False)
|
||||
|
||||
# embedding
|
||||
padding_mask = torch.BoolTensor(feats.shape).to(self.device).fill_(False)
|
||||
if embChannels == 256:
|
||||
inputs = {
|
||||
"source": feats.to(self.device),
|
||||
"padding_mask": padding_mask,
|
||||
"output_layer": 9, # layer 9
|
||||
}
|
||||
t0 = ttime()
|
||||
with torch.no_grad():
|
||||
logits = model.extract_features(**inputs)
|
||||
feats = model.final_proj(logits[0])
|
||||
else:
|
||||
inputs = {
|
||||
"source": feats.to(self.device),
|
||||
"padding_mask": padding_mask,
|
||||
}
|
||||
|
||||
if (isinstance(index, type(None)) == False and isinstance(big_npy, type(None)) == False and index_rate != 0):
|
||||
with torch.no_grad():
|
||||
logits = embedder.extract_features(**inputs)
|
||||
if embChannels == 256:
|
||||
feats = embedder.final_proj(logits[0])
|
||||
else:
|
||||
feats = logits[0]
|
||||
|
||||
# Index - feature抽出
|
||||
if (
|
||||
isinstance(index, type(None)) is False
|
||||
and isinstance(big_npy, type(None)) is False
|
||||
and index_rate != 0
|
||||
):
|
||||
npy = feats[0].cpu().numpy()
|
||||
if (self.is_half == True):
|
||||
if self.is_half is True:
|
||||
npy = npy.astype("float32")
|
||||
D, I = index.search(npy, 1)
|
||||
npy = big_npy[I.squeeze()]
|
||||
if (self.is_half == True):
|
||||
if self.is_half is True:
|
||||
npy = npy.astype("float16")
|
||||
feats = torch.from_numpy(npy).unsqueeze(0).to(self.device) * index_rate + (1 - index_rate) * feats
|
||||
|
||||
feats = (
|
||||
torch.from_numpy(npy).unsqueeze(0).to(self.device) * index_rate
|
||||
+ (1 - index_rate) * feats
|
||||
)
|
||||
|
||||
#
|
||||
feats = F.interpolate(feats.permute(0, 2, 1), scale_factor=2).permute(0, 2, 1)
|
||||
|
||||
t1 = ttime()
|
||||
p_len = audio0.shape[0] // self.window
|
||||
if (feats.shape[1] < p_len):
|
||||
# ピッチ抽出
|
||||
p_len = audio_pad.shape[0] // self.window
|
||||
if feats.shape[1] < p_len:
|
||||
p_len = feats.shape[1]
|
||||
if (pitch != None and pitchf != None):
|
||||
if pitch is not None and pitchf is not None:
|
||||
pitch = pitch[:, :p_len]
|
||||
pitchf = pitchf[:, :p_len]
|
||||
p_len = torch.tensor([p_len], device=self.device).long()
|
||||
|
||||
# 推論実行
|
||||
with torch.no_grad():
|
||||
audio1 = (net_g.infer(feats, p_len, pitch, pitchf, sid)[0][0, 0] * 32768).data.cpu().float().numpy().astype(np.int16)
|
||||
if pitch is not None:
|
||||
audio1 = (
|
||||
(model.infer(feats, p_len, pitch, pitchf, sid)[0][0, 0] * 32768)
|
||||
.data.cpu()
|
||||
.float()
|
||||
.numpy()
|
||||
.astype(np.int16)
|
||||
)
|
||||
else:
|
||||
if hasattr(model, "infer_pitchless"):
|
||||
audio1 = (
|
||||
(model.infer_pitchless(feats, p_len, sid)[0][0, 0] * 32768)
|
||||
.data.cpu()
|
||||
.float()
|
||||
.numpy()
|
||||
.astype(np.int16)
|
||||
)
|
||||
else:
|
||||
audio1 = (
|
||||
(model.infer(feats, p_len, sid)[0][0, 0] * 32768)
|
||||
.data.cpu()
|
||||
.float()
|
||||
.numpy()
|
||||
.astype(np.int16)
|
||||
)
|
||||
|
||||
del feats, p_len, padding_mask
|
||||
torch.cuda.empty_cache()
|
||||
t2 = ttime()
|
||||
times[0] += (t1 - t0)
|
||||
times[2] += (t2 - t1)
|
||||
return audio1
|
||||
|
||||
def pipeline(self, model, net_g, sid, audio, times, f0_up_key, f0_method, file_index, file_big_npy, index_rate, if_f0, f0_file=None, silence_front=0):
|
||||
if (file_big_npy != "" and file_index != "" and os.path.exists(file_big_npy) == True and os.path.exists(file_index) == True and index_rate != 0):
|
||||
try:
|
||||
index = faiss.read_index(file_index)
|
||||
big_npy = np.load(file_big_npy)
|
||||
except:
|
||||
traceback.print_exc()
|
||||
index = big_npy = None
|
||||
else:
|
||||
index = big_npy = None
|
||||
if self.t_pad_tgt != 0:
|
||||
offset = self.t_pad_tgt
|
||||
end = -1 * self.t_pad_tgt
|
||||
audio1 = audio1[offset:end]
|
||||
|
||||
audio_opt = []
|
||||
t = None
|
||||
t1 = ttime()
|
||||
audio_pad = np.pad(audio, (self.t_pad, self.t_pad), mode='reflect')
|
||||
p_len = audio_pad.shape[0] // self.window
|
||||
inp_f0 = None
|
||||
|
||||
sid = torch.tensor(sid, device=self.device).unsqueeze(0).long()
|
||||
pitch, pitchf = None, None
|
||||
if (if_f0 == 1):
|
||||
pitch, pitchf = self.get_f0(audio_pad, p_len, f0_up_key, f0_method, inp_f0, silence_front=silence_front)
|
||||
pitch = pitch[:p_len]
|
||||
pitchf = pitchf[:p_len]
|
||||
pitch = torch.tensor(pitch, device=self.device).unsqueeze(0).long()
|
||||
pitchf = torch.tensor(pitchf, device=self.device, dtype=torch.float).unsqueeze(0)
|
||||
|
||||
t2 = ttime()
|
||||
times[1] += (t2 - t1)
|
||||
if self.t_pad_tgt == 0:
|
||||
audio_opt.append(self.vc(model, net_g, sid, audio_pad[t:], pitch[:, t // self.window:]if t is not None else pitch, pitchf[:,
|
||||
t // self.window:]if t is not None else pitchf, times, index, big_npy, index_rate))
|
||||
else:
|
||||
audio_opt.append(self.vc(model, net_g, sid, audio_pad[t:], pitch[:, t // self.window:]if t is not None else pitch, pitchf[:,
|
||||
t // self.window:]if t is not None else pitchf, times, index, big_npy, index_rate)[self.t_pad_tgt:-self.t_pad_tgt])
|
||||
|
||||
audio_opt = np.concatenate(audio_opt)
|
||||
del pitch, pitchf, sid
|
||||
torch.cuda.empty_cache()
|
||||
return audio_opt
|
||||
return audio1
|
||||
|
@ -1,137 +1,78 @@
|
||||
import sys
|
||||
import os
|
||||
import argparse
|
||||
from distutils.util import strtobool
|
||||
import json
|
||||
import torch
|
||||
from torch import nn
|
||||
from onnxsim import simplify
|
||||
import onnx
|
||||
|
||||
from infer_pack.models import TextEncoder256, GeneratorNSF, PosteriorEncoder, ResidualCouplingBlock
|
||||
from voice_changer.RVC.onnx.SynthesizerTrnMs256NSFsid_ONNX import (
|
||||
SynthesizerTrnMs256NSFsid_ONNX,
|
||||
)
|
||||
from voice_changer.RVC.onnx.SynthesizerTrnMs256NSFsid_nono_ONNX import (
|
||||
SynthesizerTrnMs256NSFsid_nono_ONNX,
|
||||
)
|
||||
from voice_changer.RVC.onnx.SynthesizerTrnMsNSFsidNono_webui_ONNX import (
|
||||
SynthesizerTrnMsNSFsidNono_webui_ONNX,
|
||||
)
|
||||
from voice_changer.RVC.onnx.SynthesizerTrnMsNSFsid_webui_ONNX import (
|
||||
SynthesizerTrnMsNSFsid_webui_ONNX,
|
||||
)
|
||||
from .const import RVC_MODEL_TYPE_RVC, RVC_MODEL_TYPE_WEBUI
|
||||
|
||||
|
||||
class SynthesizerTrnMs256NSFsid_ONNX(nn.Module):
|
||||
def __init__(
|
||||
self,
|
||||
spec_channels,
|
||||
segment_size,
|
||||
inter_channels,
|
||||
hidden_channels,
|
||||
filter_channels,
|
||||
n_heads,
|
||||
n_layers,
|
||||
kernel_size,
|
||||
p_dropout,
|
||||
resblock,
|
||||
resblock_kernel_sizes,
|
||||
resblock_dilation_sizes,
|
||||
upsample_rates,
|
||||
upsample_initial_channel,
|
||||
upsample_kernel_sizes,
|
||||
spk_embed_dim,
|
||||
gin_channels,
|
||||
sr,
|
||||
**kwargs
|
||||
):
|
||||
|
||||
super().__init__()
|
||||
if (type(sr) == type("strr")):
|
||||
sr = sr2sr[sr]
|
||||
self.spec_channels = spec_channels
|
||||
self.inter_channels = inter_channels
|
||||
self.hidden_channels = hidden_channels
|
||||
self.filter_channels = filter_channels
|
||||
self.n_heads = n_heads
|
||||
self.n_layers = n_layers
|
||||
self.kernel_size = kernel_size
|
||||
self.p_dropout = p_dropout
|
||||
self.resblock = resblock
|
||||
self.resblock_kernel_sizes = resblock_kernel_sizes
|
||||
self.resblock_dilation_sizes = resblock_dilation_sizes
|
||||
self.upsample_rates = upsample_rates
|
||||
self.upsample_initial_channel = upsample_initial_channel
|
||||
self.upsample_kernel_sizes = upsample_kernel_sizes
|
||||
self.segment_size = segment_size
|
||||
self.gin_channels = gin_channels
|
||||
# self.hop_length = hop_length#
|
||||
self.spk_embed_dim = spk_embed_dim
|
||||
self.enc_p = TextEncoder256(
|
||||
inter_channels,
|
||||
hidden_channels,
|
||||
filter_channels,
|
||||
n_heads,
|
||||
n_layers,
|
||||
kernel_size,
|
||||
p_dropout,
|
||||
)
|
||||
self.dec = GeneratorNSF(
|
||||
inter_channels,
|
||||
resblock,
|
||||
resblock_kernel_sizes,
|
||||
resblock_dilation_sizes,
|
||||
upsample_rates,
|
||||
upsample_initial_channel,
|
||||
upsample_kernel_sizes,
|
||||
gin_channels=gin_channels, sr=sr, is_half=kwargs["is_half"]
|
||||
)
|
||||
self.enc_q = PosteriorEncoder(
|
||||
spec_channels,
|
||||
inter_channels,
|
||||
hidden_channels,
|
||||
5,
|
||||
1,
|
||||
16,
|
||||
gin_channels=gin_channels,
|
||||
)
|
||||
self.flow = ResidualCouplingBlock(
|
||||
inter_channels, hidden_channels, 5, 1, 3, gin_channels=gin_channels
|
||||
)
|
||||
self.emb_g = nn.Embedding(self.spk_embed_dim, gin_channels)
|
||||
print("gin_channels:", gin_channels, "self.spk_embed_dim:", self.spk_embed_dim)
|
||||
|
||||
def forward(self, phone, phone_lengths, pitch, nsff0, sid, max_len=None):
|
||||
g = self.emb_g(sid).unsqueeze(-1)
|
||||
m_p, logs_p, x_mask = self.enc_p(phone, pitch, phone_lengths)
|
||||
z_p = (m_p + torch.exp(logs_p) * torch.randn_like(m_p) * 0.66666) * x_mask
|
||||
z = self.flow(z_p, x_mask, g=g, reverse=True)
|
||||
o = self.dec((z * x_mask)[:, :, :max_len], nsff0, g=g)
|
||||
return o, x_mask, (z, z_p, m_p, logs_p)
|
||||
|
||||
|
||||
def export2onnx(input_model, output_model, output_model_simple, is_half):
|
||||
def export2onnx(input_model, output_model, output_model_simple, is_half, metadata):
|
||||
cpt = torch.load(input_model, map_location="cpu")
|
||||
if is_half:
|
||||
dev = torch.device("cuda", index=0)
|
||||
else:
|
||||
dev = torch.device("cpu")
|
||||
|
||||
if metadata["f0"] is True and metadata["modelType"] == RVC_MODEL_TYPE_RVC:
|
||||
net_g_onnx = SynthesizerTrnMs256NSFsid_ONNX(*cpt["config"], is_half=is_half)
|
||||
elif metadata["f0"] is True and metadata["modelType"] == RVC_MODEL_TYPE_WEBUI:
|
||||
net_g_onnx = SynthesizerTrnMsNSFsid_webui_ONNX(**cpt["params"], is_half=is_half)
|
||||
elif metadata["f0"] is False and metadata["modelType"] == RVC_MODEL_TYPE_RVC:
|
||||
net_g_onnx = SynthesizerTrnMs256NSFsid_nono_ONNX(*cpt["config"])
|
||||
elif metadata["f0"] is False and metadata["modelType"] == RVC_MODEL_TYPE_WEBUI:
|
||||
net_g_onnx = SynthesizerTrnMsNSFsidNono_webui_ONNX(**cpt["params"])
|
||||
|
||||
net_g_onnx.eval().to(dev)
|
||||
net_g_onnx.load_state_dict(cpt["weight"], strict=False)
|
||||
if is_half:
|
||||
net_g_onnx = net_g_onnx.half()
|
||||
|
||||
if is_half:
|
||||
feats = torch.HalfTensor(1, 2192, 256).to(dev)
|
||||
feats = torch.HalfTensor(1, 2192, metadata["embChannels"]).to(dev)
|
||||
else:
|
||||
feats = torch.FloatTensor(1, 2192, 256).to(dev)
|
||||
feats = torch.FloatTensor(1, 2192, metadata["embChannels"]).to(dev)
|
||||
p_len = torch.LongTensor([2192]).to(dev)
|
||||
pitch = torch.zeros(1, 2192, dtype=torch.int64).to(dev)
|
||||
|
||||
pitchf = torch.FloatTensor(1, 2192).to(dev)
|
||||
sid = torch.LongTensor([0]).to(dev)
|
||||
|
||||
if metadata["f0"] is True:
|
||||
pitch = torch.zeros(1, 2192, dtype=torch.int64).to(dev)
|
||||
pitchf = torch.FloatTensor(1, 2192).to(dev)
|
||||
input_names = ["feats", "p_len", "pitch", "pitchf", "sid"]
|
||||
output_names = ["audio", ]
|
||||
|
||||
torch.onnx.export(net_g_onnx,
|
||||
(
|
||||
inputs = (
|
||||
feats,
|
||||
p_len,
|
||||
pitch,
|
||||
pitchf,
|
||||
sid,
|
||||
),
|
||||
)
|
||||
|
||||
else:
|
||||
input_names = ["feats", "p_len", "sid"]
|
||||
inputs = (
|
||||
feats,
|
||||
p_len,
|
||||
sid,
|
||||
)
|
||||
|
||||
output_names = [
|
||||
"audio",
|
||||
]
|
||||
|
||||
torch.onnx.export(
|
||||
net_g_onnx,
|
||||
inputs,
|
||||
output_model,
|
||||
dynamic_axes={
|
||||
"feats": [1],
|
||||
@ -142,8 +83,12 @@ def export2onnx(input_model, output_model, output_model_simple, is_half):
|
||||
opset_version=17,
|
||||
verbose=False,
|
||||
input_names=input_names,
|
||||
output_names=output_names)
|
||||
output_names=output_names,
|
||||
)
|
||||
|
||||
model_onnx2 = onnx.load(output_model)
|
||||
model_simp, check = simplify(model_onnx2)
|
||||
meta = model_simp.metadata_props.add()
|
||||
meta.key = "metadata"
|
||||
meta.value = json.dumps(metadata)
|
||||
onnx.save(model_simp, output_model_simple)
|
||||
|
277
server/voice_changer/RVC/models.py
Normal file
277
server/voice_changer/RVC/models.py
Normal file
@ -0,0 +1,277 @@
|
||||
import math
|
||||
import torch
|
||||
from torch import nn
|
||||
|
||||
from infer_pack.models import ( # type:ignore
|
||||
GeneratorNSF,
|
||||
PosteriorEncoder,
|
||||
ResidualCouplingBlock,
|
||||
Generator,
|
||||
)
|
||||
from infer_pack import commons, attentions # type:ignore
|
||||
|
||||
|
||||
class TextEncoder(nn.Module):
|
||||
def __init__(
|
||||
self,
|
||||
out_channels,
|
||||
hidden_channels,
|
||||
filter_channels,
|
||||
emb_channels,
|
||||
n_heads,
|
||||
n_layers,
|
||||
kernel_size,
|
||||
p_dropout,
|
||||
f0=True,
|
||||
):
|
||||
super().__init__()
|
||||
self.out_channels = out_channels
|
||||
self.hidden_channels = hidden_channels
|
||||
self.filter_channels = filter_channels
|
||||
self.emb_channels = emb_channels
|
||||
self.n_heads = n_heads
|
||||
self.n_layers = n_layers
|
||||
self.kernel_size = kernel_size
|
||||
self.p_dropout = p_dropout
|
||||
self.emb_phone = nn.Linear(emb_channels, hidden_channels)
|
||||
self.lrelu = nn.LeakyReLU(0.1, inplace=True)
|
||||
if f0 is True:
|
||||
self.emb_pitch = nn.Embedding(256, hidden_channels) # pitch 256
|
||||
self.encoder = attentions.Encoder(
|
||||
hidden_channels, filter_channels, n_heads, n_layers, kernel_size, p_dropout
|
||||
)
|
||||
self.proj = nn.Conv1d(hidden_channels, out_channels * 2, 1)
|
||||
|
||||
def forward(self, phone, pitch, lengths):
|
||||
if pitch is None:
|
||||
x = self.emb_phone(phone)
|
||||
else:
|
||||
x = self.emb_phone(phone) + self.emb_pitch(pitch)
|
||||
x = x * math.sqrt(self.hidden_channels) # [b, t, h]
|
||||
x = self.lrelu(x)
|
||||
x = torch.transpose(x, 1, -1) # [b, h, t]
|
||||
x_mask = torch.unsqueeze(commons.sequence_mask(lengths, x.size(2)), 1).to(
|
||||
x.dtype
|
||||
)
|
||||
x = self.encoder(x * x_mask, x_mask)
|
||||
stats = self.proj(x) * x_mask
|
||||
|
||||
m, logs = torch.split(stats, self.out_channels, dim=1)
|
||||
return m, logs, x_mask
|
||||
|
||||
|
||||
class SynthesizerTrnMsNSFsid(nn.Module):
|
||||
def __init__(
|
||||
self,
|
||||
spec_channels,
|
||||
segment_size,
|
||||
inter_channels,
|
||||
hidden_channels,
|
||||
filter_channels,
|
||||
n_heads,
|
||||
n_layers,
|
||||
kernel_size,
|
||||
p_dropout,
|
||||
resblock,
|
||||
resblock_kernel_sizes,
|
||||
resblock_dilation_sizes,
|
||||
upsample_rates,
|
||||
upsample_initial_channel,
|
||||
upsample_kernel_sizes,
|
||||
spk_embed_dim,
|
||||
gin_channels,
|
||||
emb_channels,
|
||||
sr,
|
||||
**kwargs
|
||||
):
|
||||
super().__init__()
|
||||
self.spec_channels = spec_channels
|
||||
self.inter_channels = inter_channels
|
||||
self.hidden_channels = hidden_channels
|
||||
self.filter_channels = filter_channels
|
||||
self.n_heads = n_heads
|
||||
self.n_layers = n_layers
|
||||
self.kernel_size = kernel_size
|
||||
self.p_dropout = p_dropout
|
||||
self.resblock = resblock
|
||||
self.resblock_kernel_sizes = resblock_kernel_sizes
|
||||
self.resblock_dilation_sizes = resblock_dilation_sizes
|
||||
self.upsample_rates = upsample_rates
|
||||
self.upsample_initial_channel = upsample_initial_channel
|
||||
self.upsample_kernel_sizes = upsample_kernel_sizes
|
||||
self.segment_size = segment_size
|
||||
self.gin_channels = gin_channels
|
||||
self.emb_channels = emb_channels
|
||||
# self.hop_length = hop_length#
|
||||
self.spk_embed_dim = spk_embed_dim
|
||||
self.enc_p = TextEncoder(
|
||||
inter_channels,
|
||||
hidden_channels,
|
||||
filter_channels,
|
||||
emb_channels,
|
||||
n_heads,
|
||||
n_layers,
|
||||
kernel_size,
|
||||
p_dropout,
|
||||
)
|
||||
self.dec = GeneratorNSF(
|
||||
inter_channels,
|
||||
resblock,
|
||||
resblock_kernel_sizes,
|
||||
resblock_dilation_sizes,
|
||||
upsample_rates,
|
||||
upsample_initial_channel,
|
||||
upsample_kernel_sizes,
|
||||
gin_channels=gin_channels,
|
||||
sr=sr,
|
||||
is_half=kwargs["is_half"],
|
||||
)
|
||||
self.enc_q = PosteriorEncoder(
|
||||
spec_channels,
|
||||
inter_channels,
|
||||
hidden_channels,
|
||||
5,
|
||||
1,
|
||||
16,
|
||||
gin_channels=gin_channels,
|
||||
)
|
||||
self.flow = ResidualCouplingBlock(
|
||||
inter_channels, hidden_channels, 5, 1, 3, gin_channels=gin_channels
|
||||
)
|
||||
self.emb_g = nn.Embedding(self.spk_embed_dim, gin_channels)
|
||||
print("gin_channels:", gin_channels, "self.spk_embed_dim:", self.spk_embed_dim)
|
||||
|
||||
def remove_weight_norm(self):
|
||||
self.dec.remove_weight_norm()
|
||||
self.flow.remove_weight_norm()
|
||||
self.enc_q.remove_weight_norm()
|
||||
|
||||
def forward(
|
||||
self, phone, phone_lengths, pitch, pitchf, y, y_lengths, ds
|
||||
): # 这里ds是id,[bs,1]
|
||||
# print(1,pitch.shape)#[bs,t]
|
||||
g = self.emb_g(ds).unsqueeze(-1) # [b, 256, 1]##1是t,广播的
|
||||
m_p, logs_p, x_mask = self.enc_p(phone, pitch, phone_lengths)
|
||||
z, m_q, logs_q, y_mask = self.enc_q(y, y_lengths, g=g)
|
||||
z_p = self.flow(z, y_mask, g=g)
|
||||
z_slice, ids_slice = commons.rand_slice_segments(
|
||||
z, y_lengths, self.segment_size
|
||||
)
|
||||
# print(-1,pitchf.shape,ids_slice,self.segment_size,self.hop_length,self.segment_size//self.hop_length)
|
||||
pitchf = commons.slice_segments2(pitchf, ids_slice, self.segment_size)
|
||||
# print(-2,pitchf.shape,z_slice.shape)
|
||||
o = self.dec(z_slice, pitchf, g=g)
|
||||
return o, ids_slice, x_mask, y_mask, (z, z_p, m_p, logs_p, m_q, logs_q)
|
||||
|
||||
def infer(self, phone, phone_lengths, pitch, nsff0, sid, max_len=None):
|
||||
g = self.emb_g(sid).unsqueeze(-1)
|
||||
m_p, logs_p, x_mask = self.enc_p(phone, pitch, phone_lengths)
|
||||
z_p = (m_p + torch.exp(logs_p) * torch.randn_like(m_p) * 0.66666) * x_mask
|
||||
z = self.flow(z_p, x_mask, g=g, reverse=True)
|
||||
o = self.dec((z * x_mask)[:, :, :max_len], nsff0, g=g)
|
||||
return o, x_mask, (z, z_p, m_p, logs_p)
|
||||
|
||||
|
||||
class SynthesizerTrnMsNSFsidNono(nn.Module):
|
||||
def __init__(
|
||||
self,
|
||||
spec_channels,
|
||||
segment_size,
|
||||
inter_channels,
|
||||
hidden_channels,
|
||||
filter_channels,
|
||||
n_heads,
|
||||
n_layers,
|
||||
kernel_size,
|
||||
p_dropout,
|
||||
resblock,
|
||||
resblock_kernel_sizes,
|
||||
resblock_dilation_sizes,
|
||||
upsample_rates,
|
||||
upsample_initial_channel,
|
||||
upsample_kernel_sizes,
|
||||
spk_embed_dim,
|
||||
gin_channels,
|
||||
emb_channels,
|
||||
sr=None,
|
||||
**kwargs
|
||||
):
|
||||
super().__init__()
|
||||
self.spec_channels = spec_channels
|
||||
self.inter_channels = inter_channels
|
||||
self.hidden_channels = hidden_channels
|
||||
self.filter_channels = filter_channels
|
||||
self.n_heads = n_heads
|
||||
self.n_layers = n_layers
|
||||
self.kernel_size = kernel_size
|
||||
self.p_dropout = p_dropout
|
||||
self.resblock = resblock
|
||||
self.resblock_kernel_sizes = resblock_kernel_sizes
|
||||
self.resblock_dilation_sizes = resblock_dilation_sizes
|
||||
self.upsample_rates = upsample_rates
|
||||
self.upsample_initial_channel = upsample_initial_channel
|
||||
self.upsample_kernel_sizes = upsample_kernel_sizes
|
||||
self.segment_size = segment_size
|
||||
self.gin_channels = gin_channels
|
||||
self.emb_channels = emb_channels
|
||||
# self.hop_length = hop_length#
|
||||
self.spk_embed_dim = spk_embed_dim
|
||||
self.enc_p = TextEncoder(
|
||||
inter_channels,
|
||||
hidden_channels,
|
||||
filter_channels,
|
||||
emb_channels,
|
||||
n_heads,
|
||||
n_layers,
|
||||
kernel_size,
|
||||
p_dropout,
|
||||
f0=False,
|
||||
)
|
||||
self.dec = Generator(
|
||||
inter_channels,
|
||||
resblock,
|
||||
resblock_kernel_sizes,
|
||||
resblock_dilation_sizes,
|
||||
upsample_rates,
|
||||
upsample_initial_channel,
|
||||
upsample_kernel_sizes,
|
||||
gin_channels=gin_channels,
|
||||
)
|
||||
self.enc_q = PosteriorEncoder(
|
||||
spec_channels,
|
||||
inter_channels,
|
||||
hidden_channels,
|
||||
5,
|
||||
1,
|
||||
16,
|
||||
gin_channels=gin_channels,
|
||||
)
|
||||
self.flow = ResidualCouplingBlock(
|
||||
inter_channels, hidden_channels, 5, 1, 3, gin_channels=gin_channels
|
||||
)
|
||||
self.emb_g = nn.Embedding(self.spk_embed_dim, gin_channels)
|
||||
print("gin_channels:", gin_channels, "self.spk_embed_dim:", self.spk_embed_dim)
|
||||
|
||||
def remove_weight_norm(self):
|
||||
self.dec.remove_weight_norm()
|
||||
self.flow.remove_weight_norm()
|
||||
self.enc_q.remove_weight_norm()
|
||||
|
||||
def forward(self, phone, phone_lengths, y, y_lengths, ds): # 这里ds是id,[bs,1]
|
||||
g = self.emb_g(ds).unsqueeze(-1) # [b, 256, 1]##1是t,广播的
|
||||
m_p, logs_p, x_mask = self.enc_p(phone, None, phone_lengths)
|
||||
z, m_q, logs_q, y_mask = self.enc_q(y, y_lengths, g=g)
|
||||
z_p = self.flow(z, y_mask, g=g)
|
||||
z_slice, ids_slice = commons.rand_slice_segments(
|
||||
z, y_lengths, self.segment_size
|
||||
)
|
||||
o = self.dec(z_slice, g=g)
|
||||
return o, ids_slice, x_mask, y_mask, (z, z_p, m_p, logs_p, m_q, logs_q)
|
||||
|
||||
def infer(self, phone, phone_lengths, sid, max_len=None):
|
||||
g = self.emb_g(sid).unsqueeze(-1)
|
||||
m_p, logs_p, x_mask = self.enc_p(phone, None, phone_lengths)
|
||||
z_p = (m_p + torch.exp(logs_p) * torch.randn_like(m_p) * 0.66666) * x_mask
|
||||
z = self.flow(z_p, x_mask, g=g, reverse=True)
|
||||
o = self.dec((z * x_mask)[:, :, :max_len], g=g)
|
||||
return o, x_mask, (z, z_p, m_p, logs_p)
|
@ -0,0 +1,95 @@
|
||||
from torch import nn
|
||||
from infer_pack.models import ( # type:ignore
|
||||
TextEncoder256,
|
||||
GeneratorNSF,
|
||||
PosteriorEncoder,
|
||||
ResidualCouplingBlock,
|
||||
)
|
||||
import torch
|
||||
|
||||
|
||||
class SynthesizerTrnMs256NSFsid_ONNX(nn.Module):
|
||||
def __init__(
|
||||
self,
|
||||
spec_channels,
|
||||
segment_size,
|
||||
inter_channels,
|
||||
hidden_channels,
|
||||
filter_channels,
|
||||
n_heads,
|
||||
n_layers,
|
||||
kernel_size,
|
||||
p_dropout,
|
||||
resblock,
|
||||
resblock_kernel_sizes,
|
||||
resblock_dilation_sizes,
|
||||
upsample_rates,
|
||||
upsample_initial_channel,
|
||||
upsample_kernel_sizes,
|
||||
spk_embed_dim,
|
||||
gin_channels,
|
||||
sr,
|
||||
**kwargs
|
||||
):
|
||||
super().__init__()
|
||||
self.spec_channels = spec_channels
|
||||
self.inter_channels = inter_channels
|
||||
self.hidden_channels = hidden_channels
|
||||
self.filter_channels = filter_channels
|
||||
self.n_heads = n_heads
|
||||
self.n_layers = n_layers
|
||||
self.kernel_size = kernel_size
|
||||
self.p_dropout = p_dropout
|
||||
self.resblock = resblock
|
||||
self.resblock_kernel_sizes = resblock_kernel_sizes
|
||||
self.resblock_dilation_sizes = resblock_dilation_sizes
|
||||
self.upsample_rates = upsample_rates
|
||||
self.upsample_initial_channel = upsample_initial_channel
|
||||
self.upsample_kernel_sizes = upsample_kernel_sizes
|
||||
self.segment_size = segment_size
|
||||
self.gin_channels = gin_channels
|
||||
# self.hop_length = hop_length#
|
||||
self.spk_embed_dim = spk_embed_dim
|
||||
self.enc_p = TextEncoder256(
|
||||
inter_channels,
|
||||
hidden_channels,
|
||||
filter_channels,
|
||||
n_heads,
|
||||
n_layers,
|
||||
kernel_size,
|
||||
p_dropout,
|
||||
)
|
||||
self.dec = GeneratorNSF(
|
||||
inter_channels,
|
||||
resblock,
|
||||
resblock_kernel_sizes,
|
||||
resblock_dilation_sizes,
|
||||
upsample_rates,
|
||||
upsample_initial_channel,
|
||||
upsample_kernel_sizes,
|
||||
gin_channels=gin_channels,
|
||||
sr=sr,
|
||||
is_half=kwargs["is_half"],
|
||||
)
|
||||
self.enc_q = PosteriorEncoder(
|
||||
spec_channels,
|
||||
inter_channels,
|
||||
hidden_channels,
|
||||
5,
|
||||
1,
|
||||
16,
|
||||
gin_channels=gin_channels,
|
||||
)
|
||||
self.flow = ResidualCouplingBlock(
|
||||
inter_channels, hidden_channels, 5, 1, 3, gin_channels=gin_channels
|
||||
)
|
||||
self.emb_g = nn.Embedding(self.spk_embed_dim, gin_channels)
|
||||
print("gin_channels:", gin_channels, "self.spk_embed_dim:", self.spk_embed_dim)
|
||||
|
||||
def forward(self, phone, phone_lengths, pitch, nsff0, sid, max_len=None):
|
||||
g = self.emb_g(sid).unsqueeze(-1)
|
||||
m_p, logs_p, x_mask = self.enc_p(phone, pitch, phone_lengths)
|
||||
z_p = (m_p + torch.exp(logs_p) * torch.randn_like(m_p) * 0.66666) * x_mask
|
||||
z = self.flow(z_p, x_mask, g=g, reverse=True)
|
||||
o = self.dec((z * x_mask)[:, :, :max_len], nsff0, g=g)
|
||||
return o, x_mask, (z, z_p, m_p, logs_p)
|
@ -0,0 +1,94 @@
|
||||
from torch import nn
|
||||
from infer_pack.models import ( # type:ignore
|
||||
TextEncoder256,
|
||||
PosteriorEncoder,
|
||||
ResidualCouplingBlock,
|
||||
Generator,
|
||||
)
|
||||
import torch
|
||||
|
||||
|
||||
class SynthesizerTrnMs256NSFsid_nono_ONNX(nn.Module):
|
||||
def __init__(
|
||||
self,
|
||||
spec_channels,
|
||||
segment_size,
|
||||
inter_channels,
|
||||
hidden_channels,
|
||||
filter_channels,
|
||||
n_heads,
|
||||
n_layers,
|
||||
kernel_size,
|
||||
p_dropout,
|
||||
resblock,
|
||||
resblock_kernel_sizes,
|
||||
resblock_dilation_sizes,
|
||||
upsample_rates,
|
||||
upsample_initial_channel,
|
||||
upsample_kernel_sizes,
|
||||
spk_embed_dim,
|
||||
gin_channels,
|
||||
sr=None,
|
||||
**kwargs
|
||||
):
|
||||
super().__init__()
|
||||
self.spec_channels = spec_channels
|
||||
self.inter_channels = inter_channels
|
||||
self.hidden_channels = hidden_channels
|
||||
self.filter_channels = filter_channels
|
||||
self.n_heads = n_heads
|
||||
self.n_layers = n_layers
|
||||
self.kernel_size = kernel_size
|
||||
self.p_dropout = p_dropout
|
||||
self.resblock = resblock
|
||||
self.resblock_kernel_sizes = resblock_kernel_sizes
|
||||
self.resblock_dilation_sizes = resblock_dilation_sizes
|
||||
self.upsample_rates = upsample_rates
|
||||
self.upsample_initial_channel = upsample_initial_channel
|
||||
self.upsample_kernel_sizes = upsample_kernel_sizes
|
||||
self.segment_size = segment_size
|
||||
self.gin_channels = gin_channels
|
||||
# self.hop_length = hop_length#
|
||||
self.spk_embed_dim = spk_embed_dim
|
||||
self.enc_p = TextEncoder256(
|
||||
inter_channels,
|
||||
hidden_channels,
|
||||
filter_channels,
|
||||
n_heads,
|
||||
n_layers,
|
||||
kernel_size,
|
||||
p_dropout,
|
||||
f0=False,
|
||||
)
|
||||
self.dec = Generator(
|
||||
inter_channels,
|
||||
resblock,
|
||||
resblock_kernel_sizes,
|
||||
resblock_dilation_sizes,
|
||||
upsample_rates,
|
||||
upsample_initial_channel,
|
||||
upsample_kernel_sizes,
|
||||
gin_channels=gin_channels,
|
||||
)
|
||||
self.enc_q = PosteriorEncoder(
|
||||
spec_channels,
|
||||
inter_channels,
|
||||
hidden_channels,
|
||||
5,
|
||||
1,
|
||||
16,
|
||||
gin_channels=gin_channels,
|
||||
)
|
||||
self.flow = ResidualCouplingBlock(
|
||||
inter_channels, hidden_channels, 5, 1, 3, gin_channels=gin_channels
|
||||
)
|
||||
self.emb_g = nn.Embedding(self.spk_embed_dim, gin_channels)
|
||||
print("gin_channels:", gin_channels, "self.spk_embed_dim:", self.spk_embed_dim)
|
||||
|
||||
def forward(self, phone, phone_lengths, sid, max_len=None):
|
||||
g = self.emb_g(sid).unsqueeze(-1)
|
||||
m_p, logs_p, x_mask = self.enc_p(phone, None, phone_lengths)
|
||||
z_p = (m_p + torch.exp(logs_p) * torch.randn_like(m_p) * 0.66666) * x_mask
|
||||
z = self.flow(z_p, x_mask, g=g, reverse=True)
|
||||
o = self.dec((z * x_mask)[:, :, :max_len], g=g)
|
||||
return o, x_mask, (z, z_p, m_p, logs_p)
|
@ -0,0 +1,97 @@
|
||||
from torch import nn
|
||||
from infer_pack.models import ( # type:ignore
|
||||
PosteriorEncoder,
|
||||
ResidualCouplingBlock,
|
||||
Generator,
|
||||
)
|
||||
from voice_changer.RVC.models import TextEncoder
|
||||
import torch
|
||||
|
||||
|
||||
class SynthesizerTrnMsNSFsidNono_webui_ONNX(nn.Module):
|
||||
def __init__(
|
||||
self,
|
||||
spec_channels,
|
||||
segment_size,
|
||||
inter_channels,
|
||||
hidden_channels,
|
||||
filter_channels,
|
||||
n_heads,
|
||||
n_layers,
|
||||
kernel_size,
|
||||
p_dropout,
|
||||
resblock,
|
||||
resblock_kernel_sizes,
|
||||
resblock_dilation_sizes,
|
||||
upsample_rates,
|
||||
upsample_initial_channel,
|
||||
upsample_kernel_sizes,
|
||||
spk_embed_dim,
|
||||
gin_channels,
|
||||
emb_channels,
|
||||
sr=None,
|
||||
**kwargs
|
||||
):
|
||||
super().__init__()
|
||||
self.spec_channels = spec_channels
|
||||
self.inter_channels = inter_channels
|
||||
self.hidden_channels = hidden_channels
|
||||
self.filter_channels = filter_channels
|
||||
self.n_heads = n_heads
|
||||
self.n_layers = n_layers
|
||||
self.kernel_size = kernel_size
|
||||
self.p_dropout = p_dropout
|
||||
self.resblock = resblock
|
||||
self.resblock_kernel_sizes = resblock_kernel_sizes
|
||||
self.resblock_dilation_sizes = resblock_dilation_sizes
|
||||
self.upsample_rates = upsample_rates
|
||||
self.upsample_initial_channel = upsample_initial_channel
|
||||
self.upsample_kernel_sizes = upsample_kernel_sizes
|
||||
self.segment_size = segment_size
|
||||
self.gin_channels = gin_channels
|
||||
self.emb_channels = emb_channels
|
||||
# self.hop_length = hop_length#
|
||||
self.spk_embed_dim = spk_embed_dim
|
||||
self.enc_p = TextEncoder(
|
||||
inter_channels,
|
||||
hidden_channels,
|
||||
filter_channels,
|
||||
emb_channels,
|
||||
n_heads,
|
||||
n_layers,
|
||||
kernel_size,
|
||||
p_dropout,
|
||||
f0=False,
|
||||
)
|
||||
self.dec = Generator(
|
||||
inter_channels,
|
||||
resblock,
|
||||
resblock_kernel_sizes,
|
||||
resblock_dilation_sizes,
|
||||
upsample_rates,
|
||||
upsample_initial_channel,
|
||||
upsample_kernel_sizes,
|
||||
gin_channels=gin_channels,
|
||||
)
|
||||
self.enc_q = PosteriorEncoder(
|
||||
spec_channels,
|
||||
inter_channels,
|
||||
hidden_channels,
|
||||
5,
|
||||
1,
|
||||
16,
|
||||
gin_channels=gin_channels,
|
||||
)
|
||||
self.flow = ResidualCouplingBlock(
|
||||
inter_channels, hidden_channels, 5, 1, 3, gin_channels=gin_channels
|
||||
)
|
||||
self.emb_g = nn.Embedding(self.spk_embed_dim, gin_channels)
|
||||
print("gin_channels:", gin_channels, "self.spk_embed_dim:", self.spk_embed_dim)
|
||||
|
||||
def forward(self, phone, phone_lengths, sid, max_len=None):
|
||||
g = self.emb_g(sid).unsqueeze(-1)
|
||||
m_p, logs_p, x_mask = self.enc_p(phone, None, phone_lengths)
|
||||
z_p = (m_p + torch.exp(logs_p) * torch.randn_like(m_p) * 0.66666) * x_mask
|
||||
z = self.flow(z_p, x_mask, g=g, reverse=True)
|
||||
o = self.dec((z * x_mask)[:, :, :max_len], g=g)
|
||||
return o, x_mask, (z, z_p, m_p, logs_p)
|
@ -0,0 +1,98 @@
|
||||
from torch import nn
|
||||
from infer_pack.models import ( # type:ignore
|
||||
GeneratorNSF,
|
||||
PosteriorEncoder,
|
||||
ResidualCouplingBlock,
|
||||
)
|
||||
from voice_changer.RVC.models import TextEncoder
|
||||
import torch
|
||||
|
||||
|
||||
class SynthesizerTrnMsNSFsid_webui_ONNX(nn.Module):
|
||||
def __init__(
|
||||
self,
|
||||
spec_channels,
|
||||
segment_size,
|
||||
inter_channels,
|
||||
hidden_channels,
|
||||
filter_channels,
|
||||
n_heads,
|
||||
n_layers,
|
||||
kernel_size,
|
||||
p_dropout,
|
||||
resblock,
|
||||
resblock_kernel_sizes,
|
||||
resblock_dilation_sizes,
|
||||
upsample_rates,
|
||||
upsample_initial_channel,
|
||||
upsample_kernel_sizes,
|
||||
spk_embed_dim,
|
||||
gin_channels,
|
||||
emb_channels,
|
||||
sr,
|
||||
**kwargs
|
||||
):
|
||||
super().__init__()
|
||||
self.spec_channels = spec_channels
|
||||
self.inter_channels = inter_channels
|
||||
self.hidden_channels = hidden_channels
|
||||
self.filter_channels = filter_channels
|
||||
self.n_heads = n_heads
|
||||
self.n_layers = n_layers
|
||||
self.kernel_size = kernel_size
|
||||
self.p_dropout = p_dropout
|
||||
self.resblock = resblock
|
||||
self.resblock_kernel_sizes = resblock_kernel_sizes
|
||||
self.resblock_dilation_sizes = resblock_dilation_sizes
|
||||
self.upsample_rates = upsample_rates
|
||||
self.upsample_initial_channel = upsample_initial_channel
|
||||
self.upsample_kernel_sizes = upsample_kernel_sizes
|
||||
self.segment_size = segment_size
|
||||
self.gin_channels = gin_channels
|
||||
self.emb_channels = emb_channels
|
||||
# self.hop_length = hop_length#
|
||||
self.spk_embed_dim = spk_embed_dim
|
||||
self.enc_p = TextEncoder(
|
||||
inter_channels,
|
||||
hidden_channels,
|
||||
filter_channels,
|
||||
emb_channels,
|
||||
n_heads,
|
||||
n_layers,
|
||||
kernel_size,
|
||||
p_dropout,
|
||||
)
|
||||
self.dec = GeneratorNSF(
|
||||
inter_channels,
|
||||
resblock,
|
||||
resblock_kernel_sizes,
|
||||
resblock_dilation_sizes,
|
||||
upsample_rates,
|
||||
upsample_initial_channel,
|
||||
upsample_kernel_sizes,
|
||||
gin_channels=gin_channels,
|
||||
sr=sr,
|
||||
is_half=kwargs["is_half"],
|
||||
)
|
||||
self.enc_q = PosteriorEncoder(
|
||||
spec_channels,
|
||||
inter_channels,
|
||||
hidden_channels,
|
||||
5,
|
||||
1,
|
||||
16,
|
||||
gin_channels=gin_channels,
|
||||
)
|
||||
self.flow = ResidualCouplingBlock(
|
||||
inter_channels, hidden_channels, 5, 1, 3, gin_channels=gin_channels
|
||||
)
|
||||
self.emb_g = nn.Embedding(self.spk_embed_dim, gin_channels)
|
||||
print("gin_channels:", gin_channels, "self.spk_embed_dim:", self.spk_embed_dim)
|
||||
|
||||
def forward(self, phone, phone_lengths, pitch, nsff0, sid, max_len=None):
|
||||
g = self.emb_g(sid).unsqueeze(-1)
|
||||
m_p, logs_p, x_mask = self.enc_p(phone, pitch, phone_lengths)
|
||||
z_p = (m_p + torch.exp(logs_p) * torch.randn_like(m_p) * 0.66666) * x_mask
|
||||
z = self.flow(z_p, x_mask, g=g, reverse=True)
|
||||
o = self.dec((z * x_mask)[:, :, :max_len], nsff0, g=g)
|
||||
return o, x_mask, (z, z_p, m_p, logs_p)
|
@ -1,6 +1,11 @@
|
||||
import sys
|
||||
import os
|
||||
if sys.platform.startswith('darwin'):
|
||||
|
||||
from voice_changer.utils.LoadModelParams import LoadModelParams
|
||||
from voice_changer.utils.VoiceChangerModel import AudioInOut
|
||||
from voice_changer.utils.VoiceChangerParams import VoiceChangerParams
|
||||
|
||||
if sys.platform.startswith("darwin"):
|
||||
baseDir = [x for x in sys.path if x.endswith("Contents/MacOS")]
|
||||
if len(baseDir) != 1:
|
||||
print("baseDir should be only one ", baseDir)
|
||||
@ -12,17 +17,16 @@ else:
|
||||
|
||||
import io
|
||||
from dataclasses import dataclass, asdict, field
|
||||
from functools import reduce
|
||||
import numpy as np
|
||||
import torch
|
||||
import onnxruntime
|
||||
|
||||
# onnxruntime.set_default_logger_severity(3)
|
||||
from const import HUBERT_ONNX_MODEL_PATH
|
||||
|
||||
import pyworld as pw
|
||||
|
||||
from models import SynthesizerTrn
|
||||
import cluster
|
||||
from models import SynthesizerTrn # type:ignore
|
||||
import cluster # type:ignore
|
||||
import utils
|
||||
from fairseq import checkpoint_utils
|
||||
import librosa
|
||||
@ -30,11 +34,16 @@ import librosa
|
||||
from Exceptions import NoModeLoadedException
|
||||
|
||||
|
||||
providers = ['OpenVINOExecutionProvider', "CUDAExecutionProvider", "DmlExecutionProvider", "CPUExecutionProvider"]
|
||||
providers = [
|
||||
"OpenVINOExecutionProvider",
|
||||
"CUDAExecutionProvider",
|
||||
"DmlExecutionProvider",
|
||||
"CPUExecutionProvider",
|
||||
]
|
||||
|
||||
|
||||
@dataclass
|
||||
class SoVitsSvc40Settings():
|
||||
class SoVitsSvc40Settings:
|
||||
gpu: int = 0
|
||||
dstId: int = 0
|
||||
|
||||
@ -51,9 +60,7 @@ class SoVitsSvc40Settings():
|
||||
onnxModelFile: str = ""
|
||||
configFile: str = ""
|
||||
|
||||
speakers: dict[str, int] = field(
|
||||
default_factory=lambda: {}
|
||||
)
|
||||
speakers: dict[str, int] = field(default_factory=lambda: {})
|
||||
|
||||
# ↓mutableな物だけ列挙
|
||||
intData = ["gpu", "dstId", "tran", "predictF0", "extraConvertSize"]
|
||||
@ -62,7 +69,9 @@ class SoVitsSvc40Settings():
|
||||
|
||||
|
||||
class SoVitsSvc40:
|
||||
def __init__(self, params):
|
||||
audio_buffer: AudioInOut | None = None
|
||||
|
||||
def __init__(self, params: VoiceChangerParams):
|
||||
self.settings = SoVitsSvc40Settings()
|
||||
self.net_g = None
|
||||
self.onnx_session = None
|
||||
@ -74,32 +83,30 @@ class SoVitsSvc40:
|
||||
print("so-vits-svc40 initialization:", params)
|
||||
|
||||
# def loadModel(self, config: str, pyTorch_model_file: str = None, onnx_model_file: str = None, clusterTorchModel: str = None):
|
||||
def loadModel(self, props):
|
||||
self.settings.configFile = props["files"]["configFilename"]
|
||||
def loadModel(self, props: LoadModelParams):
|
||||
self.settings.configFile = props.files.configFilename
|
||||
self.hps = utils.get_hparams_from_file(self.settings.configFile)
|
||||
self.settings.speakers = self.hps.spk
|
||||
|
||||
self.settings.pyTorchModelFile = props["files"]["pyTorchModelFilename"]
|
||||
self.settings.onnxModelFile = props["files"]["onnxModelFilename"]
|
||||
clusterTorchModel = props["files"]["clusterTorchModelFilename"]
|
||||
self.settings.pyTorchModelFile = props.files.pyTorchModelFilename
|
||||
self.settings.onnxModelFile = props.files.onnxModelFilename
|
||||
clusterTorchModel = props.files.clusterTorchModelFilename
|
||||
|
||||
content_vec_path = self.params["content_vec_500"]
|
||||
content_vec_onnx_path = self.params["content_vec_500_onnx"]
|
||||
content_vec_onnx_on = self.params["content_vec_500_onnx_on"]
|
||||
hubert_base_path = self.params["hubert_base"]
|
||||
content_vec_path = self.params.content_vec_500
|
||||
content_vec_onnx_path = self.params.content_vec_500_onnx
|
||||
content_vec_onnx_on = self.params.content_vec_500_onnx_on
|
||||
hubert_base_path = self.params.hubert_base
|
||||
|
||||
# hubert model
|
||||
try:
|
||||
|
||||
if os.path.exists(content_vec_path) == False:
|
||||
if os.path.exists(content_vec_path) is False:
|
||||
content_vec_path = hubert_base_path
|
||||
|
||||
if content_vec_onnx_on == True:
|
||||
if content_vec_onnx_on is True:
|
||||
ort_options = onnxruntime.SessionOptions()
|
||||
ort_options.intra_op_num_threads = 8
|
||||
self.content_vec_onnx = onnxruntime.InferenceSession(
|
||||
content_vec_onnx_path,
|
||||
providers=providers
|
||||
content_vec_onnx_path, providers=providers
|
||||
)
|
||||
else:
|
||||
models, saved_cfg, task = checkpoint_utils.load_model_ensemble_and_task(
|
||||
@ -114,7 +121,7 @@ class SoVitsSvc40:
|
||||
|
||||
# cluster
|
||||
try:
|
||||
if clusterTorchModel != None and os.path.exists(clusterTorchModel):
|
||||
if clusterTorchModel is not None and os.path.exists(clusterTorchModel):
|
||||
self.cluster_model = cluster.get_cluster_model(clusterTorchModel)
|
||||
else:
|
||||
self.cluster_model = None
|
||||
@ -122,22 +129,22 @@ class SoVitsSvc40:
|
||||
print("EXCEPTION during loading cluster model ", e)
|
||||
|
||||
# PyTorchモデル生成
|
||||
if self.settings.pyTorchModelFile != None:
|
||||
self.net_g = SynthesizerTrn(
|
||||
if self.settings.pyTorchModelFile is not None:
|
||||
net_g = SynthesizerTrn(
|
||||
self.hps.data.filter_length // 2 + 1,
|
||||
self.hps.train.segment_size // self.hps.data.hop_length,
|
||||
**self.hps.model
|
||||
**self.hps.model,
|
||||
)
|
||||
self.net_g.eval()
|
||||
net_g.eval()
|
||||
self.net_g = net_g
|
||||
utils.load_checkpoint(self.settings.pyTorchModelFile, self.net_g, None)
|
||||
|
||||
# ONNXモデル生成
|
||||
if self.settings.onnxModelFile != None:
|
||||
if self.settings.onnxModelFile is not None:
|
||||
ort_options = onnxruntime.SessionOptions()
|
||||
ort_options.intra_op_num_threads = 8
|
||||
self.onnx_session = onnxruntime.InferenceSession(
|
||||
self.settings.onnxModelFile,
|
||||
providers=providers
|
||||
self.settings.onnxModelFile, providers=providers
|
||||
)
|
||||
# input_info = self.onnx_session.get_inputs()
|
||||
# for i in input_info:
|
||||
@ -147,30 +154,43 @@ class SoVitsSvc40:
|
||||
# print("output", i)
|
||||
return self.get_info()
|
||||
|
||||
def update_settings(self, key: str, val: any):
|
||||
if key == "onnxExecutionProvider" and self.onnx_session != None:
|
||||
def update_settings(self, key: str, val: int | float | str):
|
||||
if key == "onnxExecutionProvider" and self.onnx_session is not None:
|
||||
if val == "CUDAExecutionProvider":
|
||||
if self.settings.gpu < 0 or self.settings.gpu >= self.gpu_num:
|
||||
self.settings.gpu = 0
|
||||
provider_options = [{'device_id': self.settings.gpu}]
|
||||
self.onnx_session.set_providers(providers=[val], provider_options=provider_options)
|
||||
provider_options = [{"device_id": self.settings.gpu}]
|
||||
self.onnx_session.set_providers(
|
||||
providers=[val], provider_options=provider_options
|
||||
)
|
||||
if hasattr(self, "content_vec_onnx"):
|
||||
self.content_vec_onnx.set_providers(providers=[val], provider_options=provider_options)
|
||||
self.content_vec_onnx.set_providers(
|
||||
providers=[val], provider_options=provider_options
|
||||
)
|
||||
else:
|
||||
self.onnx_session.set_providers(providers=[val])
|
||||
if hasattr(self, "content_vec_onnx"):
|
||||
self.content_vec_onnx.set_providers(providers=[val])
|
||||
elif key == "onnxExecutionProvider" and self.onnx_session == None:
|
||||
elif key == "onnxExecutionProvider" and self.onnx_session is None:
|
||||
print("Onnx is not enabled. Please load model.")
|
||||
return False
|
||||
elif key in self.settings.intData:
|
||||
setattr(self.settings, key, int(val))
|
||||
if key == "gpu" and val >= 0 and val < self.gpu_num and self.onnx_session != None:
|
||||
val = int(val)
|
||||
setattr(self.settings, key, val)
|
||||
if (
|
||||
key == "gpu"
|
||||
and val >= 0
|
||||
and val < self.gpu_num
|
||||
and self.onnx_session is not None
|
||||
):
|
||||
providers = self.onnx_session.get_providers()
|
||||
print("Providers:", providers)
|
||||
if "CUDAExecutionProvider" in providers:
|
||||
provider_options = [{'device_id': self.settings.gpu}]
|
||||
self.onnx_session.set_providers(providers=["CUDAExecutionProvider"], provider_options=provider_options)
|
||||
provider_options = [{"device_id": self.settings.gpu}]
|
||||
self.onnx_session.set_providers(
|
||||
providers=["CUDAExecutionProvider"],
|
||||
provider_options=provider_options,
|
||||
)
|
||||
elif key in self.settings.floatData:
|
||||
setattr(self.settings, key, float(val))
|
||||
elif key in self.settings.strData:
|
||||
@ -183,10 +203,12 @@ class SoVitsSvc40:
|
||||
def get_info(self):
|
||||
data = asdict(self.settings)
|
||||
|
||||
data["onnxExecutionProviders"] = self.onnx_session.get_providers() if self.onnx_session != None else []
|
||||
data["onnxExecutionProviders"] = (
|
||||
self.onnx_session.get_providers() if self.onnx_session is not None else []
|
||||
)
|
||||
files = ["configFile", "pyTorchModelFile", "onnxModelFile"]
|
||||
for f in files:
|
||||
if data[f] != None and os.path.exists(data[f]):
|
||||
if data[f] is not None and os.path.exists(data[f]):
|
||||
data[f] = os.path.basename(data[f])
|
||||
else:
|
||||
data[f] = ""
|
||||
@ -194,22 +216,30 @@ class SoVitsSvc40:
|
||||
return data
|
||||
|
||||
def get_processing_sampling_rate(self):
|
||||
if hasattr(self, "hps") == False:
|
||||
if hasattr(self, "hps") is False:
|
||||
raise NoModeLoadedException("config")
|
||||
return self.hps.data.sampling_rate
|
||||
|
||||
def get_unit_f0(self, audio_buffer, tran):
|
||||
wav_44k = audio_buffer
|
||||
# f0 = utils.compute_f0_parselmouth(wav, sampling_rate=self.target_sample, hop_length=self.hop_size)
|
||||
# f0 = utils.compute_f0_dio(wav_44k, sampling_rate=self.hps.data.sampling_rate, hop_length=self.hps.data.hop_length)
|
||||
|
||||
if self.settings.f0Detector == "dio":
|
||||
f0 = compute_f0_dio(wav_44k, sampling_rate=self.hps.data.sampling_rate, hop_length=self.hps.data.hop_length)
|
||||
f0 = compute_f0_dio(
|
||||
wav_44k,
|
||||
sampling_rate=self.hps.data.sampling_rate,
|
||||
hop_length=self.hps.data.hop_length,
|
||||
)
|
||||
else:
|
||||
f0 = compute_f0_harvest(wav_44k, sampling_rate=self.hps.data.sampling_rate, hop_length=self.hps.data.hop_length)
|
||||
f0 = compute_f0_harvest(
|
||||
wav_44k,
|
||||
sampling_rate=self.hps.data.sampling_rate,
|
||||
hop_length=self.hps.data.hop_length,
|
||||
)
|
||||
|
||||
if wav_44k.shape[0] % self.hps.data.hop_length != 0:
|
||||
print(f" !!! !!! !!! wav size not multiple of hopsize: {wav_44k.shape[0] / self.hps.data.hop_length}")
|
||||
print(
|
||||
f" !!! !!! !!! wav size not multiple of hopsize: {wav_44k.shape[0] / self.hps.data.hop_length}"
|
||||
)
|
||||
|
||||
f0, uv = utils.interpolate_f0(f0)
|
||||
f0 = torch.FloatTensor(f0)
|
||||
@ -218,11 +248,14 @@ class SoVitsSvc40:
|
||||
f0 = f0.unsqueeze(0)
|
||||
uv = uv.unsqueeze(0)
|
||||
|
||||
# wav16k = librosa.resample(audio_buffer, orig_sr=24000, target_sr=16000)
|
||||
wav16k_numpy = librosa.resample(audio_buffer, orig_sr=self.hps.data.sampling_rate, target_sr=16000)
|
||||
wav16k_numpy = librosa.resample(
|
||||
audio_buffer, orig_sr=self.hps.data.sampling_rate, target_sr=16000
|
||||
)
|
||||
wav16k_tensor = torch.from_numpy(wav16k_numpy)
|
||||
|
||||
if (self.settings.gpu < 0 or self.gpu_num == 0) or self.settings.framework == "ONNX":
|
||||
if (
|
||||
self.settings.gpu < 0 or self.gpu_num == 0
|
||||
) or self.settings.framework == "ONNX":
|
||||
dev = torch.device("cpu")
|
||||
else:
|
||||
dev = torch.device("cuda", index=self.settings.gpu)
|
||||
@ -232,53 +265,87 @@ class SoVitsSvc40:
|
||||
["units"],
|
||||
{
|
||||
"audio": wav16k_numpy.reshape(1, -1),
|
||||
})
|
||||
},
|
||||
)
|
||||
c = torch.from_numpy(np.array(c)).squeeze(0).transpose(1, 2)
|
||||
# print("onnx hubert:", self.content_vec_onnx.get_providers())
|
||||
else:
|
||||
if self.hps.model.ssl_dim == 768:
|
||||
self.hubert_model = self.hubert_model.to(dev)
|
||||
wav16k_tensor = wav16k_tensor.to(dev)
|
||||
c = get_hubert_content_layer9(self.hubert_model, wav_16k_tensor=wav16k_tensor)
|
||||
c = get_hubert_content_layer9(
|
||||
self.hubert_model, wav_16k_tensor=wav16k_tensor
|
||||
)
|
||||
else:
|
||||
self.hubert_model = self.hubert_model.to(dev)
|
||||
wav16k_tensor = wav16k_tensor.to(dev)
|
||||
c = utils.get_hubert_content(self.hubert_model, wav_16k_tensor=wav16k_tensor)
|
||||
c = utils.get_hubert_content(
|
||||
self.hubert_model, wav_16k_tensor=wav16k_tensor
|
||||
)
|
||||
|
||||
uv = uv.to(dev)
|
||||
f0 = f0.to(dev)
|
||||
|
||||
c = utils.repeat_expand_2d(c.squeeze(0), f0.shape[1])
|
||||
|
||||
if self.settings.clusterInferRatio != 0 and hasattr(self, "cluster_model") and self.cluster_model != None:
|
||||
speaker = [key for key, value in self.settings.speakers.items() if value == self.settings.dstId]
|
||||
if (
|
||||
self.settings.clusterInferRatio != 0
|
||||
and hasattr(self, "cluster_model")
|
||||
and self.cluster_model is not None
|
||||
):
|
||||
speaker = [
|
||||
key
|
||||
for key, value in self.settings.speakers.items()
|
||||
if value == self.settings.dstId
|
||||
]
|
||||
if len(speaker) != 1:
|
||||
print("not only one speaker found.", speaker)
|
||||
pass
|
||||
# print("not only one speaker found.", speaker)
|
||||
else:
|
||||
cluster_c = cluster.get_cluster_center_result(self.cluster_model, c.cpu().numpy().T, speaker[0]).T
|
||||
cluster_c = cluster.get_cluster_center_result(
|
||||
self.cluster_model, c.cpu().numpy().T, speaker[0]
|
||||
).T
|
||||
cluster_c = torch.FloatTensor(cluster_c).to(dev)
|
||||
c = c.to(dev)
|
||||
c = self.settings.clusterInferRatio * cluster_c + (1 - self.settings.clusterInferRatio) * c
|
||||
c = (
|
||||
self.settings.clusterInferRatio * cluster_c
|
||||
+ (1 - self.settings.clusterInferRatio) * c
|
||||
)
|
||||
|
||||
c = c.unsqueeze(0)
|
||||
return c, f0, uv
|
||||
|
||||
def generate_input(self, newData: any, inputSize: int, crossfadeSize: int, solaSearchFrame: int = 0):
|
||||
def generate_input(
|
||||
self,
|
||||
newData: AudioInOut,
|
||||
inputSize: int,
|
||||
crossfadeSize: int,
|
||||
solaSearchFrame: int = 0,
|
||||
):
|
||||
newData = newData.astype(np.float32) / self.hps.data.max_wav_value
|
||||
|
||||
if hasattr(self, "audio_buffer"):
|
||||
self.audio_buffer = np.concatenate([self.audio_buffer, newData], 0) # 過去のデータに連結
|
||||
if self.audio_buffer is not None:
|
||||
self.audio_buffer = np.concatenate(
|
||||
[self.audio_buffer, newData], 0
|
||||
) # 過去のデータに連結
|
||||
else:
|
||||
self.audio_buffer = newData
|
||||
|
||||
convertSize = inputSize + crossfadeSize + solaSearchFrame + self.settings.extraConvertSize
|
||||
convertSize = (
|
||||
inputSize + crossfadeSize + solaSearchFrame + self.settings.extraConvertSize
|
||||
)
|
||||
|
||||
if convertSize % self.hps.data.hop_length != 0: # モデルの出力のホップサイズで切り捨てが発生するので補う。
|
||||
convertSize = convertSize + (self.hps.data.hop_length - (convertSize % self.hps.data.hop_length))
|
||||
convertSize = convertSize + (
|
||||
self.hps.data.hop_length - (convertSize % self.hps.data.hop_length)
|
||||
)
|
||||
|
||||
self.audio_buffer = self.audio_buffer[-1 * convertSize:] # 変換対象の部分だけ抽出
|
||||
convertOffset = -1 * convertSize
|
||||
self.audio_buffer = self.audio_buffer[convertOffset:] # 変換対象の部分だけ抽出
|
||||
|
||||
crop = self.audio_buffer[-1 * (inputSize + crossfadeSize):-1 * (crossfadeSize)]
|
||||
cropOffset = -1 * (inputSize + crossfadeSize)
|
||||
cropEnd = -1 * (crossfadeSize)
|
||||
crop = self.audio_buffer[cropOffset:cropEnd]
|
||||
|
||||
rms = np.sqrt(np.square(crop).mean(axis=0))
|
||||
vol = max(rms, self.prevVol * 0.0)
|
||||
@ -288,38 +355,46 @@ class SoVitsSvc40:
|
||||
return (c, f0, uv, convertSize, vol)
|
||||
|
||||
def _onnx_inference(self, data):
|
||||
if hasattr(self, "onnx_session") == False or self.onnx_session == None:
|
||||
if hasattr(self, "onnx_session") is False or self.onnx_session is None:
|
||||
print("[Voice Changer] No onnx session.")
|
||||
raise NoModeLoadedException("ONNX")
|
||||
|
||||
convertSize = data[3]
|
||||
vol = data[4]
|
||||
data = (data[0], data[1], data[2],)
|
||||
data = (
|
||||
data[0],
|
||||
data[1],
|
||||
data[2],
|
||||
)
|
||||
|
||||
if vol < self.settings.silentThreshold:
|
||||
return np.zeros(convertSize).astype(np.int16)
|
||||
|
||||
c, f0, uv = [x.numpy() for x in data]
|
||||
sid_target = torch.LongTensor([self.settings.dstId]).unsqueeze(0).numpy()
|
||||
audio1 = self.onnx_session.run(
|
||||
audio1 = (
|
||||
self.onnx_session.run(
|
||||
["audio"],
|
||||
{
|
||||
"c": c.astype(np.float32),
|
||||
"f0": f0.astype(np.float32),
|
||||
"uv": uv.astype(np.float32),
|
||||
"g": sid_target.astype(np.int64),
|
||||
"noise_scale": np.array([self.settings.noiseScale]).astype(np.float32),
|
||||
"noise_scale": np.array([self.settings.noiseScale]).astype(
|
||||
np.float32
|
||||
),
|
||||
# "predict_f0": np.array([self.settings.dstId]).astype(np.int64),
|
||||
|
||||
|
||||
})[0][0, 0] * self.hps.data.max_wav_value
|
||||
},
|
||||
)[0][0, 0]
|
||||
* self.hps.data.max_wav_value
|
||||
)
|
||||
|
||||
audio1 = audio1 * vol
|
||||
result = audio1
|
||||
return result
|
||||
|
||||
def _pyTorch_inference(self, data):
|
||||
if hasattr(self, "net_g") == False or self.net_g == None:
|
||||
if hasattr(self, "net_g") is False or self.net_g is None:
|
||||
print("[Voice Changer] No pyTorch session.")
|
||||
raise NoModeLoadedException("pytorch")
|
||||
|
||||
@ -330,19 +405,29 @@ class SoVitsSvc40:
|
||||
|
||||
convertSize = data[3]
|
||||
vol = data[4]
|
||||
data = (data[0], data[1], data[2],)
|
||||
data = (
|
||||
data[0],
|
||||
data[1],
|
||||
data[2],
|
||||
)
|
||||
|
||||
if vol < self.settings.silentThreshold:
|
||||
return np.zeros(convertSize).astype(np.int16)
|
||||
|
||||
with torch.no_grad():
|
||||
c, f0, uv = [x.to(dev)for x in data]
|
||||
c, f0, uv = [x.to(dev) for x in data]
|
||||
sid_target = torch.LongTensor([self.settings.dstId]).to(dev).unsqueeze(0)
|
||||
self.net_g.to(dev)
|
||||
# audio1 = self.net_g.infer(c, f0=f0, g=sid_target, uv=uv, predict_f0=True, noice_scale=0.1)[0][0, 0].data.float()
|
||||
predict_f0_flag = True if self.settings.predictF0 == 1 else False
|
||||
audio1 = self.net_g.infer(c, f0=f0, g=sid_target, uv=uv, predict_f0=predict_f0_flag,
|
||||
noice_scale=self.settings.noiseScale)
|
||||
audio1 = self.net_g.infer(
|
||||
c,
|
||||
f0=f0,
|
||||
g=sid_target,
|
||||
uv=uv,
|
||||
predict_f0=predict_f0_flag,
|
||||
noice_scale=self.settings.noiseScale,
|
||||
)
|
||||
audio1 = audio1[0][0].data.float()
|
||||
# audio1 = self.net_g.infer(c, f0=f0, g=sid_target, uv=uv, predict_f0=predict_f0_flag,
|
||||
# noice_scale=self.settings.noiceScale)[0][0, 0].data.float()
|
||||
@ -367,7 +452,7 @@ class SoVitsSvc40:
|
||||
del self.net_g
|
||||
del self.onnx_session
|
||||
remove_path = os.path.join("so-vits-svc-40")
|
||||
sys.path = [x for x in sys.path if x.endswith(remove_path) == False]
|
||||
sys.path = [x for x in sys.path if x.endswith(remove_path) is False]
|
||||
|
||||
for key in list(sys.modules):
|
||||
val = sys.modules.get(key)
|
||||
@ -376,14 +461,18 @@ class SoVitsSvc40:
|
||||
if file_path.find("so-vits-svc-40" + os.path.sep) >= 0:
|
||||
print("remove", key, file_path)
|
||||
sys.modules.pop(key)
|
||||
except Exception as e:
|
||||
except Exception: # type:ignore
|
||||
pass
|
||||
|
||||
|
||||
def resize_f0(x, target_len):
|
||||
source = np.array(x)
|
||||
source[source < 0.001] = np.nan
|
||||
target = np.interp(np.arange(0, len(source) * target_len, len(source)) / target_len, np.arange(0, len(source)), source)
|
||||
target = np.interp(
|
||||
np.arange(0, len(source) * target_len, len(source)) / target_len,
|
||||
np.arange(0, len(source)),
|
||||
source,
|
||||
)
|
||||
res = np.nan_to_num(target)
|
||||
return res
|
||||
|
||||
@ -406,7 +495,13 @@ def compute_f0_dio(wav_numpy, p_len=None, sampling_rate=44100, hop_length=512):
|
||||
def compute_f0_harvest(wav_numpy, p_len=None, sampling_rate=44100, hop_length=512):
|
||||
if p_len is None:
|
||||
p_len = wav_numpy.shape[0] // hop_length
|
||||
f0, t = pw.harvest(wav_numpy.astype(np.double), fs=sampling_rate, frame_period=5.5, f0_floor=71.0, f0_ceil=1000.0)
|
||||
f0, t = pw.harvest(
|
||||
wav_numpy.astype(np.double),
|
||||
fs=sampling_rate,
|
||||
frame_period=5.5,
|
||||
f0_floor=71.0,
|
||||
f0_ceil=1000.0,
|
||||
)
|
||||
|
||||
for index, pitch in enumerate(f0):
|
||||
f0[index] = round(pitch, 1)
|
||||
|
@ -1,6 +1,11 @@
|
||||
import sys
|
||||
import os
|
||||
if sys.platform.startswith('darwin'):
|
||||
|
||||
from voice_changer.utils.LoadModelParams import LoadModelParams
|
||||
from voice_changer.utils.VoiceChangerModel import AudioInOut
|
||||
from voice_changer.utils.VoiceChangerParams import VoiceChangerParams
|
||||
|
||||
if sys.platform.startswith("darwin"):
|
||||
baseDir = [x for x in sys.path if x.endswith("Contents/MacOS")]
|
||||
if len(baseDir) != 1:
|
||||
print("baseDir should be only one ", baseDir)
|
||||
@ -12,25 +17,29 @@ else:
|
||||
|
||||
import io
|
||||
from dataclasses import dataclass, asdict, field
|
||||
from functools import reduce
|
||||
import numpy as np
|
||||
import torch
|
||||
import onnxruntime
|
||||
import pyworld as pw
|
||||
|
||||
from models import SynthesizerTrn
|
||||
import cluster
|
||||
from models import SynthesizerTrn # type:ignore
|
||||
import cluster # type:ignore
|
||||
import utils
|
||||
from fairseq import checkpoint_utils
|
||||
import librosa
|
||||
|
||||
from Exceptions import NoModeLoadedException
|
||||
|
||||
providers = ['OpenVINOExecutionProvider', "CUDAExecutionProvider", "DmlExecutionProvider", "CPUExecutionProvider"]
|
||||
providers = [
|
||||
"OpenVINOExecutionProvider",
|
||||
"CUDAExecutionProvider",
|
||||
"DmlExecutionProvider",
|
||||
"CPUExecutionProvider",
|
||||
]
|
||||
|
||||
|
||||
@dataclass
|
||||
class SoVitsSvc40v2Settings():
|
||||
class SoVitsSvc40v2Settings:
|
||||
gpu: int = 0
|
||||
dstId: int = 0
|
||||
|
||||
@ -47,9 +56,7 @@ class SoVitsSvc40v2Settings():
|
||||
onnxModelFile: str = ""
|
||||
configFile: str = ""
|
||||
|
||||
speakers: dict[str, int] = field(
|
||||
default_factory=lambda: {}
|
||||
)
|
||||
speakers: dict[str, int] = field(default_factory=lambda: {})
|
||||
|
||||
# ↓mutableな物だけ列挙
|
||||
intData = ["gpu", "dstId", "tran", "predictF0", "extraConvertSize"]
|
||||
@ -58,7 +65,9 @@ class SoVitsSvc40v2Settings():
|
||||
|
||||
|
||||
class SoVitsSvc40v2:
|
||||
def __init__(self, params):
|
||||
audio_buffer: AudioInOut | None = None
|
||||
|
||||
def __init__(self, params: VoiceChangerParams):
|
||||
self.settings = SoVitsSvc40v2Settings()
|
||||
self.net_g = None
|
||||
self.onnx_session = None
|
||||
@ -69,23 +78,21 @@ class SoVitsSvc40v2:
|
||||
self.params = params
|
||||
print("so-vits-svc 40v2 initialization:", params)
|
||||
|
||||
def loadModel(self, props):
|
||||
self.settings.configFile = props["files"]["configFilename"]
|
||||
def loadModel(self, props: LoadModelParams):
|
||||
self.settings.configFile = props.files.configFilename
|
||||
self.hps = utils.get_hparams_from_file(self.settings.configFile)
|
||||
self.settings.speakers = self.hps.spk
|
||||
|
||||
self.settings.pyTorchModelFile = props["files"]["pyTorchModelFilename"]
|
||||
self.settings.onnxModelFile = props["files"]["onnxModelFilename"]
|
||||
clusterTorchModel = props["files"]["clusterTorchModelFilename"]
|
||||
self.settings.pyTorchModelFile = props.files.pyTorchModelFilename
|
||||
self.settings.onnxModelFile = props.files.onnxModelFilename
|
||||
clusterTorchModel = props.files.clusterTorchModelFilename
|
||||
|
||||
content_vec_path = self.params["content_vec_500"]
|
||||
# content_vec_hubert_onnx_path = self.params["content_vec_500_onnx"]
|
||||
# content_vec_hubert_onnx_on = self.params["content_vec_500_onnx_on"]
|
||||
hubert_base_path = self.params["hubert_base"]
|
||||
content_vec_path = self.params.content_vec_500
|
||||
hubert_base_path = self.params.hubert_base
|
||||
|
||||
# hubert model
|
||||
try:
|
||||
if os.path.exists(content_vec_path) == False:
|
||||
if os.path.exists(content_vec_path) is False:
|
||||
content_vec_path = hubert_base_path
|
||||
|
||||
models, saved_cfg, task = checkpoint_utils.load_model_ensemble_and_task(
|
||||
@ -100,7 +107,7 @@ class SoVitsSvc40v2:
|
||||
|
||||
# cluster
|
||||
try:
|
||||
if clusterTorchModel != None and os.path.exists(clusterTorchModel):
|
||||
if clusterTorchModel is not None and os.path.exists(clusterTorchModel):
|
||||
self.cluster_model = cluster.get_cluster_model(clusterTorchModel)
|
||||
else:
|
||||
self.cluster_model = None
|
||||
@ -108,41 +115,50 @@ class SoVitsSvc40v2:
|
||||
print("EXCEPTION during loading cluster model ", e)
|
||||
|
||||
# PyTorchモデル生成
|
||||
if self.settings.pyTorchModelFile != None:
|
||||
self.net_g = SynthesizerTrn(
|
||||
self.hps
|
||||
)
|
||||
self.net_g.eval()
|
||||
if self.settings.pyTorchModelFile is not None:
|
||||
net_g = SynthesizerTrn(self.hps)
|
||||
net_g.eval()
|
||||
self.net_g = net_g
|
||||
utils.load_checkpoint(self.settings.pyTorchModelFile, self.net_g, None)
|
||||
|
||||
# ONNXモデル生成
|
||||
if self.settings.onnxModelFile != None:
|
||||
if self.settings.onnxModelFile is not None:
|
||||
ort_options = onnxruntime.SessionOptions()
|
||||
ort_options.intra_op_num_threads = 8
|
||||
self.onnx_session = onnxruntime.InferenceSession(
|
||||
self.settings.onnxModelFile,
|
||||
providers=providers
|
||||
self.settings.onnxModelFile, providers=providers
|
||||
)
|
||||
input_info = self.onnx_session.get_inputs()
|
||||
# input_info = self.onnx_session.get_inputs()
|
||||
return self.get_info()
|
||||
|
||||
def update_settings(self, key: str, val: any):
|
||||
if key == "onnxExecutionProvider" and self.onnx_session != None:
|
||||
def update_settings(self, key: str, val: int | float | str):
|
||||
if key == "onnxExecutionProvider" and self.onnx_session is not None:
|
||||
if val == "CUDAExecutionProvider":
|
||||
if self.settings.gpu < 0 or self.settings.gpu >= self.gpu_num:
|
||||
self.settings.gpu = 0
|
||||
provider_options = [{'device_id': self.settings.gpu}]
|
||||
self.onnx_session.set_providers(providers=[val], provider_options=provider_options)
|
||||
provider_options = [{"device_id": self.settings.gpu}]
|
||||
self.onnx_session.set_providers(
|
||||
providers=[val], provider_options=provider_options
|
||||
)
|
||||
else:
|
||||
self.onnx_session.set_providers(providers=[val])
|
||||
elif key in self.settings.intData:
|
||||
setattr(self.settings, key, int(val))
|
||||
if key == "gpu" and val >= 0 and val < self.gpu_num and self.onnx_session != None:
|
||||
val = int(val)
|
||||
setattr(self.settings, key, val)
|
||||
if (
|
||||
key == "gpu"
|
||||
and val >= 0
|
||||
and val < self.gpu_num
|
||||
and self.onnx_session is not None
|
||||
):
|
||||
providers = self.onnx_session.get_providers()
|
||||
print("Providers:", providers)
|
||||
if "CUDAExecutionProvider" in providers:
|
||||
provider_options = [{'device_id': self.settings.gpu}]
|
||||
self.onnx_session.set_providers(providers=["CUDAExecutionProvider"], provider_options=provider_options)
|
||||
provider_options = [{"device_id": self.settings.gpu}]
|
||||
self.onnx_session.set_providers(
|
||||
providers=["CUDAExecutionProvider"],
|
||||
provider_options=provider_options,
|
||||
)
|
||||
elif key in self.settings.floatData:
|
||||
setattr(self.settings, key, float(val))
|
||||
elif key in self.settings.strData:
|
||||
@ -155,10 +171,12 @@ class SoVitsSvc40v2:
|
||||
def get_info(self):
|
||||
data = asdict(self.settings)
|
||||
|
||||
data["onnxExecutionProviders"] = self.onnx_session.get_providers() if self.onnx_session != None else []
|
||||
data["onnxExecutionProviders"] = (
|
||||
self.onnx_session.get_providers() if self.onnx_session is not None else []
|
||||
)
|
||||
files = ["configFile", "pyTorchModelFile", "onnxModelFile"]
|
||||
for f in files:
|
||||
if data[f] != None and os.path.exists(data[f]):
|
||||
if data[f] is not None and os.path.exists(data[f]):
|
||||
data[f] = os.path.basename(data[f])
|
||||
else:
|
||||
data[f] = ""
|
||||
@ -166,7 +184,7 @@ class SoVitsSvc40v2:
|
||||
return data
|
||||
|
||||
def get_processing_sampling_rate(self):
|
||||
if hasattr(self, "hps") == False:
|
||||
if hasattr(self, "hps") is False:
|
||||
raise NoModeLoadedException("config")
|
||||
return self.hps.data.sampling_rate
|
||||
|
||||
@ -175,12 +193,22 @@ class SoVitsSvc40v2:
|
||||
# f0 = utils.compute_f0_parselmouth(wav, sampling_rate=self.target_sample, hop_length=self.hop_size)
|
||||
# f0 = utils.compute_f0_dio(wav_44k, sampling_rate=self.hps.data.sampling_rate, hop_length=self.hps.data.hop_length)
|
||||
if self.settings.f0Detector == "dio":
|
||||
f0 = compute_f0_dio(wav_44k, sampling_rate=self.hps.data.sampling_rate, hop_length=self.hps.data.hop_length)
|
||||
f0 = compute_f0_dio(
|
||||
wav_44k,
|
||||
sampling_rate=self.hps.data.sampling_rate,
|
||||
hop_length=self.hps.data.hop_length,
|
||||
)
|
||||
else:
|
||||
f0 = compute_f0_harvest(wav_44k, sampling_rate=self.hps.data.sampling_rate, hop_length=self.hps.data.hop_length)
|
||||
f0 = compute_f0_harvest(
|
||||
wav_44k,
|
||||
sampling_rate=self.hps.data.sampling_rate,
|
||||
hop_length=self.hps.data.hop_length,
|
||||
)
|
||||
|
||||
if wav_44k.shape[0] % self.hps.data.hop_length != 0:
|
||||
print(f" !!! !!! !!! wav size not multiple of hopsize: {wav_44k.shape[0] / self.hps.data.hop_length}")
|
||||
print(
|
||||
f" !!! !!! !!! wav size not multiple of hopsize: {wav_44k.shape[0] / self.hps.data.hop_length}"
|
||||
)
|
||||
|
||||
f0, uv = utils.interpolate_f0(f0)
|
||||
f0 = torch.FloatTensor(f0)
|
||||
@ -190,10 +218,14 @@ class SoVitsSvc40v2:
|
||||
uv = uv.unsqueeze(0)
|
||||
|
||||
# wav16k = librosa.resample(audio_buffer, orig_sr=24000, target_sr=16000)
|
||||
wav16k = librosa.resample(audio_buffer, orig_sr=self.hps.data.sampling_rate, target_sr=16000)
|
||||
wav16k = librosa.resample(
|
||||
audio_buffer, orig_sr=self.hps.data.sampling_rate, target_sr=16000
|
||||
)
|
||||
wav16k = torch.from_numpy(wav16k)
|
||||
|
||||
if (self.settings.gpu < 0 or self.gpu_num == 0) or self.settings.framework == "ONNX":
|
||||
if (
|
||||
self.settings.gpu < 0 or self.gpu_num == 0
|
||||
) or self.settings.framework == "ONNX":
|
||||
dev = torch.device("cpu")
|
||||
else:
|
||||
dev = torch.device("cuda", index=self.settings.gpu)
|
||||
@ -206,36 +238,64 @@ class SoVitsSvc40v2:
|
||||
c = utils.get_hubert_content(self.hubert_model, wav_16k_tensor=wav16k)
|
||||
c = utils.repeat_expand_2d(c.squeeze(0), f0.shape[1])
|
||||
|
||||
if self.settings.clusterInferRatio != 0 and hasattr(self, "cluster_model") and self.cluster_model != None:
|
||||
speaker = [key for key, value in self.settings.speakers.items() if value == self.settings.dstId]
|
||||
if (
|
||||
self.settings.clusterInferRatio != 0
|
||||
and hasattr(self, "cluster_model")
|
||||
and self.cluster_model is not None
|
||||
):
|
||||
speaker = [
|
||||
key
|
||||
for key, value in self.settings.speakers.items()
|
||||
if value == self.settings.dstId
|
||||
]
|
||||
if len(speaker) != 1:
|
||||
print("not only one speaker found.", speaker)
|
||||
pass
|
||||
# print("not only one speaker found.", speaker)
|
||||
else:
|
||||
cluster_c = cluster.get_cluster_center_result(self.cluster_model, c.cpu().numpy().T, speaker[0]).T
|
||||
cluster_c = cluster.get_cluster_center_result(
|
||||
self.cluster_model, c.cpu().numpy().T, speaker[0]
|
||||
).T
|
||||
# cluster_c = cluster.get_cluster_center_result(self.cluster_model, c.cpu().numpy().T, self.settings.dstId).T
|
||||
cluster_c = torch.FloatTensor(cluster_c).to(dev)
|
||||
# print("cluster DEVICE", cluster_c.device, c.device)
|
||||
c = self.settings.clusterInferRatio * cluster_c + (1 - self.settings.clusterInferRatio) * c
|
||||
c = (
|
||||
self.settings.clusterInferRatio * cluster_c
|
||||
+ (1 - self.settings.clusterInferRatio) * c
|
||||
)
|
||||
|
||||
c = c.unsqueeze(0)
|
||||
return c, f0, uv
|
||||
|
||||
def generate_input(self, newData: any, inputSize: int, crossfadeSize: int, solaSearchFrame: int = 0):
|
||||
def generate_input(
|
||||
self,
|
||||
newData: AudioInOut,
|
||||
inputSize: int,
|
||||
crossfadeSize: int,
|
||||
solaSearchFrame: int = 0,
|
||||
):
|
||||
newData = newData.astype(np.float32) / self.hps.data.max_wav_value
|
||||
|
||||
if hasattr(self, "audio_buffer"):
|
||||
self.audio_buffer = np.concatenate([self.audio_buffer, newData], 0) # 過去のデータに連結
|
||||
if self.audio_buffer is not None:
|
||||
self.audio_buffer = np.concatenate(
|
||||
[self.audio_buffer, newData], 0
|
||||
) # 過去のデータに連結
|
||||
else:
|
||||
self.audio_buffer = newData
|
||||
|
||||
convertSize = inputSize + crossfadeSize + solaSearchFrame + self.settings.extraConvertSize
|
||||
convertSize = (
|
||||
inputSize + crossfadeSize + solaSearchFrame + self.settings.extraConvertSize
|
||||
)
|
||||
|
||||
if convertSize % self.hps.data.hop_length != 0: # モデルの出力のホップサイズで切り捨てが発生するので補う。
|
||||
convertSize = convertSize + (self.hps.data.hop_length - (convertSize % self.hps.data.hop_length))
|
||||
convertSize = convertSize + (
|
||||
self.hps.data.hop_length - (convertSize % self.hps.data.hop_length)
|
||||
)
|
||||
convertOffset = -1 * convertSize
|
||||
self.audio_buffer = self.audio_buffer[convertOffset:] # 変換対象の部分だけ抽出
|
||||
|
||||
self.audio_buffer = self.audio_buffer[-1 * convertSize:] # 変換対象の部分だけ抽出
|
||||
|
||||
crop = self.audio_buffer[-1 * (inputSize + crossfadeSize):-1 * (crossfadeSize)]
|
||||
cropOffset = -1 * (inputSize + crossfadeSize)
|
||||
cropEnd = -1 * (crossfadeSize)
|
||||
crop = self.audio_buffer[cropOffset:cropEnd]
|
||||
|
||||
rms = np.sqrt(np.square(crop).mean(axis=0))
|
||||
vol = max(rms, self.prevVol * 0.0)
|
||||
@ -245,19 +305,24 @@ class SoVitsSvc40v2:
|
||||
return (c, f0, uv, convertSize, vol)
|
||||
|
||||
def _onnx_inference(self, data):
|
||||
if hasattr(self, "onnx_session") == False or self.onnx_session == None:
|
||||
if hasattr(self, "onnx_session") is False or self.onnx_session is None:
|
||||
print("[Voice Changer] No onnx session.")
|
||||
raise NoModeLoadedException("ONNX")
|
||||
|
||||
convertSize = data[3]
|
||||
vol = data[4]
|
||||
data = (data[0], data[1], data[2],)
|
||||
data = (
|
||||
data[0],
|
||||
data[1],
|
||||
data[2],
|
||||
)
|
||||
|
||||
if vol < self.settings.silentThreshold:
|
||||
return np.zeros(convertSize).astype(np.int16)
|
||||
|
||||
c, f0, uv = [x.numpy() for x in data]
|
||||
audio1 = self.onnx_session.run(
|
||||
audio1 = (
|
||||
self.onnx_session.run(
|
||||
["audio"],
|
||||
{
|
||||
"c": c,
|
||||
@ -266,9 +331,10 @@ class SoVitsSvc40v2:
|
||||
"uv": np.array([self.settings.dstId]).astype(np.int64),
|
||||
"predict_f0": np.array([self.settings.dstId]).astype(np.int64),
|
||||
"noice_scale": np.array([self.settings.dstId]).astype(np.int64),
|
||||
|
||||
|
||||
})[0][0, 0] * self.hps.data.max_wav_value
|
||||
},
|
||||
)[0][0, 0]
|
||||
* self.hps.data.max_wav_value
|
||||
)
|
||||
|
||||
audio1 = audio1 * vol
|
||||
|
||||
@ -277,7 +343,7 @@ class SoVitsSvc40v2:
|
||||
return result
|
||||
|
||||
def _pyTorch_inference(self, data):
|
||||
if hasattr(self, "net_g") == False or self.net_g == None:
|
||||
if hasattr(self, "net_g") is False or self.net_g is None:
|
||||
print("[Voice Changer] No pyTorch session.")
|
||||
raise NoModeLoadedException("pytorch")
|
||||
|
||||
@ -288,19 +354,29 @@ class SoVitsSvc40v2:
|
||||
|
||||
convertSize = data[3]
|
||||
vol = data[4]
|
||||
data = (data[0], data[1], data[2],)
|
||||
data = (
|
||||
data[0],
|
||||
data[1],
|
||||
data[2],
|
||||
)
|
||||
|
||||
if vol < self.settings.silentThreshold:
|
||||
return np.zeros(convertSize).astype(np.int16)
|
||||
|
||||
with torch.no_grad():
|
||||
c, f0, uv = [x.to(dev)for x in data]
|
||||
c, f0, uv = [x.to(dev) for x in data]
|
||||
sid_target = torch.LongTensor([self.settings.dstId]).to(dev)
|
||||
self.net_g.to(dev)
|
||||
# audio1 = self.net_g.infer(c, f0=f0, g=sid_target, uv=uv, predict_f0=True, noice_scale=0.1)[0][0, 0].data.float()
|
||||
predict_f0_flag = True if self.settings.predictF0 == 1 else False
|
||||
audio1 = self.net_g.infer(c, f0=f0, g=sid_target, uv=uv, predict_f0=predict_f0_flag,
|
||||
noice_scale=self.settings.noiseScale)[0][0, 0].data.float()
|
||||
audio1 = self.net_g.infer(
|
||||
c,
|
||||
f0=f0,
|
||||
g=sid_target,
|
||||
uv=uv,
|
||||
predict_f0=predict_f0_flag,
|
||||
noice_scale=self.settings.noiseScale,
|
||||
)[0][0, 0].data.float()
|
||||
audio1 = audio1 * self.hps.data.max_wav_value
|
||||
|
||||
audio1 = audio1 * vol
|
||||
@ -322,7 +398,7 @@ class SoVitsSvc40v2:
|
||||
del self.onnx_session
|
||||
|
||||
remove_path = os.path.join("so-vits-svc-40v2")
|
||||
sys.path = [x for x in sys.path if x.endswith(remove_path) == False]
|
||||
sys.path = [x for x in sys.path if x.endswith(remove_path) is False]
|
||||
|
||||
for key in list(sys.modules):
|
||||
val = sys.modules.get(key)
|
||||
@ -331,14 +407,18 @@ class SoVitsSvc40v2:
|
||||
if file_path.find("so-vits-svc-40v2" + os.path.sep) >= 0:
|
||||
print("remove", key, file_path)
|
||||
sys.modules.pop(key)
|
||||
except Exception as e:
|
||||
except: # type:ignore
|
||||
pass
|
||||
|
||||
|
||||
def resize_f0(x, target_len):
|
||||
source = np.array(x)
|
||||
source[source < 0.001] = np.nan
|
||||
target = np.interp(np.arange(0, len(source) * target_len, len(source)) / target_len, np.arange(0, len(source)), source)
|
||||
target = np.interp(
|
||||
np.arange(0, len(source) * target_len, len(source)) / target_len,
|
||||
np.arange(0, len(source)),
|
||||
source,
|
||||
)
|
||||
res = np.nan_to_num(target)
|
||||
return res
|
||||
|
||||
@ -361,7 +441,13 @@ def compute_f0_dio(wav_numpy, p_len=None, sampling_rate=44100, hop_length=512):
|
||||
def compute_f0_harvest(wav_numpy, p_len=None, sampling_rate=44100, hop_length=512):
|
||||
if p_len is None:
|
||||
p_len = wav_numpy.shape[0] // hop_length
|
||||
f0, t = pw.harvest(wav_numpy.astype(np.double), fs=sampling_rate, frame_period=5.5, f0_floor=71.0, f0_ceil=1000.0)
|
||||
f0, t = pw.harvest(
|
||||
wav_numpy.astype(np.double),
|
||||
fs=sampling_rate,
|
||||
frame_period=5.5,
|
||||
f0_floor=71.0,
|
||||
f0_ceil=1000.0,
|
||||
)
|
||||
|
||||
for index, pitch in enumerate(f0):
|
||||
f0[index] = round(pitch, 1)
|
||||
|
@ -1,4 +1,4 @@
|
||||
from typing import Any, Callable, Optional, Protocol, TypeAlias, Union, cast
|
||||
from typing import Any, Union, cast
|
||||
from const import TMP_DIR, ModelType
|
||||
import torch
|
||||
import os
|
||||
@ -9,23 +9,26 @@ import resampy
|
||||
|
||||
|
||||
from voice_changer.IORecorder import IORecorder
|
||||
# from voice_changer.IOAnalyzer import IOAnalyzer
|
||||
from voice_changer.utils.LoadModelParams import LoadModelParams
|
||||
|
||||
from voice_changer.utils.Timer import Timer
|
||||
from voice_changer.utils.VoiceChangerModel import VoiceChangerModel, AudioInOut
|
||||
import time
|
||||
from Exceptions import NoModeLoadedException, ONNXInputArgumentException
|
||||
from voice_changer.utils.VoiceChangerParams import VoiceChangerParams
|
||||
|
||||
providers = ['OpenVINOExecutionProvider', "CUDAExecutionProvider", "DmlExecutionProvider", "CPUExecutionProvider"]
|
||||
providers = [
|
||||
"OpenVINOExecutionProvider",
|
||||
"CUDAExecutionProvider",
|
||||
"DmlExecutionProvider",
|
||||
"CPUExecutionProvider",
|
||||
]
|
||||
|
||||
STREAM_INPUT_FILE = os.path.join(TMP_DIR, "in.wav")
|
||||
STREAM_OUTPUT_FILE = os.path.join(TMP_DIR, "out.wav")
|
||||
STREAM_ANALYZE_FILE_DIO = os.path.join(TMP_DIR, "analyze-dio.png")
|
||||
STREAM_ANALYZE_FILE_HARVEST = os.path.join(TMP_DIR, "analyze-harvest.png")
|
||||
|
||||
|
||||
@dataclass
|
||||
class VoiceChangerSettings():
|
||||
class VoiceChangerSettings:
|
||||
inputSampleRate: int = 48000 # 48000 or 24000
|
||||
|
||||
crossFadeOffsetRate: float = 0.1
|
||||
@ -41,35 +44,40 @@ class VoiceChangerSettings():
|
||||
floatData: list[str] = field(
|
||||
default_factory=lambda: ["crossFadeOffsetRate", "crossFadeEndRate"]
|
||||
)
|
||||
strData: list[str] = field(
|
||||
default_factory=lambda: []
|
||||
)
|
||||
strData: list[str] = field(default_factory=lambda: [])
|
||||
|
||||
|
||||
class VoiceChanger():
|
||||
class VoiceChanger:
|
||||
settings: VoiceChangerSettings
|
||||
voiceChanger: VoiceChangerModel
|
||||
ioRecorder: IORecorder
|
||||
sola_buffer: AudioInOut
|
||||
|
||||
def __init__(self, params):
|
||||
def __init__(self, params: VoiceChangerParams):
|
||||
# 初期化
|
||||
self.settings = VoiceChangerSettings()
|
||||
self.onnx_session = None
|
||||
self.currentCrossFadeOffsetRate = 0
|
||||
self.currentCrossFadeEndRate = 0
|
||||
self.currentCrossFadeOffsetRate = 0.0
|
||||
self.currentCrossFadeEndRate = 0.0
|
||||
self.currentCrossFadeOverlapSize = 0 # setting
|
||||
self.crossfadeSize = 0 # calculated
|
||||
|
||||
self.voiceChanger = None
|
||||
self.modelType = None
|
||||
self.modelType: ModelType | None = None
|
||||
self.params = params
|
||||
self.gpu_num = torch.cuda.device_count()
|
||||
self.prev_audio = np.zeros(4096)
|
||||
self.mps_enabled: bool = getattr(torch.backends, "mps", None) is not None and torch.backends.mps.is_available()
|
||||
self.mps_enabled: bool = (
|
||||
getattr(torch.backends, "mps", None) is not None
|
||||
and torch.backends.mps.is_available()
|
||||
)
|
||||
|
||||
print(f"VoiceChanger Initialized (GPU_NUM:{self.gpu_num}, mps_enabled:{self.mps_enabled})")
|
||||
print(
|
||||
f"VoiceChanger Initialized (GPU_NUM:{self.gpu_num}, mps_enabled:{self.mps_enabled})"
|
||||
)
|
||||
|
||||
def switchModelType(self, modelType: ModelType):
|
||||
if hasattr(self, "voiceChanger") and self.voiceChanger != None:
|
||||
if hasattr(self, "voiceChanger") and self.voiceChanger is not None:
|
||||
# return {"status": "ERROR", "msg": "vc is already selected. currently re-select is not implemented"}
|
||||
del self.voiceChanger
|
||||
self.voiceChanger = None
|
||||
@ -77,58 +85,49 @@ class VoiceChanger():
|
||||
self.modelType = modelType
|
||||
if self.modelType == "MMVCv15":
|
||||
from voice_changer.MMVCv15.MMVCv15 import MMVCv15
|
||||
|
||||
self.voiceChanger = MMVCv15() # type: ignore
|
||||
elif self.modelType == "MMVCv13":
|
||||
from voice_changer.MMVCv13.MMVCv13 import MMVCv13
|
||||
|
||||
self.voiceChanger = MMVCv13()
|
||||
elif self.modelType == "so-vits-svc-40v2":
|
||||
from voice_changer.SoVitsSvc40v2.SoVitsSvc40v2 import SoVitsSvc40v2
|
||||
|
||||
self.voiceChanger = SoVitsSvc40v2(self.params)
|
||||
elif self.modelType == "so-vits-svc-40" or self.modelType == "so-vits-svc-40_c":
|
||||
from voice_changer.SoVitsSvc40.SoVitsSvc40 import SoVitsSvc40
|
||||
|
||||
self.voiceChanger = SoVitsSvc40(self.params)
|
||||
elif self.modelType == "DDSP-SVC":
|
||||
from voice_changer.DDSP_SVC.DDSP_SVC import DDSP_SVC
|
||||
|
||||
self.voiceChanger = DDSP_SVC(self.params)
|
||||
elif self.modelType == "RVC":
|
||||
from voice_changer.RVC.RVC import RVC
|
||||
|
||||
self.voiceChanger = RVC(self.params)
|
||||
else:
|
||||
from voice_changer.MMVCv13.MMVCv13 import MMVCv13
|
||||
|
||||
self.voiceChanger = MMVCv13()
|
||||
|
||||
return {"status": "OK", "msg": "vc is switched."}
|
||||
|
||||
def getModelType(self):
|
||||
if self.modelType != None:
|
||||
if self.modelType is not None:
|
||||
return {"status": "OK", "vc": self.modelType}
|
||||
else:
|
||||
return {"status": "OK", "vc": "none"}
|
||||
|
||||
def loadModel(
|
||||
self,
|
||||
props,
|
||||
):
|
||||
|
||||
def loadModel(self, props: LoadModelParams):
|
||||
try:
|
||||
return self.voiceChanger.loadModel(props)
|
||||
except Exception as e:
|
||||
print(traceback.format_exc())
|
||||
print("[Voice Changer] Model Load Error! Check your model is valid.", e)
|
||||
return {"status": "NG"}
|
||||
|
||||
# try:
|
||||
# if self.modelType == "MMVCv15" or self.modelType == "MMVCv13":
|
||||
# return self.voiceChanger.loadModel(config, pyTorch_model_file, onnx_model_file)
|
||||
# elif self.modelType == "so-vits-svc-40" or self.modelType == "so-vits-svc-40_c" or self.modelType == "so-vits-svc-40v2":
|
||||
# return self.voiceChanger.loadModel(config, pyTorch_model_file, onnx_model_file, clusterTorchModel)
|
||||
# elif self.modelType == "RVC":
|
||||
# return self.voiceChanger.loadModel(slot, config, pyTorch_model_file, onnx_model_file, feature_file, index_file, is_half)
|
||||
# else:
|
||||
# return self.voiceChanger.loadModel(config, pyTorch_model_file, onnx_model_file, clusterTorchModel)
|
||||
# except Exception as e:
|
||||
# print("[Voice Changer] Model Load Error! Check your model is valid.", e)
|
||||
# return {"status": "NG"}
|
||||
|
||||
def get_info(self):
|
||||
data = asdict(self.settings)
|
||||
if hasattr(self, "voiceChanger"):
|
||||
@ -143,7 +142,9 @@ class VoiceChanger():
|
||||
if key == "recordIO" and val == 1:
|
||||
if hasattr(self, "ioRecorder"):
|
||||
self.ioRecorder.close()
|
||||
self.ioRecorder = IORecorder(STREAM_INPUT_FILE, STREAM_OUTPUT_FILE, self.settings.inputSampleRate)
|
||||
self.ioRecorder = IORecorder(
|
||||
STREAM_INPUT_FILE, STREAM_OUTPUT_FILE, self.settings.inputSampleRate
|
||||
)
|
||||
if key == "recordIO" and val == 0:
|
||||
if hasattr(self, "ioRecorder"):
|
||||
self.ioRecorder.close()
|
||||
@ -152,14 +153,6 @@ class VoiceChanger():
|
||||
if hasattr(self, "ioRecorder"):
|
||||
self.ioRecorder.close()
|
||||
|
||||
# if hasattr(self, "ioAnalyzer") == False:
|
||||
# self.ioAnalyzer = IOAnalyzer()
|
||||
|
||||
# try:
|
||||
# self.ioAnalyzer.analyze(STREAM_INPUT_FILE, STREAM_ANALYZE_FILE_DIO, STREAM_ANALYZE_FILE_HARVEST, self.settings.inputSampleRate)
|
||||
|
||||
# except Exception as e:
|
||||
# print("recordIO exception", e)
|
||||
elif key in self.settings.floatData:
|
||||
setattr(self.settings, key, float(val))
|
||||
elif key in self.settings.strData:
|
||||
@ -167,19 +160,19 @@ class VoiceChanger():
|
||||
else:
|
||||
if hasattr(self, "voiceChanger"):
|
||||
ret = self.voiceChanger.update_settings(key, val)
|
||||
if ret == False:
|
||||
if ret is False:
|
||||
print(f"{key} is not mutable variable or unknown variable!")
|
||||
else:
|
||||
print(f"voice changer is not initialized!")
|
||||
print("voice changer is not initialized!")
|
||||
return self.get_info()
|
||||
|
||||
def _generate_strength(self, crossfadeSize: int):
|
||||
|
||||
if self.crossfadeSize != crossfadeSize or \
|
||||
self.currentCrossFadeOffsetRate != self.settings.crossFadeOffsetRate or \
|
||||
self.currentCrossFadeEndRate != self.settings.crossFadeEndRate or \
|
||||
self.currentCrossFadeOverlapSize != self.settings.crossFadeOverlapSize:
|
||||
|
||||
if (
|
||||
self.crossfadeSize != crossfadeSize
|
||||
or self.currentCrossFadeOffsetRate != self.settings.crossFadeOffsetRate
|
||||
or self.currentCrossFadeEndRate != self.settings.crossFadeEndRate
|
||||
or self.currentCrossFadeOverlapSize != self.settings.crossFadeOverlapSize
|
||||
):
|
||||
self.crossfadeSize = crossfadeSize
|
||||
self.currentCrossFadeOffsetRate = self.settings.crossFadeOffsetRate
|
||||
self.currentCrossFadeEndRate = self.settings.crossFadeEndRate
|
||||
@ -193,30 +186,54 @@ class VoiceChanger():
|
||||
np_prev_strength = np.cos(percent * 0.5 * np.pi) ** 2
|
||||
np_cur_strength = np.cos((1 - percent) * 0.5 * np.pi) ** 2
|
||||
|
||||
self.np_prev_strength = np.concatenate([np.ones(cf_offset), np_prev_strength,
|
||||
np.zeros(crossfadeSize - cf_offset - len(np_prev_strength))])
|
||||
self.np_cur_strength = np.concatenate([np.zeros(cf_offset), np_cur_strength, np.ones(crossfadeSize - cf_offset - len(np_cur_strength))])
|
||||
self.np_prev_strength = np.concatenate(
|
||||
[
|
||||
np.ones(cf_offset),
|
||||
np_prev_strength,
|
||||
np.zeros(crossfadeSize - cf_offset - len(np_prev_strength)),
|
||||
]
|
||||
)
|
||||
self.np_cur_strength = np.concatenate(
|
||||
[
|
||||
np.zeros(cf_offset),
|
||||
np_cur_strength,
|
||||
np.ones(crossfadeSize - cf_offset - len(np_cur_strength)),
|
||||
]
|
||||
)
|
||||
|
||||
print(f"Generated Strengths: for prev:{self.np_prev_strength.shape}, for cur:{self.np_cur_strength.shape}")
|
||||
print(
|
||||
f"Generated Strengths: for prev:{self.np_prev_strength.shape}, for cur:{self.np_cur_strength.shape}"
|
||||
)
|
||||
|
||||
# ひとつ前の結果とサイズが変わるため、記録は消去する。
|
||||
if hasattr(self, 'np_prev_audio1') == True:
|
||||
if hasattr(self, "np_prev_audio1") is True:
|
||||
delattr(self, "np_prev_audio1")
|
||||
if hasattr(self, "sola_buffer"):
|
||||
if hasattr(self, "sola_buffer") is True:
|
||||
del self.sola_buffer
|
||||
|
||||
# receivedData: tuple of short
|
||||
def on_request(self, receivedData: AudioInOut) -> tuple[AudioInOut, list[Union[int, float]]]:
|
||||
def on_request(
|
||||
self, receivedData: AudioInOut
|
||||
) -> tuple[AudioInOut, list[Union[int, float]]]:
|
||||
return self.on_request_sola(receivedData)
|
||||
|
||||
def on_request_sola(self, receivedData: AudioInOut) -> tuple[AudioInOut, list[Union[int, float]]]:
|
||||
def on_request_sola(
|
||||
self, receivedData: AudioInOut
|
||||
) -> tuple[AudioInOut, list[Union[int, float]]]:
|
||||
try:
|
||||
processing_sampling_rate = self.voiceChanger.get_processing_sampling_rate()
|
||||
|
||||
# 前処理
|
||||
with Timer("pre-process") as t:
|
||||
if self.settings.inputSampleRate != processing_sampling_rate:
|
||||
newData = cast(AudioInOut, resampy.resample(receivedData, self.settings.inputSampleRate, processing_sampling_rate))
|
||||
newData = cast(
|
||||
AudioInOut,
|
||||
resampy.resample(
|
||||
receivedData,
|
||||
self.settings.inputSampleRate,
|
||||
processing_sampling_rate,
|
||||
),
|
||||
)
|
||||
else:
|
||||
newData = receivedData
|
||||
|
||||
@ -226,7 +243,9 @@ class VoiceChanger():
|
||||
crossfade_frame = min(self.settings.crossFadeOverlapSize, block_frame)
|
||||
self._generate_strength(crossfade_frame)
|
||||
|
||||
data = self.voiceChanger.generate_input(newData, block_frame, crossfade_frame, sola_search_frame)
|
||||
data = self.voiceChanger.generate_input(
|
||||
newData, block_frame, crossfade_frame, sola_search_frame
|
||||
)
|
||||
preprocess_time = t.secs
|
||||
|
||||
# 変換処理
|
||||
@ -234,15 +253,31 @@ class VoiceChanger():
|
||||
# Inference
|
||||
audio = self.voiceChanger.inference(data)
|
||||
|
||||
if hasattr(self, 'sola_buffer') == True:
|
||||
if hasattr(self, "sola_buffer") is True:
|
||||
np.set_printoptions(threshold=10000)
|
||||
audio = audio[-sola_search_frame - crossfade_frame - block_frame:]
|
||||
audio_offset = -1 * (
|
||||
sola_search_frame + crossfade_frame + block_frame
|
||||
)
|
||||
audio = audio[audio_offset:]
|
||||
a = 0
|
||||
audio = audio[a:]
|
||||
# SOLA algorithm from https://github.com/yxlllc/DDSP-SVC, https://github.com/liujing04/Retrieval-based-Voice-Conversion-WebUI
|
||||
cor_nom = np.convolve(audio[: crossfade_frame + sola_search_frame], np.flip(self.sola_buffer), 'valid')
|
||||
cor_den = np.sqrt(np.convolve(audio[: crossfade_frame + sola_search_frame] ** 2, np.ones(crossfade_frame), 'valid') + 1e-3)
|
||||
sola_offset = np.argmax(cor_nom / cor_den)
|
||||
|
||||
output_wav = audio[sola_offset: sola_offset + block_frame].astype(np.float64)
|
||||
cor_nom = np.convolve(
|
||||
audio[: crossfade_frame + sola_search_frame],
|
||||
np.flip(self.sola_buffer),
|
||||
"valid",
|
||||
)
|
||||
cor_den = np.sqrt(
|
||||
np.convolve(
|
||||
audio[: crossfade_frame + sola_search_frame] ** 2,
|
||||
np.ones(crossfade_frame),
|
||||
"valid",
|
||||
)
|
||||
+ 1e-3
|
||||
)
|
||||
sola_offset = int(np.argmax(cor_nom / cor_den))
|
||||
sola_end = sola_offset + block_frame
|
||||
output_wav = audio[sola_offset:sola_end].astype(np.float64)
|
||||
output_wav[:crossfade_frame] *= self.np_cur_strength
|
||||
output_wav[:crossfade_frame] += self.sola_buffer[:]
|
||||
|
||||
@ -251,11 +286,16 @@ class VoiceChanger():
|
||||
print("[Voice Changer] no sola buffer. (You can ignore this.)")
|
||||
result = np.zeros(4096).astype(np.int16)
|
||||
|
||||
if hasattr(self, 'sola_buffer') == True and sola_offset < sola_search_frame:
|
||||
sola_buf_org = audio[- sola_search_frame - crossfade_frame + sola_offset: -sola_search_frame + sola_offset]
|
||||
if (
|
||||
hasattr(self, "sola_buffer") is True
|
||||
and sola_offset < sola_search_frame
|
||||
):
|
||||
offset = -1 * (sola_search_frame + crossfade_frame - sola_offset)
|
||||
end = -1 * (sola_search_frame - sola_offset)
|
||||
sola_buf_org = audio[offset:end]
|
||||
self.sola_buffer = sola_buf_org * self.np_prev_strength
|
||||
else:
|
||||
self.sola_buffer = audio[- crossfade_frame:] * self.np_prev_strength
|
||||
self.sola_buffer = audio[-crossfade_frame:] * self.np_prev_strength
|
||||
# self.sola_buffer = audio[- crossfade_frame:]
|
||||
mainprocess_time = t.secs
|
||||
|
||||
@ -263,12 +303,20 @@ class VoiceChanger():
|
||||
with Timer("post-process") as t:
|
||||
result = result.astype(np.int16)
|
||||
if self.settings.inputSampleRate != processing_sampling_rate:
|
||||
outputData = cast(AudioInOut, resampy.resample(result, processing_sampling_rate, self.settings.inputSampleRate).astype(np.int16))
|
||||
outputData = cast(
|
||||
AudioInOut,
|
||||
resampy.resample(
|
||||
result,
|
||||
processing_sampling_rate,
|
||||
self.settings.inputSampleRate,
|
||||
).astype(np.int16),
|
||||
)
|
||||
else:
|
||||
outputData = result
|
||||
|
||||
print_convert_processing(
|
||||
f" Output data size of {result.shape[0]}/{processing_sampling_rate}hz {outputData.shape[0]}/{self.settings.inputSampleRate}hz")
|
||||
f" Output data size of {result.shape[0]}/{processing_sampling_rate}hz {outputData.shape[0]}/{self.settings.inputSampleRate}hz"
|
||||
)
|
||||
|
||||
if self.settings.recordIO == 1:
|
||||
self.ioRecorder.writeInput(receivedData)
|
||||
@ -281,7 +329,9 @@ class VoiceChanger():
|
||||
# # f" Padded!, Output data size of {result.shape[0]}/{processing_sampling_rate}hz {outputData.shape[0]}/{self.settings.inputSampleRate}hz")
|
||||
postprocess_time = t.secs
|
||||
|
||||
print_convert_processing(f" [fin] Input/Output size:{receivedData.shape[0]},{outputData.shape[0]}")
|
||||
print_convert_processing(
|
||||
f" [fin] Input/Output size:{receivedData.shape[0]},{outputData.shape[0]}"
|
||||
)
|
||||
perf = [preprocess_time, mainprocess_time, postprocess_time]
|
||||
return outputData, perf
|
||||
|
||||
@ -299,14 +349,15 @@ class VoiceChanger():
|
||||
def export2onnx(self):
|
||||
return self.voiceChanger.export2onnx()
|
||||
|
||||
|
||||
##############
|
||||
|
||||
|
||||
PRINT_CONVERT_PROCESSING: bool = False
|
||||
# PRINT_CONVERT_PROCESSING = True
|
||||
|
||||
|
||||
def print_convert_processing(mess: str):
|
||||
if PRINT_CONVERT_PROCESSING == True:
|
||||
if PRINT_CONVERT_PROCESSING is True:
|
||||
print(mess)
|
||||
|
||||
|
||||
@ -318,5 +369,7 @@ def pad_array(arr: AudioInOut, target_length: int):
|
||||
pad_width = target_length - current_length
|
||||
pad_left = pad_width // 2
|
||||
pad_right = pad_width - pad_left
|
||||
padded_arr = np.pad(arr, (pad_left, pad_right), 'constant', constant_values=(0, 0))
|
||||
padded_arr = np.pad(
|
||||
arr, (pad_left, pad_right), "constant", constant_values=(0, 0)
|
||||
)
|
||||
return padded_arr
|
||||
|
@ -1,17 +1,23 @@
|
||||
import numpy as np
|
||||
from voice_changer.VoiceChanger import VoiceChanger
|
||||
from const import ModelType
|
||||
from voice_changer.utils.LoadModelParams import LoadModelParams
|
||||
from voice_changer.utils.VoiceChangerModel import AudioInOut
|
||||
from voice_changer.utils.VoiceChangerParams import VoiceChangerParams
|
||||
|
||||
|
||||
class VoiceChangerManager():
|
||||
class VoiceChangerManager(object):
|
||||
_instance = None
|
||||
voiceChanger: VoiceChanger = None
|
||||
|
||||
@classmethod
|
||||
def get_instance(cls, params):
|
||||
if not hasattr(cls, "_instance"):
|
||||
def get_instance(cls, params: VoiceChangerParams):
|
||||
if cls._instance is None:
|
||||
cls._instance = cls()
|
||||
cls._instance.voiceChanger = VoiceChanger(params)
|
||||
return cls._instance
|
||||
|
||||
def loadModel(self, props):
|
||||
def loadModel(self, props: LoadModelParams):
|
||||
info = self.voiceChanger.loadModel(props)
|
||||
if hasattr(info, "status") and info["status"] == "NG":
|
||||
return info
|
||||
@ -20,23 +26,23 @@ class VoiceChangerManager():
|
||||
return info
|
||||
|
||||
def get_info(self):
|
||||
if hasattr(self, 'voiceChanger'):
|
||||
if hasattr(self, "voiceChanger"):
|
||||
info = self.voiceChanger.get_info()
|
||||
info["status"] = "OK"
|
||||
return info
|
||||
else:
|
||||
return {"status": "ERROR", "msg": "no model loaded"}
|
||||
|
||||
def update_settings(self, key: str, val: any):
|
||||
if hasattr(self, 'voiceChanger'):
|
||||
def update_settings(self, key: str, val: str | int | float):
|
||||
if hasattr(self, "voiceChanger"):
|
||||
info = self.voiceChanger.update_settings(key, val)
|
||||
info["status"] = "OK"
|
||||
return info
|
||||
else:
|
||||
return {"status": "ERROR", "msg": "no model loaded"}
|
||||
|
||||
def changeVoice(self, receivedData: any):
|
||||
if hasattr(self, 'voiceChanger') == True:
|
||||
def changeVoice(self, receivedData: AudioInOut):
|
||||
if hasattr(self, "voiceChanger") is True:
|
||||
return self.voiceChanger.on_request(receivedData)
|
||||
else:
|
||||
print("Voice Change is not loaded. Did you load a correct model?")
|
||||
|
19
server/voice_changer/utils/LoadModelParams.py
Normal file
19
server/voice_changer/utils/LoadModelParams.py
Normal file
@ -0,0 +1,19 @@
|
||||
from dataclasses import dataclass
|
||||
|
||||
|
||||
@dataclass
|
||||
class FilePaths:
|
||||
configFilename: str | None
|
||||
pyTorchModelFilename: str | None
|
||||
onnxModelFilename: str | None
|
||||
clusterTorchModelFilename: str | None
|
||||
featureFilename: str | None
|
||||
indexFilename: str | None
|
||||
|
||||
|
||||
@dataclass
|
||||
class LoadModelParams:
|
||||
slot: int
|
||||
isHalf: bool
|
||||
files: FilePaths
|
||||
params: str
|
@ -1,14 +1,30 @@
|
||||
from typing import Any, Callable, Protocol, TypeAlias
|
||||
from typing import Any, Protocol, TypeAlias
|
||||
import numpy as np
|
||||
|
||||
from voice_changer.utils.LoadModelParams import LoadModelParams
|
||||
|
||||
|
||||
AudioInOut: TypeAlias = np.ndarray[Any, np.dtype[np.int16]]
|
||||
|
||||
|
||||
class VoiceChangerModel(Protocol):
|
||||
loadModel: Callable[..., dict[str, Any]]
|
||||
def get_processing_sampling_rate(self) -> int: ...
|
||||
def get_info(self) -> dict[str, Any]: ...
|
||||
def inference(self, data: tuple[Any, ...]) -> Any: ...
|
||||
def generate_input(self, newData: AudioInOut, inputSize: int, crossfadeSize: int) -> tuple[Any, ...]: ...
|
||||
def update_settings(self, key: str, val: Any) -> bool: ...
|
||||
# loadModel: Callable[..., dict[str, Any]]
|
||||
def loadModel(self, params: LoadModelParams):
|
||||
...
|
||||
|
||||
def get_processing_sampling_rate(self) -> int:
|
||||
...
|
||||
|
||||
def get_info(self) -> dict[str, Any]:
|
||||
...
|
||||
|
||||
def inference(self, data: tuple[Any, ...]) -> Any:
|
||||
...
|
||||
|
||||
def generate_input(
|
||||
self, newData: AudioInOut, inputSize: int, crossfadeSize: int
|
||||
) -> tuple[Any, ...]:
|
||||
...
|
||||
|
||||
def update_settings(self, key: str, val: Any) -> bool:
|
||||
...
|
||||
|
11
server/voice_changer/utils/VoiceChangerParams.py
Normal file
11
server/voice_changer/utils/VoiceChangerParams.py
Normal file
@ -0,0 +1,11 @@
|
||||
from dataclasses import dataclass
|
||||
|
||||
|
||||
@dataclass
|
||||
class VoiceChangerParams():
|
||||
content_vec_500: str
|
||||
content_vec_500_onnx: str
|
||||
content_vec_500_onnx_on: bool
|
||||
hubert_base: str
|
||||
hubert_soft: str
|
||||
nsf_hifigan: str
|
@ -1,7 +1,7 @@
|
||||
#!/bin/bash
|
||||
set -eu
|
||||
|
||||
DOCKER_IMAGE=dannadori/vcclient:20230420_003000
|
||||
DOCKER_IMAGE=dannadori/vcclient:20230428_190513
|
||||
#DOCKER_IMAGE=vcclient
|
||||
|
||||
### DEFAULT VAR ###
|
||||
|
Loading…
Reference in New Issue
Block a user